Generative AI in healthcare: Scope, integration, use cases, challenges and future outlook
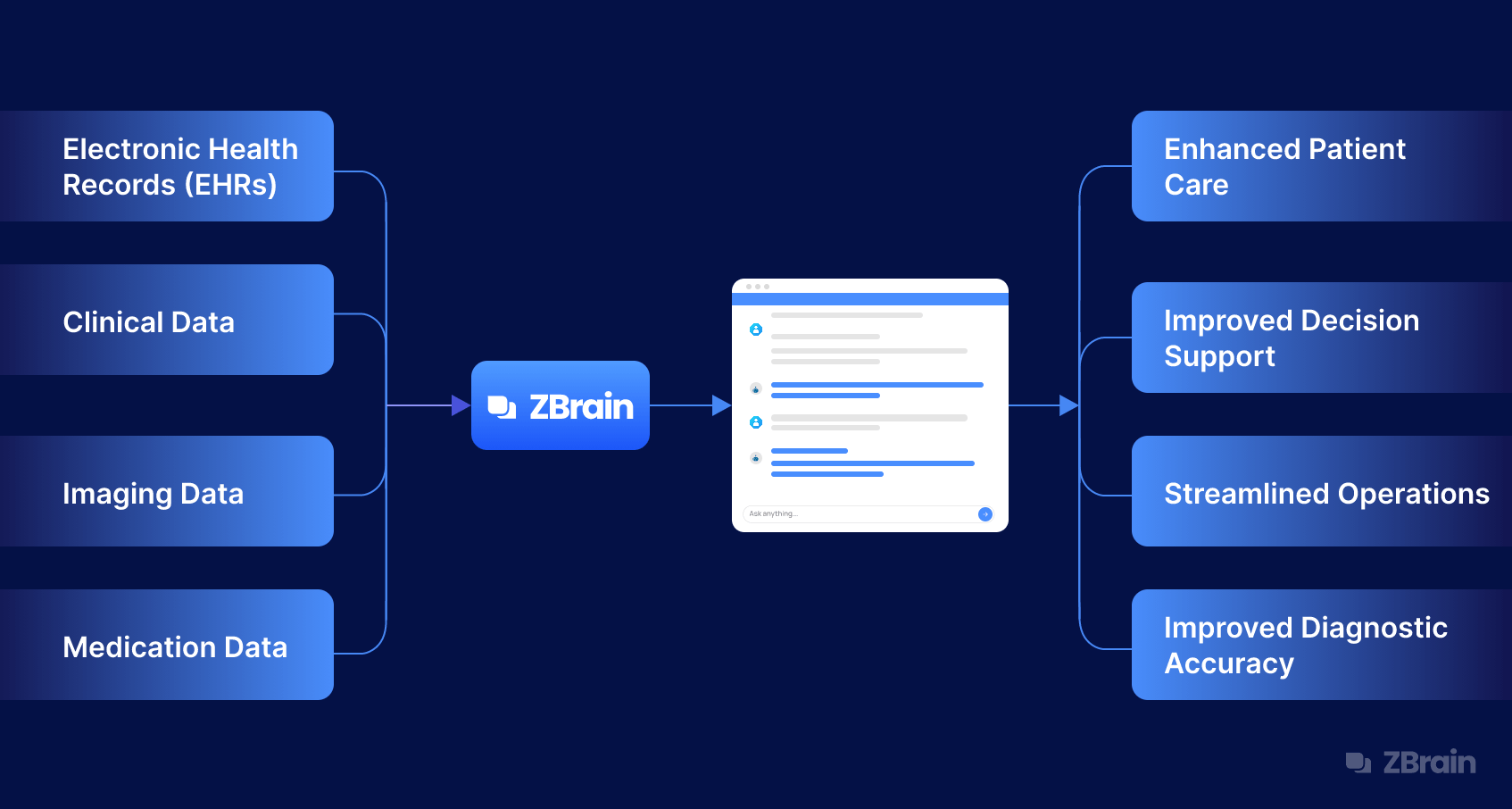
Listen to the article
The healthcare industry is undergoing a technological revolution driven by the rise of generative AI. From enhancing patient care to streamlining complex medical processes, GenAI is rapidly transforming how healthcare professionals diagnose, treat, and engage with patients. The implications are profound: large language models (LLMs), the foundation of GenAI, are poised to create a significant, transformative, and even disruptive impact on the industry.
Consider this: In a recent Gartner Healthcare Provider Research Panel survey from December 2023, a staggering 84% of healthcare executives predicted that GenAI—will either significantly (35%), transformatively (37%), or disruptively (12%) impact the healthcare sector. And healthcare professionals are catching on fast. A recent Wolters Kluwer Health survey shows that 40% of U.S. physicians are already prepared to leverage GenAI at the point of care. Even more telling, 68% of doctors say their views on GenAI have shifted in the past year—they now believe that this technology holds tangible benefits for healthcare.
So, what’s driving this rapid shift? GenAI’s potential to reduce administrative burdens, enhance decision-making, deliver more personalized patient experiences, and streamline workflows is transforming the healthcare landscape, enabling more efficient operations and better patient outcomes. While the potential is immense, the road to successful implementation isn’t without its obstacles. Healthcare organizations must overcome challenges like data privacy concerns, regulatory hurdles, and system integration issues. However, platforms like ZBrain can help streamline this transition by providing a seamless way to integrate generative AI into existing healthcare ecosystems. With features designed to scale, secure, and simplify AI adoption, ZBrain enables healthcare providers to harness the full potential of generative AI effectively.
