Understanding vertical AI agents: Applications, challenges, implementations and opportunities
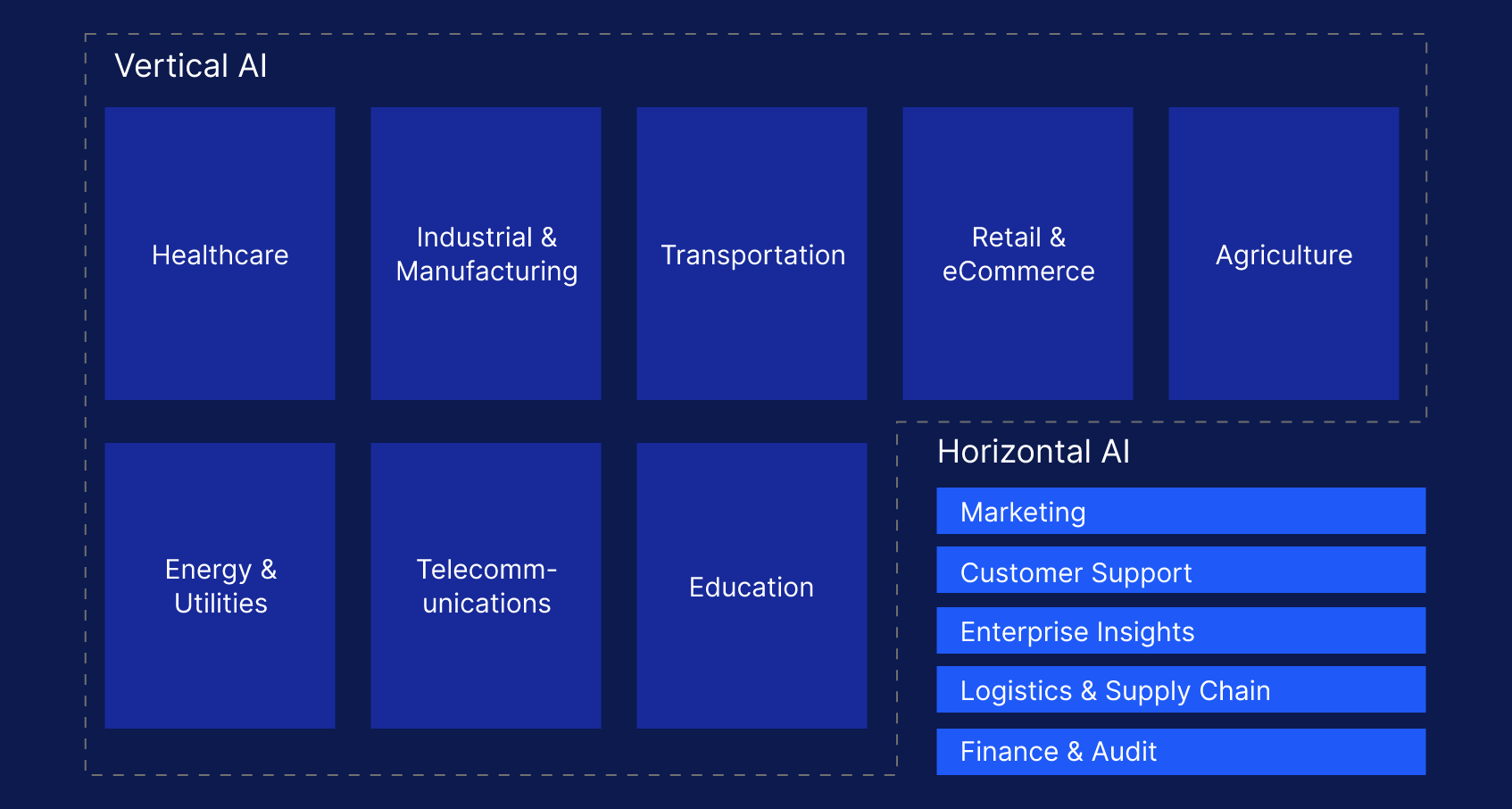
Listen to the article
While the artificial intelligence market is experiencing rapid growth, vertical AI remains a largely untapped domain with immense potential. Traditional software solutions, particularly SaaS offerings, often struggle to effectively address the unique challenges faced by specific industries. Industries reliant on unstructured data (like legal documents or medical records), those with small total addressable markets (TAMs), or those characterized by long sales cycles and resistance to change were largely underserved. This left many sectors ripe for disruption—a situation vertical AI agents, with their focus on industry-specific solutions powered by advanced language models, are now changing.
