AI in account-to-report: Scope, integration, use cases, challenges and future outlook
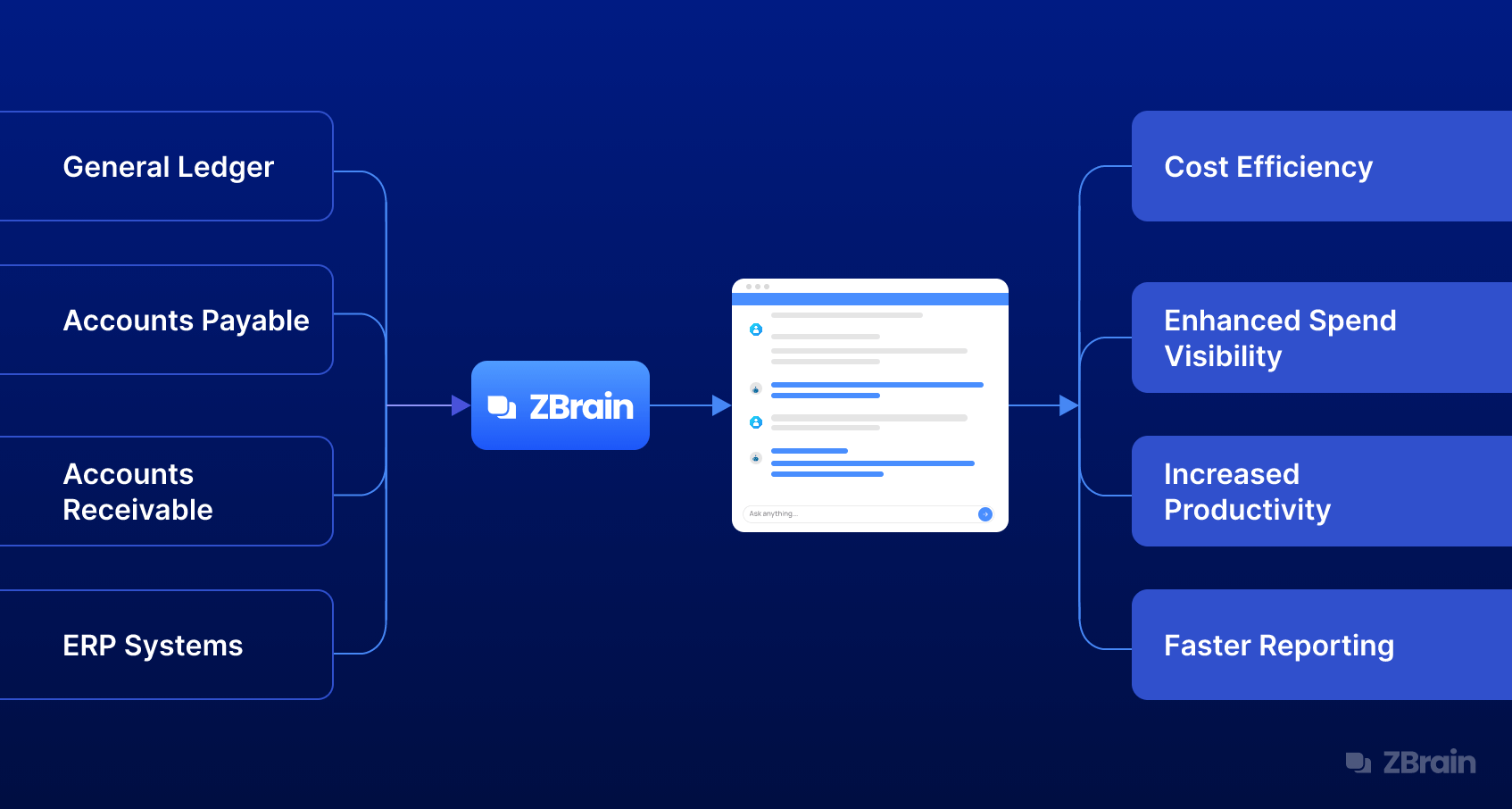
Listen to the article
The account-to-report (A2R) domain, encompassing critical financial operations such as journal entries, general ledger updates, and financial reporting, plays a vital role in ensuring transparency, compliance, and strategic decision-making. However, traditional A2R processes are often hindered by inefficiencies, errors, and long processing times, limiting the accuracy and timeliness of financial data. These challenges are exacerbated by the ever-growing volume of financial transactions and regulatory complexities.