This article explores the transformative potential of generative AI for healthcare. From assisting clinicians in real-time decision-making to enhancing personalized treatment plans and optimizing administrative workflows, GenAI is setting the stage for a healthcare future that’s more efficient, intelligent, and patient-centered. Join us as we dive into this cutting-edge technology’s most promising use cases and discover how it’s reshaping the healthcare landscape for good.
- What is generative AI?
- Why is generative AI crucial in modern healthcare?
- The current landscape of GenAI in healthcare
- Different approaches to integrating generative AI into healthcare systems
- Generative AI use cases for healthcare
- Why is ZBrain the ideal platform for healthcare organizations?
- Measuring the ROI of generative AI in healthcare
- Challenges and considerations in adopting generative AI for healthcare
- Best practices for implementing generative AI in healthcare operations
- Future outlook of generative AI in healthcare
- Transform healthcare with full-stack generative AI
What is generative AI?
Generative AI is a class of artificial intelligence (AI) that can create original content based on user prompts, such as text, images, video, audio, or software code. It is powered by deep learning models-complex algorithms that simulate human learning and decision-making processes by recognizing patterns and relationships within vast amounts of data. These models “learn” from the vast amounts of data they are trained on, enabling them to generate new and meaningful content in response to natural language requests or other inputs.
The global spotlight on generative AI intensified with the launch of ChatGPT in 2022, a tool capable of generating human-like conversations, driving widespread innovation and adoption. Its ability to boost productivity, streamline workflows, and enhance content creation has made it a critical tool across various industries, including healthcare, manufacturing, and education. According to McKinsey, one-third of organizations already use GenAI in at least one business function, and Gartner predicts that by 2026, over 80% of organizations will have deployed GenAI applications or APIs. Generative AI represents a new era in AI-driven innovation, poised to transform industries by enabling unprecedented levels of creativity and efficiency.
Why is generative AI crucial in modern healthcare?
Generative AI is poised to transform the healthcare industry by significantly enhancing operational efficiency, improving clinical outcomes, and personalizing patient care. Its capabilities allow healthcare providers, payers, and technology companies to optimize processes that were previously time-consuming or prone to human error. Key areas where GenAI can make a profound impact include:
- Clinical productivity: By automating tasks like clinical note-taking, medical document generation, and data extraction from electronic health records (EHRs), healthcare professionals can focus more on patient care, thus improving clinical efficiency.
- Patient engagement and experience: GenAI enhances patient interactions through personalized communication, virtual assistants, and automated follow-up, leading to better patient engagement. It can also aid diagnostic support by analyzing patient data and recommending tailored treatments.
- Streamlining administrative operations: Administrative tasks, including billing, appointment scheduling, and data entry, can be automated using generative AI, reducing the workload on healthcare staff. This increases operational efficiency, minimizes human error, and allows faster decision-making.
- Supporting clinical decision-making: GenAI can be used to analyze clinical notes, electronic health records (EHR), and medical literature, helping healthcare providers make more informed and timely decisions. This offers data-driven insights that support diagnosis, treatment planning, and patient management, improving the overall quality of care.
- Operational efficiency: Hospitals and healthcare organizations use GenAI to streamline operations, from scheduling and resource allocation to optimizing supply chains. This improves cost-effectiveness and reduces bottlenecks in service delivery.
Integrating generative AI into healthcare is crucial for enhancing operational efficiency and delivering more personalized, data-driven care. This integration empowers providers to automate tasks, streamline workflows, and improve decision-making, ultimately transforming patient outcomes and healthcare delivery.
The current landscape of GenAI in healthcare
The adoption of generative AI in healthcare is rapidly growing, supported by both technological advancements and the increasing demand for efficient healthcare solutions. The global market for GenAI in healthcare was valued at approximately $1.6 billion in 2022 and is projected to reach over $30 billion by 2032, growing at a compound annual growth rate (CAGR) of around 35%. North America leads in adoption due to its advanced healthcare infrastructure and the presence of major AI players, while the Asia-Pacific region is expected to see the highest growth, driven by government initiatives and a large patient base.
A recent survey of healthcare leaders indicates that over 70% of healthcare organizations are either in the process of implementing GenAI or actively testing it. Many are starting with proofs of concept, with a focus on clinical and operational applications. Nearly 60% of these organizations are partnering with third-party vendors to co-build customized solutions, while others are developing in-house systems or purchasing off-the-shelf AI tools for specific functions like customer service or diagnostics.
As generative AI continues to gain traction in the healthcare sector, its potential to transform clinical, administrative, and patient care processes is becoming increasingly evident. With growing investments, partnerships, and technological developments, GenAI is set to play a critical role in shaping the future of healthcare, driving both innovation and efficiency across the industry. The ongoing expansion in AI adoption points to a transformative shift in how healthcare services are delivered, ultimately improving patient outcomes and streamlining operations on a global scale.
Optimize Your Operations With AI Agents
Our AI agents streamline your workflows, unlocking new levels of business efficiency!
Different approaches to integrating generative AI into healthcare systems
As healthcare systems increasingly recognize the transformative potential of generative AI, decision-makers have several strategies for integration. Each approach varies in terms of customization, control, and scalability, catering to the diverse needs of healthcare organizations. The primary strategies for integrating generative AI include:
Developing a custom in-house AI stack
This approach involves creating a tailored AI solution specifically designed to meet the unique needs and workflows of the organization. Organizations may choose to build their AI stack from scratch or adapt existing foundation models to align with their operational requirements.
Advantages:
- Customization: By developing a bespoke solution, organizations can ensure that the AI tools fit their specific processes and challenges, enhancing overall operational efficiency.
- Control over security: This method allows for strict oversight of data management and model training, ensuring compliance with healthcare regulations and minimizing data privacy risks.
- Flexibility: Organizations can continuously refine and improve the AI models as they evolve, ensuring that they remain relevant and effective over time.