The evidence of this shift is compelling. Early data from vertical AI companies founded since 2019 demonstrates remarkable growth: achieving 80% of the average contract value (ACV) of established vertical SaaS systems and experiencing year-over-year growth exceeding 400%, all while maintaining healthy margins. This isn’t merely theoretical; significant M&A activity confirms this explosive growth. The $650 million acquisition of CaseText by Thomson Reuters and DocuSign’s $165 million acquisition of Lexion are just two examples illustrating the immense value being created in this space. Furthermore, established companies are not passive observers; a recent survey confirms that a significant number of investors in vertical SaaS report their portfolio companies are rapidly integrating AI features. The market has moved beyond being ripe for disruption and is now in the midst of a profound transformation. The next two to three years are projected to see at least five vertical AI companies reach $100M+ in annual recurring revenue (ARR), with the first IPO expected within the same timeframe. This isn’t a future prediction; it’s a present-day reality, underscoring the urgency for businesses to understand and capitalize on the vertical AI opportunity.
This insight discusses the potential of vertical AI agents, the key considerations for successful vertical AI adoption, outlining the hurdles to overcome and the strategies to employ for achieving lasting success.
- What are vertical AI agents?
- Vertical AI vs. horizontal AI vs. SaaS
- The vertical AI opportunity: Why now?
- Why are vertical AI agents important?
- Vertical LLM agents: The next big thing
- Challenges and considerations in vertical AI adoption
- Overcoming the challenges of vertical AI agent implementation with ZBrain
- ZBrain's prebuilt agents: An immediate solution to operational challenges across industries
- Strategic considerations for success with vertical LLM agents
What are vertical AI agents?
Vertical AI agents are systems designed to carry out industry-specific tasks. Unlike horizontal AI, which focuses on cross-functional applications (like marketing automation applicable across sectors), vertical AI leverages industry-specific data and domain expertise to deliver the solutions. This enables them to comprehend the industry’s nuances and perform tasks better than general AI systems. They often seamlessly integrate with existing workflows and systems, automating complex processes previously requiring human intervention.
Examples of vertical AI agents include AI legal assistants, AI-powered diagnostic tools in healthcare, and AI systems designed to optimize manufacturing processes. Their strength lies in their specialized knowledge of a specific domain, enabling them to deliver highly accurate results and operate with exceptional efficiency.
Vertical AI vs. horizontal AI vs. SaaS
-
Vertical AI vs. horizontal AI: The key difference lies in scope. Horizontal AI aims for broad applicability across industries, while vertical AI is deeply specialized. Both are valuable, and the most powerful AI solutions will likely integrate both approaches.
-
Vertical AI vs. SaaS: While Software-as-a-service (SaaS) provides software solutions, vertical AI agents automate entire business processes, integrating software and human tasks. This represents a paradigm shift: SaaS delivers tools; vertical AI delivers automated outcomes. Vertical AI could significantly surpass the impact of SaaS, potentially automating the functions and tasks of an entire team. SaaS might become a component within the broader vertical AI ecosystem.
The vertical AI opportunity: Why now?
The convergence of several key factors has created an unprecedented opportunity for vertical AI. While general-purpose AI models like those from OpenAI have shown remarkable capabilities, their true potential is unleashed when applied to the specific needs and challenges of individual industries. This focus on specialized domains is what defines vertical AI, creating powerful tools that drive transformation across sectors. The landscape is ripe for disruption, and the timing is ideal for several reasons:
- The maturation of Large Language Models (LLMs): Recent breakthroughs in LLMs have provided the foundational technology necessary for building sophisticated multimodal vertical AI agents. These models now possess the linguistic capabilities to understand and process complex, industry-specific data, automating tasks previously considered too intricate for automation. The evolution, particularly advancements like GPT-4, Gemini, Gemma and more, have significantly lowered the barrier to entry for creating highly functional vertical AI solutions.