The increasing complexity of financial operations and mounting regulatory requirements have put significant pressure on the A2R process. To stay competitive, organizations must embrace digital transformation, moving away from manual and error-prone tasks. AI offers an efficient way forward, enabling automation, improving accuracy, and accelerating reporting cycles. Recent KPMG studies reveal that 72% of organizations are already piloting or using AI in financial reporting, with widespread adoption expected within 2027. AI’s role in A2R is especially crucial in addressing inefficiencies—automating manual tasks, detecting anomalies, and providing real-time insights into financial performance. In fact, KPMG’s research shows that AI could revolutionize financial reporting by speeding up tasks, enhancing audit accuracy, and reducing fraud.
As AI adoption continues to surge, platforms like ZBrain play a crucial role in helping businesses integrate AI into financial workflows, optimizing their A2R processes effectively. These platforms help finance teams automate tasks, detect anomalies, and generate real-time insights for strategic decision-making. ZBrain goes beyond traditional automation by evaluating an organization’s AI readiness within the account-to-report process. It identifies key opportunities for optimization across financial workflows, from journal entries and ledger maintenance to regulatory reporting and financial consolidation. ZBrain provides customized AI solutions to streamline A2R tasks, with the potential to reduce manual effort and improve reporting cycles. The platform is designed to support finance teams in enhancing efficiencies, improving data accuracy, and ensuring compliance, which can drive smarter decision-making.
This article delves into how AI is transforming the account-to-report process, driving efficiency, accuracy, and compliance. It also discusses how, with platforms like ZBrain, businesses can harness AI to streamline A2R workflows, improve decision-making, and stay competitive in a fast-changing financial landscape.
- What is account-to-report(A2R)?
- Understanding the account-to-report process flow
- Transforming account-to-report: How AI solves traditional challenges
- Approaches to integrating AI into account-to-report
- AI solutions transforming account-to-report processes
- ZBrain AI solutions for account-to-report use cases
- Why ZBrain is the ideal platform for account-to-report
- Benefits of implementing AI in account-to-report (A2R)
- Measuring the ROI of AI in account-to-report
- Challenges and considerations in adopting AI for account-to-report
- Best practices for implementing AI in account-to-report
- The future of AI in account-to-report
- Transform account-to-report operations with ZBrain
What is account-to-report(A2R)?
Account-to-report (A2R) is a critical financial process that governs the management and reporting of accounting data within an organization. It encompasses the entire lifecycle of accounting activities, from capturing financial transactions and maintaining the general ledger to reconciling accounts and preparing trial balances. A2R acts as the backbone of financial reporting, ensuring that data is accurate, reliable, and compliant with regulatory standards.
Key stages of the A2R process include recording transactions, performing account reconciliations, closing accounting periods, and preparing accurate inputs for financial statements. By seamlessly integrating accounting activities, A2R provides organizations with a structured and transparent framework to ensure financial accuracy and operational efficiency.
Although A2R is a process framework rather than a technology, its stages can be significantly enhanced through automation and Enterprise Resource Planning (ERP) systems. Automating tasks such as journal entry creation, reconciliation, and adjustment reduces manual errors, accelerates close cycles, and ensures the timely availability of financial data. This integration empowers organizations to maintain data integrity, improve compliance, and make well-informed business decisions.
By efficiently managing the A2R process, businesses can establish a robust foundation for financial reporting, support strategic decision-making, and adapt to the growing complexities of financial operations.
Why is A2R important?
Account-to-report plays a vital role in financial management by delivering a streamlined and systematic approach to managing accounting and reporting activities. Its structured framework ensures that all financial data is accurately recorded and reconciled, forming the basis for generating reliable financial reports.
One key benefit of A2R is its ability to standardize accounting tasks such as journal entry management, account reconciliations, and period closings. This consistency minimizes discrepancies, accelerates reporting timelines, and enhances overall data accuracy. By providing real-time visibility into accounting activities, A2R enables businesses to detect and rectify errors promptly, reducing the risk of financial misstatements.
A well-executed A2R process also fosters compliance with financial regulations and standards. This ensures that organizations meet legal requirements and maintain transparency in their financial reporting. Additionally, the insights derived from A2R data support better forecasting, budgeting, and strategic planning, allowing businesses to make informed decisions and achieve their financial goals.