Using AI point solutions
AI point solutions are standalone applications that address specific tasks and are often built on top of existing large language models (LLMs). These applications may be integrated into existing software to enhance specific operational functions without requiring extensive changes to the underlying systems.
Advantages:
- Task optimization: These solutions excel at solving particular operational challenges, making them effective for targeted needs such as scheduling, patient engagement, or claims processing.
- User-friendly: Generally easier to deploy, these applications require less technical expertise, facilitating broader adoption across the organization.
- Quick deployment: Pre-built solutions can be rapidly configured and operationalized, allowing organizations to realize benefits without lengthy development timelines.
Adopting a fully-integrated platform
Opting for a comprehensive platform like ZBrain equips you with everything necessary, from foundational models for integration to seamless deployment options, all in one convenient location. This approach provides a holistic solution for healthcare organizations looking to leverage GenAI across various functions.
Advantages:
- End-to-end solution: ZBrain offers a comprehensive suite of tools that streamline every aspect of your project, from data preparation to model integration within a single platform, reducing complexity and improving efficiency.
- Scalability: ZBrain is designed to support businesses of all sizes, enabling organizations to scale their GenAI solutions seamlessly as their needs evolve.
- Faster AI implementation: With pre-built tools, advanced orchestration, and seamless third-party integration, ZBrain Builder accelerates the AI implementation process, enabling faster deployment of AI agents.
- Customizability: Organizations can customize ZBrain agents to align with their specific business processes and objectives, driving greater operational efficiency.
- Security and compliance: ZBrain is built with robust security features and meets enterprise-grade compliance standards, protecting sensitive data throughout the AI development lifecycle.
- Data integration and management: Simplifying the integration of proprietary and external data sources, ZBrain ensures the development of accurate, data-driven AI solutions.
- Reduced costs: By providing all necessary tools in one platform, ZBrain reduces the need for multiple specialized resources, streamlining the process and lowering overall AI development costs.
Choosing the right approach to integrating generative AI into healthcare systems hinges on several factors, including specific operational needs, available resources, and strategic objectives. Each option presents varying levels of control, customization, and complexity, making it crucial for healthcare leaders to carefully evaluate how to implement GenAI in a manner that aligns with their business goals and meets compliance requirements. With thoughtful planning and execution, generative AI can significantly enhance efficiency, improve patient care, and drive innovation across the healthcare landscape.
Generative AI use cases for healthcare
Generative AI is not just a buzzword in healthcare; it is a powerful tool with the potential to transform the industry. Its ability to process vast amounts of data, generate insightful content, and personalize experiences creates strategic opportunities across various segments. Here is a closer look at key use cases for strategic implementation:
Healthcare providers:
- Personalized care plans & treatment recommendations: Analyzes a patient’s medical history, genetic data, and lifestyle factors to develop highly personalized care plans and treatment recommendations.
- Enhanced diagnostic support: Analyzes medical images (radiology, pathology) and patient data to identify potential abnormalities and suggest diagnoses, aiding physicians in making quicker and more accurate decisions.
- Efficient documentation & reporting: Automates the generation of clinical notes, discharge summaries, and other reports, freeing up physicians’ time for patient care and reducing the administrative burden.
- Personalized patient education: Creates tailored health information, videos, and infographics based on patient conditions, cultural background, and learning style.
Pharmaceutical firms:
- Accelerated drug discovery & development: Analyzes vast datasets of chemical and biological information to identify promising drug candidates and optimize their properties, significantly shortening the time and cost of drug development.
- Personalized medicine & precision therapies: Predicts a patient’s response to specific treatments based on their genetic profile and other factors, leading to more effective therapies and reduced side effects.
- Clinical trial optimization: Creates synthetic patient populations, optimizes trial designs, and predicts trial outcomes, leading to faster and more efficient development of new therapies.
Payers:
- Risk stratification & predictive analytics: Identifies high-risk populations and predict potential healthcare costs based on a member’s medical history, lifestyle, and other factors, enabling payers to implement targeted interventions and manage costs more effectively.
- Personalized member engagement: Generates personalized health recommendations, educational materials, and communication strategies based on each member’s needs and preferences, improving engagement and promoting healthy behaviors.
- Automated claims processing & fraud detection: Analyzes large datasets of claims data to identify patterns, detect fraud, and automate claims processing, leading to cost savings and improved efficiency.
- Personalized benefits guidance: Gen AI chatbots answer member questions about benefits, coverage, and procedures, improving member satisfaction and reducing administrative burden.
Medtech:
- Remote patient monitoring & telehealth: Analyzes data from remote patient monitoring devices to provide real-time insights to healthcare providers and enable more efficient and personalized telehealth services.
Services & operations:
- Data-driven insights & analytics: Extracts valuable insights from healthcare data, including EHRs, claims data, and research papers, to identify trends, improve decision-making, and optimize resource allocation.
- Workflow optimization & automation: Automates repetitive tasks in healthcare operations, such as scheduling, appointment reminders, and billing, freeing up staff for more complex and value-added activities.
Public-health agencies:
- Disease surveillance & outbreak prediction: Analyzes data from multiple sources to track disease outbreaks, predict future trends, and develop effective public health interventions.
- Resource allocation & prioritization: Examines population health data and disease trends to inform resource allocation, ensuring optimal use of public health funding and interventions.
- Public health education & communication: Generates personalized health education materials and communication campaigns tailored to specific populations and health issues, promoting public awareness and preventative behaviors.
Strategic implementation of generative AI has the potential to transform healthcare by improving patient outcomes, enhancing efficiency, and driving innovation. By embracing this technology responsibly and ethically, we can unlock its full potential to create a more efficient, personalized, and equitable healthcare system.