- Addressing previously unsolvable problems: Many industries have long grappled with inefficiencies from outdated technologies, cumbersome workflows, and the sheer volume of unstructured data. Traditional software solutions, including SaaS, often fail to provide adequate solutions due to their general-purpose nature and inability to handle the complexities of these specific domains. Vertical AI directly addresses these pain points, providing solutions that improve efficiency and decision-making within existing workflows.
- A shift in startup strategies: The rise of vertical AI is also accompanied by a shift in startup strategies. The traditional model of scaling up by hiring hundreds or thousands of employees to manage significant revenue is evolving. Now, companies are increasingly leveraging the power of LLMs to automate processes, dramatically reducing the need for large teams. This new paradigm allows for the creation of highly efficient companies capable of significant growth with leaner structures—a pivotal shift made possible by advancements in this field.
- Increased market receptivity: While the concept of vertical AI is not new, recent technological advancements coupled with increased industry awareness and a growing need for efficiency and improved decision-making have created a receptive market environment. Businesses are actively seeking ways to leverage AI to gain a competitive edge, creating a robust demand for sophisticated vertical solutions.
- The role of platforms like ZBrain: The development and deployment of effective vertical AI agents requires overcoming significant technical challenges, from data acquisition and management to model training and seamless system integration. Platforms like ZBrain are accelerating this process by offering the tools and infrastructure needed to build and deploy custom vertical AI agents quickly and efficiently. The availability of pre-built, customizable agents further lowers the barriers to entry, allowing businesses to access the benefits of vertical AI readily.
In summary, this is a unique convergence of technological advancements, market demand, and supportive infrastructure. The potential for creating significant value through vertical AI is immense, and this opportunity is poised for explosive growth.
Optimize Your Operations With AI Agents
Our AI agents streamline your workflows, unlocking new levels of business efficiency!
Why are vertical AI agents important?
Vertical AI agents are crucial for several reasons, all stemming from their ability to deliver highly specialized and efficient solutions to industry-specific problems:
-
High efficiency and productivity gains: Vertical AI agents automate complex, repetitive tasks that previously consumed significant human time and resources. This leads to significant increases in productivity across various departments, freeing human employees to focus on higher-value, strategic initiatives that require creativity, critical thinking, and complex decision-making. For instance, in the legal sector, AI agents can automate contract review, freeing up lawyers to focus on client strategy and case development. In healthcare, they can automate administrative tasks, allowing doctors to spend more time with patients.
-
Enhanced accuracy and decision-making: Trained on vast amounts of industry-specific data, vertical AI agents can make more accurate and consistent decisions than humans, reducing errors and improving overall outcomes. This is especially critical in fields like finance, where small errors can have significant consequences, or in healthcare, where accurate diagnoses are paramount.
-
Unlocking untapped markets: Traditional software solutions often struggled to penetrate industries with unstructured data, complex workflows, or limited budgets. Vertical AI agents, with their ability to handle unstructured data and their targeted approach, open up new markets and allow businesses to reach previously underserved customer segments. This expansion of the addressable market creates significant new revenue opportunities.
-
Improved customer experience: By automating tasks and providing personalized service, vertical AI agents enhance customer experiences. Faster response times, personalized recommendations, and more efficient problem-solving all contribute to increased customer satisfaction and loyalty.
-
Competitive advantage: Companies that effectively leverage vertical AI agents gain a significant competitive advantage. They can work more efficiently, make improved decisions, and offer superior products or services, outpacing competitors who haven’t embraced this technology. This translates directly to increased profitability and market share.
-
Driving innovation: Vertical AI agents not only automate existing processes but also enable new forms of innovation. By assessing large amounts of data and identifying patterns, they can help companies develop new products, services, and business models, leading to breakthroughs and growth.
In short, vertical AI agents are not just a technological advancement; they are a business imperative. Their ability to drive efficiency, enhance accuracy, unlock new markets, and foster innovation makes them indispensable for companies aiming to excel in the rapidly evolving business landscape.