Ultimately, A2R strengthens financial governance, improves operational efficiency, and equips organizations to handle complex financial challenges effectively.
Understanding the account-to-report process flow
The account-to-report process is a critical component of financial management, ensuring accurate recording, reporting, and reconciliation of financial data. It encompasses a range of activities designed to support organizational decision-making and maintain compliance with regulatory standards. The process is structured into four key functional areas, each playing a distinct role in the financial ecosystem:
-
Accounting
-
Costing
-
Period closing
-
Reporting and support
Below is a detailed breakdown of these activities:
Accounting (Finance and managerial)
This area involves:
-
Financial planning: Setting financial objectives, creating budgets, and forecasting future financial needs.
-
Journal entry creation: Automating the creation and approval of journal entries to reflect business transactions accurately.
-
General ledger maintenance: Maintaining an accurate General Ledger (GL) to ensure the integrity of financial data.
-
Customer invoice clearing: Managing and reconciling customer invoices for accurate records.
-
Issuing credit notes: Adjusting invoices by issuing credit for overpayments or returns.
-
Vendor invoice clearing (Purchase order-based): Ensuring vendor payments align with purchase orders.
-
Vendor invoice clearing (FI-based invoice): Managing vendor payments directly in the financial system.
-
Intercompany (IC) invoice clearing: Handling intercompany transactions for accurate internal records.
-
Acquisition of retired assets: Processing records related to acquiring assets that were previously retired.
Costing
This area focuses on:
-
Product cost controlling: Monitoring and managing product costs to ensure efficiency.
-
Production controlling: Overseeing costs and budgets related to manufacturing processes.
-
Cost center planning and allocation: Allocating costs across departments or units to track financial performance.
Period closing (Month, Quarter, Fiscal)
Key activities include:
-
Period-end closing: Finalizing financial records at the end of each period (monthly, quarterly, or annually).
-
Trial balance preparation: Generating trial balances to confirm that debits and credits are balanced, serving as the basis for preparing financial statements.
-
Reconciliation of accounts: Verifying account balances and resolving discrepancies to ensure accuracy.
-
Financial consolidation/Reconcile: Combining financial statements from different units or entities and ensuring they are accurate and reconciled.
-
Closing period management: Automating the closing of financial periods, including posting adjustments.
Reporting and support
This area covers:
-
Financial and management accounting reporting: Preparing reports for internal and external stakeholders, including financial statements and management dashboards.
-
Consolidated financial reporting: Aggregating financial data from multiple entities or business units into consolidated financial statements for comprehensive reporting.
-
Regulatory compliance reporting: Ensuring that financial reports meet the required legal and regulatory standards, including tax filings, audits, and industry-specific compliance.
-
Financial statement analysis: Analyzing financial data to identify trends, variances, and insights to guide strategic decision-making.
-
IDOC monitoring: Managing and tracking electronic data exchange (Intermediate Documents) to ensure system transactions are processed correctly.
-
Audit trail and documentation: Maintaining an accurate audit trail of financial transactions to facilitate external audits and internal reviews.
These activities collectively ensure the accuracy, integrity, and timeliness of financial data, allowing organizations to make informed business decisions and comply with regulatory requirements.
Optimize Your Operations With AI Agents
Our AI agents streamline your workflows, unlocking new levels of business efficiency!