Why is ZBrain the ideal platform for healthcare organizations?
In an industry where privacy, accuracy, and efficiency are paramount, ZBrain stands out as the optimal platform for healthcare organizations looking to integrate GenAI into their operations. Here are the key reasons why ZBrain is the perfect fit for healthcare:
- Proprietary data utilization and privacy: ZBrain can empower healthcare organizations to leverage their proprietary data, including clinical records, patient histories, and EHR data while maintaining full control over data privacy and security. It is designed for private enterprise deployments, ensuring that sensitive healthcare information remains secure and compliant with regulations like HIPAA. ZBrain leverages private data to develop generative AI solutions that are highly tailored, contextually relevant, and optimized to enhance patient care while streamlining healthcare operations.
- Advanced knowledge base for efficient data retrieval: ZBrain excels in handling diverse and complex datasets, including structured, semi-structured, and unstructured medical data. It integrates information from electronic health records (EHRs), research databases, and clinical notes to create a robust knowledge base. This ensures efficient retrieval of relevant medical information, helping healthcare professionals make informed decisions quickly and accurately. Whether for diagnostic support, treatment recommendations, or drug interactions, ZBrain’s knowledge base can present the right information at the right time.
- Low-code platform: ZBrain Builder’s intuitive low-code interface allows users to create business logic for complex genAI solutions with minimal coding effort. Healthcare organizations can leverage this platform to develop solutions for automating intricate and multi-layered tasks, saving time and reducing dependence on specialized technical teams. With pre-built components, users can efficiently design and implement solutions tailored to specific healthcare needs.
- AI agents for automation: ZBrain enables building intelligent agents that can autonomously execute and manage workflows, enabling healthcare organizations to automate routine tasks. From automating appointment scheduling and handling patient inquiries to assisting with medical coding and billing, ZBrain’s AI agents free up healthcare staff to focus on more complex, high-value tasks. This task automation boosts operational efficiency and reduces administrative burden, leading to improved patient experience and staff productivity.
- Customizable AI agents: ZBrain Builder provides extensive flexibility, allowing users to tailor generative AI agents to their unique operational needs. Whether automating clinical report generation or handling NLP tasks like sentiment analysis of patient feedback, its adaptable architecture supports a wide range of use cases. By allowing users to create custom AI agents, ZBrain ensures these solutions are customized to specific clinical and administrative objectives, driving improvements in care delivery and patient outcomes.
- Human-in-the-loop for accuracy: GenAI in healthcare requires human oversight to ensure accuracy, especially when dealing with critical decisions like diagnoses and treatment recommendations. ZBrain’s “human-in-the-loop” feature allows clinicians and staff to provide feedback on AI-generated results, refining the model’s outputs over time. This continuous feedback loop ensures healthcare GenAI solutions or AI agents maintain accuracy and alignment with clinical standards, reducing errors and enhancing care quality.
- Cloud and model agnostic: ZBrain’s cloud- and model-agnostic design offers flexibility, allowing healthcare organizations to deploy GenAI solutions on their preferred cloud infrastructure—whether it’s AWS, Google Cloud, Azure, or a private cloud. This flexibility also extends to AI model integration, enabling the use of both proprietary models and open-source models based on the organization’s requirements. This modular architecture ensures that healthcare providers can always work with the best-fit models and cloud environments for their specific needs.
- Seamless integration with existing healthcare systems: Healthcare organizations often rely on a wide range of enterprise software systems, from EHRs to CRM platforms and data clouds. ZBrain agents integrate seamlessly with existing healthcare systems, ensuring smooth interoperability and enhancing overall efficiency. This allows healthcare providers to enhance their current infrastructure with AI capabilities without disrupting ongoing operations.
- Scalability and continuous improvement: As healthcare organizations grow and face evolving challenges, ZBrain offers the scalability to accommodate more complex and data-intensive GenAI solutions. Continuous monitoring helps ensure genAI solutions operate effectively by proactively identifying and addressing potential issues. With ZBrain, healthcare organizations can easily update and expand their knowledge base, keeping genAI solutions aligned with the latest information and operational needs.
- It features two core components: ZBrain XPLR, which helps businesses assess AI readiness, identify high-impact opportunities, and strategically align initiatives for maximum value. ZBrain Builder, a low-code interface, lets businesses leverage advanced LLMs like GPT-4, Claude, Llama-3, and Gemini to create tailored, secure AI agents and GenAI solutions.
These features collectively position ZBrain as a powerful ally for healthcare organizations seeking to integrate generative AI effectively into their operations.
Measuring the ROI of generative AI in healthcare
In healthcare organizations, measuring the return on investment (ROI) for generative AI involves assessing both direct financial benefits and indirect enhancements in operational efficiency and patient care quality. ROI is calculated by comparing the cost savings and improved productivity resulting from GenAI implementations against the initial investment in the technology. Reporting on ROI typically incorporates a mix of quantitative metrics, such as reductions in administrative costs and improved throughput, along with qualitative feedback on enhancements in patient satisfaction and healthcare outcomes. This comprehensive approach enables healthcare providers to validate the effectiveness of their GenAI investments and identify areas for further improvement. Let’s examine some specific examples from different use-case categories in healthcare:
Key ROI indicators from ZBrain implementation in healthcare organizations
- Enhanced patient engagement:
- Use case: Personalized patient communications
- ROI metrics: Time savings in content generation and increased patient satisfaction scores.
- Example: A ZBrain chatbot can be used for tailored communication with patients, improving engagement and experience.
- Streamlined operational efficiency:
- Use case: Claims processing automation
- ROI metrics: Reduction in processing times and administrative costs.
- Example: By automating claims reviews, ZBrain can decrease turnaround time, optimize workflows, and minimize overhead costs.