Vertical LLM agents: The next big thing
Vertical LLM agents represent a massive, largely untapped market opportunity. Their ability to solve complex, high-value problems within specific industries, combined with high barriers to entry and increasing market demand, creates the potential for billion-dollar companies. This potential is driven by:
-
Tackling high-value, complex problems: Vertical AI agents address niche problems within industries that general-purpose AI struggles to solve effectively. This focus on high-stakes challenges, such as legal research, medical diagnosis, or complex financial analysis, delivers immense value, justifying premium pricing and driving significant revenue.
-
Leveraging deep domain expertise: Unlike generic models, vertical LLM agents integrate industry-specific data and knowledge. This specialization results in more accurate, reliable, and contextually relevant outputs, creating a strong competitive advantage that’s difficult for general-purpose AI to replicate.
-
High barriers to entry: Developing a successful vertical AI agent requires significant domain expertise, proprietary data, and seamless integration with existing industry systems. These high barriers to entry protect market share and enable companies to command premium pricing.
-
Transforming workflows and increasing productivity: Vertical LLM agents are transforming how professionals work. By automating mundane tasks and providing advanced analytics, they free up valuable time for strategic thinking and high-level decision-making, leading to substantial increases in efficiency and productivity. This translates to quantifiable cost savings and revenue generation for businesses.
-
Market readiness and demand: The increasing awareness of AI’s potential and a growing need for efficiency and accuracy have created a receptive market. Even traditionally resistant industries actively seek AI-driven solutions, presenting a massive opportunity for early movers.
Challenges and considerations in vertical AI adoption
While the potential of vertical AI is immense, realizing that potential requires navigating a complex landscape of challenges. Successfully deploying and scaling vertical AI solutions demands careful consideration of several key areas:
Data acquisition and quality: High-quality, industry-specific data is the lifeblood of any successful vertical AI solution. Gathering, cleaning, and labeling this data can be time-consuming, expensive, and difficult. Access to proprietary datasets offers a competitive advantage, but obtaining them requires significant effort and resources. Furthermore, ensuring data quality and addressing potential biases is crucial for building reliable and fair AI systems. Incomplete or biased data can result in unreliable predictions and unreliable results.
Ensuring accuracy and reliability: LLMs are susceptible to “hallucinations”—generating inaccurate or nonsensical information. This is unacceptable in many industries, particularly those with high stakes, like healthcare or finance. Rigorous testing, prompt engineering, fine-tuning using domain-specific data and continuous validation are crucial to minimize errors and ensure the reliability of vertical AI agents. This often involves incorporating human oversight and feedback loops to identify and correct inaccuracies.
Integration with existing systems: For successful adoption, vertical AI agents must integrate seamlessly into existing workflows and systems. This requires careful consideration of compatibility with legacy systems, data formats, and existing processes. Custom integrations and robust APIs are often necessary to make sure data flows smoothly and minimize disruption to established practices.
Domain expertise and model fine-tuning: Vertical AI agents must possess deep domain knowledge to understand the nuances of a specific industry and its workflows. Simply wrapping a general-purpose LLM in an industry-specific interface is insufficient. Significant effort must be dedicated to fine-tuning the model using relevant data and incorporating industry-specific terminology, regulations, and best practices. This requires close collaboration between AI specialists and domain experts.
Explainability and transparency: Understanding how a vertical AI agent arrives at its conclusions is crucial for building trust and accountability. The “black box” nature of some AI models makes it hard to explain their decision-making processes, leading to concerns about transparency and potential biases. Explainable AI (XAI) is an active area of research, but robust solutions are still needed to address this challenge fully.
Ethical considerations: The ethical implications of vertical AI must be carefully considered. Potential biases in training data, concerns about job displacement, and issues related to data privacy and security all require careful attention. Developing and deploying vertical AI solutions responsibly is crucial for avoiding unintended negative consequences.
Addressing resistance to change: Introducing AI into established industries often faces resistance from employees concerned about job displacement or skeptical of the technology’s capabilities. Overcoming this requires clear communication, demonstrating the value proposition of the AI agent, highlighting early successes, and addressing employee concerns through training and reskilling initiatives. Showcasing how AI augments human capabilities rather than replacing them can ease anxieties.
Scalability and infrastructure: As demand for vertical AI solutions grows, ensuring scalability becomes crucial. The foundational infrastructure must be capable of handling increasing workloads and data volumes without compromising performance. Investing in robust infrastructure, optimizing algorithms for efficiency, and employing cloud-based solutions are essential for supporting growth and maintaining system responsiveness.