- Improved clinical decision support:
- Use case: GenAI-assisted diagnostics
- ROI metrics: Increased accuracy of diagnoses and faster decision-making.
- Example: ZBrain’s advanced analysis capabilities can enable rapid assessment of patient data, providing clinicians with timely and accurate insights to enhance patient care.
- Enhanced patient support services:
- Use case: Virtual health assistants
- ROI metrics: Reduction in call center volume and increased patient satisfaction.
- Example: By deploying AI-powered chatbots through ZBrain, healthcare organizations can handle patient inquiries more efficiently, reducing wait times and improving patient experience.
- Data-driven insights:
- Use case: Predictive analytics for patient outcomes
- ROI metrics: Improved patient outcomes and reduced readmission rates.
- Example: ZBrain can enable healthcare providers to analyze patient data effectively, supporting proactive interventions that may enhance care and help reduce costs associated with readmissions.
These examples highlight the benefits of generative AI in reducing costs, enhancing efficiency, and improving processes in healthcare organizations. By measuring these outcomes, providers can validate their AI investments and identify further integration opportunities, ultimately supporting better patient care and driving organizational growth.
Challenges and considerations in adopting generative AI for healthcare
Generative AI holds great promise for transforming healthcare, but its adoption comes with several key challenges that need careful consideration:
1. Bias and fairness: Generative AI models can reflect biases present in their training data. If the dataset lacks diversity, the GenAI may produce outcomes that disadvantage underrepresented groups. To address this, healthcare organizations should prioritize inclusive datasets and implement rigorous validation protocols, along with ongoing monitoring for bias and fairness, to ensure equity in healthcare delivery.
2. Information privacy and security: GenAI relies heavily on sensitive patient data, raising significant privacy concerns. Organizations must comply with strict data protection regulations like HIPAA in the USA and implement robust security measures, including encryption, access controls and regular audits. Additionally, transparent data-sharing policies and anonymization of patient data are crucial for building trust and protecting privacy.
3. Regulatory compliance: Deploying generative AI in healthcare must comply with complex regulatory requirements. Healthcare providers must navigate an intricate landscape of industry standards and secure necessary approvals from regulatory bodies. Compliance with medical device regulations, data protection laws, and guidelines for AI utilization is paramount to ensuring safe and effective implementation.
4. Integration with legacy systems: Many healthcare organizations operate on legacy IT systems that may not be compatible with advanced AI technologies. The integration process can disrupt established workflows and meet resistance from healthcare professionals accustomed to traditional methods. A thorough assessment of existing infrastructure and careful planning for interoperability between gensystems and legacy platforms is crucial to overcome these hurdles.
5. Diagnosis challenges: Accurate diagnosis, particularly for rare diseases, poses significant challenges for GenAI systems, which may struggle due to limited data representation. Ensuring that GenAI models have access to comprehensive and diverse datasets is critical for improving diagnostic accuracy. Large healthcare systems with extensive proprietary data may dominate the landscape, creating disparities in access to effective GenAI tools for smaller entities.
6. Accuracy and reliability: GenAI can produce syntactically accurate but factually incorrect outputs, raising concerns about its reliability in clinical settings. Healthcare providers must establish protocols for determining the acceptability of GenAI-generated errors and ensure that GenAI tools can justify their recommendations. The stakes are high in healthcare; thus, maintaining a high standard of accuracy is non-negotiable.
7. Treatment planning limitations: GenAI applications in treatment planning raise several challenges, including accountability, patient trust, and the ability to replicate the nuanced decision-making of human physicians. As healthcare providers are ultimately responsible for treatment outcomes, establishing clear lines of accountability when using GenAI in treatment decisions is essential. Moreover, increasing patient acceptance of GenAI-driven treatments is vital for widespread adoption.
8. Ensuring transparency and explainability: Transparency in GenAI decision-making processes is critical for building trust among healthcare providers and patients. GenAI systems should provide clear explanations for their outputs to enhance accountability and foster confidence in their recommendations. Ensuring that healthcare professionals can understand and interpret genAI-generated insights is essential for effective collaboration.
Successfully implementing generative AI in healthcare requires a careful balance of innovation and responsibility. By addressing these challenges, healthcare providers can improve patient care, streamline operations, and build trust in genAI-driven solutions for the future of medicine.
Optimize Your Operations With AI Agents
Our AI agents streamline your workflows, unlocking new levels of business efficiency!
Best practices for implementing generative AI in healthcare operations
As generative AI continues to transform healthcare operations, building trust among stakeholders is crucial for successful implementation. Trust fosters collaboration, enhances patient care, and ensures compliance with regulatory standards. To achieve this, organizations should adopt best practices that prioritize transparency, data privacy, and ethical guidelines. By embracing these principles, healthcare providers can effectively leverage generative AI to improve outcomes while addressing the concerns and expectations of all involved.
1. Ensure transparency
- Explain AI decisions: Clearly communicate how GenAI systems make decisions and provide insights. Use interpretable models where possible, and share the rationale behind GenAI-generated outcomes.
- Open data access: Allow stakeholders access to relevant datasets (while ensuring patient privacy) to promote transparency in how the GenAI solution was trained and how it operates.
2. Focus on data privacy and security
- Compliance with regulations: Adhere to HIPAA and GDPR regulations to protect patient information and implement robust data encryption and security measures.
- Anonymization techniques: Use anonymization methods to protect sensitive data during GenAI training and operations, ensuring that individual identities cannot be traced.
3. Involve stakeholders early
- Collaborative development: Engage healthcare professionals, patients, and other stakeholders in the design and implementation phases. Gather their input on needs, concerns, and expectations.
- Training programs: Develop training programs to educate healthcare staff on the capabilities and limitations of generative AI, enhancing their comfort and trust in using these technologies.
4. Establish ethical guidelines
- Create an ethical framework: Develop and communicate a clear set of ethical guidelines for GenAI use in healthcare, focusing on fairness, accountability, and non-discrimination.
- Regular audits: Conduct regular audits and evaluations of GenAI systems to ensure adherence to ethical standards and identify areas for improvement.
5. Prioritize explainability and interpretability
- Build explainable models: Choose generative AI models that can provide explanations for their outputs, helping healthcare professionals understand the basis for recommendations.
- User-friendly interfaces: Design intuitive user interfaces that allow healthcare workers to easily interact with and interpret AI outputs.
6. Implement robust validation processes
- Rigorous testing: Thoroughly test GenAI systems using diverse datasets to validate their performance, reliability, and generalizability across different patient populations.
- Clinical trials: Where appropriate, conduct clinical trials to assess the impact of GenAI tools on patient outcomes and operational efficiency.
7. Communicate benefits and limitations
- Transparent communication: Clearly articulate the benefits of generative AI, such as improved diagnostics and personalized treatment plans, while discussing its limitations and potential risks.
9. Emphasize human oversight
- Hybrid approaches: Encourage a hybrid model where GenAI solutions support human decision-making rather than replacing it, reinforcing the importance of human expertise in patient care.
- Clinical governance: Establish clear protocols for clinical governance to ensure that healthcare professionals retain control over critical decision-making processes.
By implementing these best practices, healthcare organizations can build trust in generative AI technologies, ensuring they enhance patient care and operational efficiency while addressing ethical and regulatory concerns.
Future outlook of generative AI in healthcare
The future of generative AI in healthcare will bring about significant advancements, transforming how care is delivered and experienced. As the industry embraces these technologies, we can expect a shift towards more integrated and intelligent healthcare solutions. Generative AI has the potential to seamlessly integrate with existing systems, creating a layer of intelligence that enhances workflows and improves patient interactions. By automating routine tasks and providing real-time insights, these solutions will reduce the cognitive load on healthcare professionals, allowing them to focus on patient care and strategic initiatives.
In the long term, generative AI can enhance decision-making and automate various aspects of healthcare delivery.
- Level 1 applications, which involve basic automation of administrative tasks and data entry, will pave the way for more advanced Level 2 applications that synthesize vast amounts of medical data for precise diagnoses and personalized treatment plans. This comprehensive automation streamlines administrative processes and supports clinical decision-making, improving healthcare outcomes and patient satisfaction.
- Level 3 applications will see GenAI playing a critical role in clinical workflows, such as automating utilization management and supporting advanced clinical decision-making, which streamlines processes and enhances patient outcomes.
- At Level 4, generative AI will facilitate more complex interactions, including predictive analytics to identify at-risk patients and GenAI-driven tools for remote monitoring and telehealth.
- Finally, Level 5 applications will encompass a fully integrated healthcare ecosystem where generative AI provides comprehensive solutions, from patient education to personalized health management.
This evolution will improve operational efficiency and enhance the overall patient experience, leading to more proactive and preventive care. The trajectory toward generative AI in healthcare promises a future where care is more responsive, integrated, and centered on patients’ needs.
Transform healthcare with full-stack generative AI
ZBrain, with its AI capabilities, helps healthcare organizations streamline operations, enhance patient care, and improve compliance. It provides a range of features aimed at automating processes, ensuring accuracy, and supporting informed decision-making.
-
AI readiness assessment: ZBrain’s AI readiness assessment framework, ZBrain XPLR, evaluates an organization’s preparedness for AI adoption in healthcare. It offers actionable insights to help healthcare providers identify opportunities for AI-driven improvements in patient care, administrative processes, and regulatory compliance.
-
Low-code development: ZBrain’s low-code platform, ZBrain Builder, simplifies the creation of custom AI solutions for healthcare applications, such as automated documentation, medical record analysis, and claims processing, making AI adoption accessible without extensive technical expertise.
-
Proprietary data utilization: The platform enables healthcare providers to leverage their proprietary patient data securely, ensuring AI-driven solutions are aligned with clinical workflows, regulatory requirements, and patient privacy standards.
-
Enterprise-ready: ZBrain Builder is designed for healthcare environments, offering features such as security, scalability, and seamless integration with electronic health records (EHR), hospital management systems, and other healthcare IT infrastructure.
-
End-to-end support: ZBrain Builder supports the entire AI lifecycle in healthcare, from development to deployment and continuous optimization, ensuring efficient implementation and adaptability to evolving medical and regulatory needs.
-
Flexible data ingestion: ZBrain integrates data from multiple sources, including patient records, medical literature, and administrative documents, enabling AI-driven insights that support clinical decision-making, operational efficiency, and compliance with healthcare regulations.
-
Intelligent agent creation: AI agents built on ZBrain Builder assist in automating critical healthcare tasks such as patient data processing, medical coding, prior authorization management, and clinical documentation, reducing administrative burden and allowing healthcare professionals to focus on patient care.
These capabilities position ZBrain as a valuable tool for healthcare organizations, enabling AI-driven automation and decision support while ensuring compliance, accuracy, and efficiency in healthcare operations.
Endnote
The integration of generative AI in healthcare represents a transformative shift in how healthcare organizations deliver care and manage operations. As highlighted throughout this article, GenAI offers unprecedented opportunities to improve patient engagement, improve diagnostic accuracy, and streamline administrative processes. By automating routine tasks and leveraging data-driven insights, healthcare providers can focus on what matters most: delivering high-quality patient care. This transformative technology is not just an enhancement; it is a critical enabler for the future of healthcare.