Realizing the full potential of vertical AI requires addressing these key considerations. Companies that do so effectively will be strategically positioned to leverage the vast opportunities offered by this transformative technology.
Overcoming the challenges of vertical AI agent implementation with ZBrain
Building and deploying effective vertical AI agents presents significant challenges: acquiring and managing industry-specific data, developing and training accurate models, and ensuring seamless integration with existing systems. These complexities often require significant time, resources, and specialized expertise—a significant hurdle for many organizations.
ZBrain addresses these challenges, providing a powerful platform for both rapid development and deployment of custom vertical AI agents and access to a library of pre-built, customizable agents.
-
Build custom AI agents: ZBrain’s low-code development environment simplifies the process of creating custom AI agents. With ZBrain, companies can integrate their proprietary data, define workflows, and deploy their custom vertical agents in a fraction of the time required for traditional development.
-
Leverage prebuilt agents: ZBrain’s library of pre-built vertical AI agents delivers immediate value. These agents, based on specific industry verticals (legal, finance, HR, etc.), are designed to automate common tasks, improve decision-making, and boost productivity. Businesses can readily adapt these agents to their exact requirements, integrate them with their existing systems, and get immediate value without lengthy development cycles.
ZBrain’s powerful features, including seamless data integration, knowledge base integration, and continuous improvement through reinforcement learning from human feedback, ensure your agents are accurate, efficient, and continually evolving to meet your changing needs.
Optimize Your Operations With AI Agents
Our AI agents streamline your workflows, unlocking new levels of business efficiency!
ZBrain’s prebuilt agents: An immediate solution to operational challenges across industries
ZBrain offers a range of agents designed to address common pain points in specific industry verticals and accelerate value delivery. Here are some examples:
Legal:
-
Contract review summary agent: Generates concise summaries of complex contracts, highlighting key terms and potential risks.
-
Contract compliance check agent: Validates contracts against compliance standards, ensuring adherence to regulations.
-
Regulatory gap analysis agent: Identifies compliance gaps between company policies and current regulations.
-
PII redaction agent: Automates the redaction of Personally Identifiable Information (PII) from legal documents.
-
Legal document filing agent: Automates legal document filing, ensuring accurate metadata tagging and efficient retrieval.
Finance:
-
Client payment scheduling agent: Suggests optimal payment schedules for clients based on financial forecasts and payment history.
-
Variance analysis agent: Automatically analyzes budget vs. actual spending variances, providing detailed reports.
-
Cash flow monitoring agent: Provides real-time insights into cash inflows and outflows, reducing liquidity risks.
-
Invoice validation agent: Automatically verifies invoices against purchase orders and delivery records, flagging discrepancies.
Human resources:
-
Onboarding handbook generator agent: Creates customized employee handbooks according to your company policies and job roles.
-
Training material compiler agent: Compiles training materials specific to a new hire’s role, gathering content from various sources.
-
Performance review prep guide agent: Generates personalized performance review guides, summarizing goals and achievements.
Customer service:
-
Next step suggestion agent: Provides recommended next steps for support tickets based on ticket type and history.
-
FAQ generation agent: Automatically creates FAQs from helpdesk tickets and resolutions.
-
Customer satisfaction survey agent: Automates post-interaction surveys to gather feedback and improve service quality.
Procurement:
-
Order entry management agent: Automates purchase order creation and entry, boosting procurement efficiency and minimizing errors.
-
Supplier performance monitoring agent: Monitors supplier performance based on key metrics (delivery times, quality, compliance).
-
Contract amendment monitoring agent: Tracks and documents changes to procurement contracts, ensuring compliance.
-
Vendor onboarding agent: Streamlines vendor onboarding by automating document collection and verification.
-
Vendor data validation agent: Ensures accuracy and compliance by validating vendor data.
Information technology:
-
SLA compliance monitoring agent: Monitors service level agreements (SLAs) and alerts teams to potential breaches.
-
Incident documentation generator agent: Automates initial security incident response documentation.
-
Code quality analysis agent: Automatically reviews code for errors, security vulnerabilities, and inefficiencies.