As the generative AI landscape evolves, healthcare organizations adopting this technology will be better positioned to thrive in a competitive environment. Platforms like ZBrain enable healthcare providers to develop AI agents and seamlessly integrate them into existing workflows, ensuring a smooth transition while enhancing efficiency and care delivery. As AI continues to evolve, these intelligent agents will play an even greater role in automating complex tasks, optimizing decision-making, and shaping the future of patient-centered healthcare. With a focus on operational excellence and strong security measures, ZBrain can help organizations harness the full potential of GenAI while ensuring patient safety and compliance with industry regulations.
The imperative is clear: healthcare organizations must actively explore and implement generative AI solutions to remain competitive and responsive to patient needs. By investing in advanced technologies and platforms like ZBrain, healthcare leaders can unlock new efficiencies and drive meaningful change within their operations. The time for action is now—embracing generative AI is not just an option but a necessity for those committed to improving patient outcomes and revolutionizing healthcare practices.
Excited to transform your healthcare operations with GenAI? Build custom AI agents with ZBrain to enhance efficiency and patient care!
Listen to the article
Author’s Bio
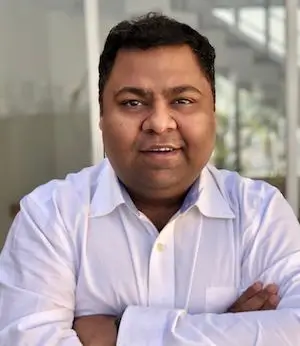
An early adopter of emerging technologies, Akash leads innovation in AI, driving transformative solutions that enhance business operations. With his entrepreneurial spirit, technical acumen and passion for AI, Akash continues to explore new horizons, empowering businesses with solutions that enable seamless automation, intelligent decision-making, and next-generation digital experiences.
Table of content
- What is generative AI?
- Why is generative AI crucial in modern healthcare?
- The current landscape of GenAI in healthcare
- Different approaches to integrating generative AI into healthcare systems
- Generative AI use cases for healthcare
- Why is ZBrain the ideal platform for healthcare organizations?
- Measuring the ROI of generative AI in healthcare
- Challenges and considerations in adopting generative AI for healthcare
- Best practices for implementing generative AI in healthcare operations
- Future outlook of generative AI in healthcare
- Transform healthcare with full-stack generative AI
Frequently Asked Questions
What is ZBrain, and how can it optimize healthcare operations with gen AI?
ZBrain is an end-to-end AI enablement platform that helps healthcare organizations seamlessly integrate AI into their operations, enhancing patient care, streamlining workflows, and improving decision-making. From assessing AI readiness to deploying intelligent solutions, ZBrain empowers healthcare teams to boost efficiency, enhance patient experiences, and align operational strategies with organizational goals.
Here’s how ZBrain enhances healthcare operations:
AI readiness assessment with ZBrain XPLR: ZBrain XPLR offers a comprehensive AI readiness assessment, assisting healthcare teams in evaluating current processes and identifying areas for AI-driven optimization. This strategic approach ensures effective AI adoption, enhancing patient care, operational efficiency, and regulatory compliance.
Seamless data ingestion and integration: ZBrain Builder integrates with electronic health records (EHR), billing systems, and other healthcare applications to unify data from multiple sources. This integration facilitates real-time analytics, enabling healthcare professionals to make data-driven decisions on patient care, resource allocation, and treatment planning.
Low-code development environment: ZBrain Builder’s intuitive, low-code interface allows healthcare professionals to create and deploy AI-driven solutions with minimal technical expertise. This capability accelerates automation in key processes, such as patient scheduling, medical coding, and administrative tasks.
Cloud and model flexibility: ZBrain Builder supports advanced AI models like GPT-4 and LLaMA and integrates with cloud platforms such as AWS, Azure, and GCP. This flexibility enables healthcare teams to deploy scalable AI solutions tailored to their organization’s unique needs.
Enhanced compliance and governance: ZBrain’s AI-driven analytics assist healthcare teams in ensuring compliance with healthcare regulations and internal policies. By continuously monitoring operations, ZBrain identifies potential risks related to data privacy and service quality, supporting adherence to compliance standards.
By combining powerful AI capabilities with seamless data integration, ZBrain enables healthcare organizations to optimize operations, improve patient outcomes, and create a more agile, future-ready healthcare environment.
How does ZBrain ensure the security and privacy of sensitive data in healthcare operations?
ZBrain is designed with a strong focus on data privacy and security, ensuring that sensitive healthcare information—such as patient records, medical histories, and treatment plans—is protected at all stages. Here’s how ZBrain safeguards healthcare data throughout the operational lifecycle:
Private cloud deployments: ZBrain AI agents can be deployed in a private cloud environment, ensuring that critical healthcare data remains securely stored within the organization’s infrastructure.
Robust security features: ZBrain incorporates multiple layers of security to protect sensitive healthcare data, including:
-
Access controls: Granular role-based access controls ensure only authorized medical personnel can view or manage confidential patient data.
-
Anonymization and compliance monitoring: AI-driven compliance tools help healthcare teams anonymize sensitive data where necessary and ensure alignment with data privacy regulations.
Compliance and governance: ZBrain is built to meet global compliance standards ISO 27001:2022 and SOC 2 Type II, ensuring that healthcare data is managed with confidentiality, integrity, and accountability.
By integrating these security and compliance measures, ZBrain enables healthcare teams to leverage AI-driven operations while ensuring that patient data remains protected and regulatory requirements are met.
Can ZBrain AI agents be integrated with existing healthcare systems?
Yes, ZBrain AI agents are designed to integrate seamlessly with existing healthcare systems, including electronic health records (EHRs), practice management software, medical billing platforms, and diagnostic tools. The platform supports various data formats and standards, ensuring smooth interoperability with both modern and legacy healthcare technologies.