These are just a few examples: ZBrain’s library of ready-made agents is constantly expanding to cover a broader range of industries and functions. Each agent is designed to be highly customizable, enabling seamless integration with your current systems and tailoring its functionality to meet your unique business needs. This combination of ready-made solutions and robust customization options empowers businesses to realize the transformative potential of vertical AI quickly.
Strategic considerations for success with vertical LLM agents
Capitalizing on the transformative potential of vertical LLM agents requires a strategic and proactive approach. Here are key steps to consider:
-
Identify your niche: Focus on specific areas where AI can deliver the greatest value within your industry.
-
Invest in expertise: Building effective vertical AI solutions requires both AI expertise and deep industry knowledge.
-
Foster innovation: Encourage experimentation and continuous learning to adapt to evolving industry practices.
-
Form strategic alliances: Collaborate with other organizations to access cutting-edge technologies and insights.
-
Develop a clear AI strategy: Align AI adoption with overall business goals and values.
Endnote
Vertical AI is not just a technological advancement; it’s a fundamental shift in the way businesses function and compete. The ability to automate complex tasks, improve decision-making, and unlock new levels of efficiency is transforming industries and creating immense market opportunities. As AI models continue to advance and the availability of industry-specific data increases, the potential for innovation and disruption will only intensify. Businesses that embrace vertical AI now will be optimally positioned to succeed in this rapidly changing landscape. The time to act is now.
Looking for AI solutions tailored to your industry? ZBrain’s custom vertical AI agents address specific challenges with precision, efficiency and accuracy to optimize operations and drive innovation.
Listen to the article
Author’s Bio
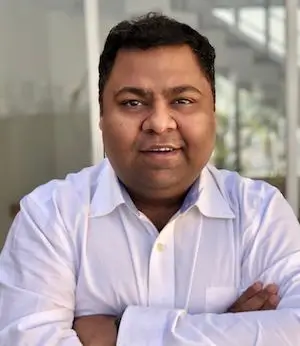
An early adopter of emerging technologies, Akash leads innovation in AI, driving transformative solutions that enhance business operations. With his entrepreneurial spirit, technical acumen and passion for AI, Akash continues to explore new horizons, empowering businesses with solutions that enable seamless automation, intelligent decision-making, and next-generation digital experiences.
Table of content
- What are vertical AI agents?
- Vertical AI vs. horizontal AI vs. SaaS
- The vertical AI opportunity: Why now?
- Why are vertical AI agents important?
- Vertical LLM agents: The next big thing
- Challenges and considerations in vertical AI adoption
- Overcoming the challenges of vertical AI agent implementation with ZBrain
- ZBrain's prebuilt agents: An immediate solution to operational challenges across industries
- Strategic considerations for success with vertical LLM agents
Insights
CUA models
CUA models, or Computer-Using Agent models, represent a significant advancement in the field of artificial intelligence, which are designed to interact with graphical user interfaces like humans.
AI in procure-to-pay processes
AI enhances the procure-to-pay cycle by automating routine tasks, improving decision-making, and ensuring better compliance, resulting in smoother, faster, and more efficient procurement operations.
AI in account-to-report
Artificial intelligence is transforming accounting-to-reporting (A2R) processes by automating repetitive tasks, improving accuracy, and enhancing decision-making.
Generative AI for contracts management
Generative AI in contract management leverages natural language processing to understand and interpret the complex language used in legal documents.
Generative AI in manufacturing
Generative AI is significantly transforming the manufacturing sector by enhancing operational efficiencies, driving innovation, and enabling mass customization.
Generative AI in customer service
As customer service practices evolve rapidly, generative AI is set to profoundly influence how these functions are managed and executed, focusing on enhancing interaction quality and operational efficiency.
Generative AI for regulatory compliance
Generative AI is transforming the field of regulatory compliance, enhancing risk management, operational efficiency, and compliance monitoring.
Generative AI in due diligence
Generative AI is redefining the scope and efficiency of due diligence by automating data-heavy tasks, enhancing analytical precision, and enabling faster, more informed decision-making.
Generative AI in logistics
Generative AI is poised to transform logistics by automating repetitive tasks, improving efficiency, and enhancing decision-making.