This integration enables healthcare organizations to:
-
Leverage existing infrastructure: Enhance current clinical workflows and administrative processes without a complete overhaul of existing systems.
-
Enrich data and workflows: Connect ZBrain AI agents with existing tools to automate patient data analysis, appointment scheduling, billing processes, and compliance monitoring.
-
Drive AI-driven insights: Utilize gen AI capabilities to predict patient health trends, optimize treatment plans, and improve clinical decision-making while maintaining compatibility with current technologies.
By enabling seamless integration, ZBrain ensures that healthcare teams can modernize their operations without disrupting existing healthcare ecosystems, leading to improved efficiency, data-driven decision-making, and enhanced patient care.
What AI agents can be built on ZBrain for healthcare operations?
ZBrain enables organizations to build genAI-powered healthcare agents that enhance decision-making, automate critical healthcare processes, and optimize patient care strategies. With ZBrain Builder, healthcare providers can develop AI agents tailored to various healthcare functions, ensuring seamless integration with existing healthcare systems and data sources.
Key AI agents that can be built on ZBrain:
-
Patient data analysis agent: Processes and analyzes patient records to assist in diagnosis, treatment planning, and personalized care recommendations.
-
Appointment scheduling optimization agent: Automates the scheduling process, reduces no-shows, and optimizes resource allocation for healthcare providers.
-
Medical billing and coding agent: Automates billing processes, ensures accurate coding, and assists in revenue cycle management.
-
Patient engagement and sentiment analysis agent: Analyzes patient feedback to assess satisfaction levels and identify areas for improvement in care delivery.
-
Healthcare compliance monitoring agent: Tracks policy adherence, flags regulatory risks, and ensures healthcare practices align with legal requirements.
By leveraging ZBrain’s AI-driven automation and analytics, healthcare organizations can streamline operations, enhance patient experiences, and build more responsive, data-driven healthcare strategies.
How does ZBrain cater to diverse healthcare operational needs across medical practices?
ZBrain’s flexibility enables healthcare organizations to build AI-driven solutions tailored to various operational needs, from patient data management and clinical decision support to administrative automation and compliance. With custom AI agents, healthcare teams can analyze patient records, automate appointment scheduling, optimize billing processes, assess patient sentiment, and ensure regulatory compliance. By leveraging ZBrain’s intelligent automation and data-driven insights, medical practices can create more strategic, agile, and efficient operations, driving better decision-making and patient outcomes.
How can we measure the ROI of ZBrain in our healthcare operations and strategy?
Measuring the ROI of ZBrain in healthcare operations and strategy involves assessing key performance indicators (KPIs) related to automation, patient care optimization, and strategic decision-making. Key metrics include:
-
Reduced manual effort: AI-driven automation in administrative tasks, such as appointment scheduling and billing, leads to faster processing and fewer errors.
-
Improved patient outcomes: Enhanced diagnostic accuracy and personalized treatment plans can be measured by analyzing patient recovery rates and satisfaction scores.
-
Enhanced decision-making: Data-driven insights for patient management, resource allocation, and clinical workflows contribute to more informed decisions.
-
Operational efficiency: AI-powered analytics, automated reporting, and streamlined healthcare workflows improve overall efficiency.
By tracking these KPIs, healthcare organizations can quantify ZBrain’s impact on streamlining processes, enhancing patient care strategies, and driving better health outcomes.
How can I get started with ZBrain for healthcare operations and strategy?
To leverage ZBrain for optimizing your healthcare operations and strategy, contact us at hello@zbrain.ai or fill out the inquiry form on our website. Our team will connect with you to discuss how ZBrain can integrate with your existing healthcare systems, automate key processes, and enhance patient care, clinical workflows, and compliance strategies.
Insights
How ZBrain drives seamless integration and intelligent automation
ZBrain is designed with integration and extensibility as core principles, ensuring that it can fit into existing ecosystems and adapt to future requirements.
Enhancing enterprise AI with a centralized agent store: ZBrain’s solution
ZBrain’s AI Agent Store addresses key enterprise pain points by providing a centralized, governed, and scalable solution for building, deploying, and managing AI agents.
Understanding ambient agents
Ambient agents are AI systems designed to run continuously in the background, monitoring streams of events and acting on them without awaiting direct human prompts.
How ZBrain accelerates AI development and deployment
ZBrain addresses the comprehensive AI development lifecycle with an integrated platform composed of distinct yet interconnected modules that ensure enterprises accelerate AI initiatives while maintaining strategic alignment, technical feasibility, and demonstrable value.
How to build AI agents with ZBrain?
By leveraging ZBrain’s blend of simplicity, customization, and advanced AI capabilities, organizations can develop and deploy AI agents that are both powerful and tailored to meet unique business demands, enhancing productivity and operational intelligence across the board.
How to build a search-optimized knowledge repository with ZBrain
ZBrain’s advanced knowledge base is engineered to scale with your enterprise needs, whether you’re handling a few thousand records or millions of documents, without compromising performance or uptime.
How ZBrain enhances knowledge retrieval with intelligent reranking
ZBrain significantly enhances the relevance of enterprise search results through reranking, acting as an intelligent gatekeeper between user queries and corporate data.
Monitoring ZBrain AI agents: Exploring key metrics
AI agent monitoring is the practice of systematically tracking and analyzing an agent’s behavior, outputs, and impact to ensure it operates reliably, fairly, and efficiently.
How ZBrain’s multi-agent collaboration works
ZBrain’s multi-agent collaboration is an advanced approach in which multiple specialized AI agents work together as a cohesive team to solve complex enterprise challenges.