Transforming account-to-report: How AI solves traditional challenges
The account-to-report process is essential for accurate financial reporting, regulatory compliance, and strategic decision-making. However, traditional A2R processes often encounter significant challenges, including inefficiencies, manual errors, and difficulties in achieving real-time insights. Integrating Artificial Intelligence (AI) into the A2R cycle can address these pain points, streamline workflows, and improve the overall effectiveness of financial operations. Below is a breakdown of key A2R challenges, their impact, and how AI helps overcome them:
Challenge |
Impact of traditional methods |
How AI helps overcome the challenge |
---|---|---|
Manual journal entries |
Increases errors, delays, and inconsistencies in financial records. |
AI automates journal entry creation, ensuring accuracy, consistency, and speed. |
Reconciliation inefficiencies |
Time-consuming manual reconciliations lead to delays in closing periods. |
AI automates reconciliations, identifies discrepancies, and accelerates period-end closings. |
Lack of real-time data visibility |
Hinders timely decision-making and increases risks of errors in financial reporting. |
AI provides real-time financial data tracking and advanced dashboards for better visibility. |
Error-prone consolidation |
Manual consolidation processes lead to inconsistencies and reporting delays. |
AI automates consolidation, ensuring faster, error-free, and consistent reporting. |
Approval bottlenecks |
Delays in approval workflows slow down journal postings and adjustments. |
AI streamlines approval workflows by automating routing and prioritizing tasks. |
Compliance risks |
Increased risk of non-compliance with accounting standards and regulations. |
AI monitors compliance rules, flags potential violations, and ensures adherence to standards. |
Fraud and anomaly detection |
Difficulty detecting fraudulent transactions in large data volumes. |
AI detects anomalies and patterns indicative of fraud, reducing financial risks. |
Period closing delays |
Manual processes cause delays in completing monthly, quarterly, and annual closings. |
AI automates closing tasks and ensures all entries are posted on time. |
Limited financial insights |
Lack of actionable insights hampers strategic planning and decision-making. |
AI provides advanced analytics, trend identification, and actionable recommendations for better decisions. |
Audit trail and documentation gaps |
Inefficient documentation increases audit preparation time and the risk of audit failures. |
AI creates a complete, automated audit trail for faster and more accurate reviews. |
Approaches to integrating AI into account-to-report
AI is transforming the account-to-report process by automating tasks, improving accuracy, and delivering valuable insights for better decision-making. Organizations seeking to integrate AI into their A2R workflows can choose from several approaches, depending on their specific requirements, resources, and long-term goals. Below is an overview of the primary strategies for integrating AI into A2R.
Custom, in-house AI development
This approach involves developing a tailored AI solution to address specific challenges in the A2R processes, such as journal entry automation, account reconciliation, and financial consolidation. It requires building or fine-tuning AI models to align with a company’s unique processes and data.
Advantages:
-
Customization: Offers flexibility to address specific A2R pain points, such as tailored compliance checks or anomaly detection in financial data.
-
Full control: Enables control over data privacy, model training, and compliance with internal and regulatory standards.
-
Long-term fit: Ensures the solution evolves with the organization, adapting to future needs and scaling with growth.
Using AI point solutions
This approach leverages pre-built, off-the-shelf AI tools designed to tackle specific tasks in the A2R process, such as automated reconciliations, variance analysis, or financial statement preparation.
Advantages:
-
Quick deployment: Point solutions are ready to deploy, offering immediate improvements in areas like account reconciliation or journal entry processing.
-
Cost-effective: These tools typically require fewer resources to implement compared to custom solutions.
-
Ease of use: Designed for non-technical users, these tools are often easy to integrate into existing financial systems and workflows.
Adopting a comprehensive AI platform
A comprehensive AI platform provides an integrated environment with multiple AI capabilities to address end-to-end A2R processes. Comprehensive AI enablement platforms like ZBrain often combine AI models, data management capabilities, and application-building frameworks to automate and optimize processes like period closings, financial reporting, and compliance monitoring.
Advantages:
-
Centralized data and governance: Ensures consistent data management and compliance with regulatory standards while maintaining data security.
-
End-to-end optimization: Supports automation and optimization across the entire A2R lifecycle, from journal entries to financial reporting and audit preparation.
-
Scalability and flexibility: Easily scalable to meet growing reporting demands and adaptable to future AI advancements.
-
Efficiency: Reduces manual intervention by automating repetitive tasks, increasing speed, and enhancing accuracy.
Choosing the right approach
Selecting the best AI integration strategy for your A2R processes involves considering several key factors:
-
Specific business needs: Identify which aspects of the A2R cycle require AI intervention, such as account reconciliation, journal entry creation, or compliance monitoring.
-
Resources and expertise: Evaluate internal expertise, budget availability, and technical infrastructure to support AI implementation.
-
Compliance and security requirements: Ensure the chosen AI solution meets industry regulations and aligns with data security standards.
-
Scalability and long-term goals: Choose a strategy that supports future growth and aligns with broader organizational objectives.
By aligning the integration approach with organizational priorities, businesses can unlock the full potential of AI to enhance efficiency, compliance, and financial insights within the A2R process.
AI solutions transforming account-to-report processes
Artificial intelligence is transforming A2R processes by automating repetitive tasks, improving accuracy, and enhancing decision-making. AI solutions optimize financial operations, from journal entry automation to period-end closing and real-time reporting. Below is a detailed explanation of AI applications across each core A2R process and the associated sub-processes, highlighting how AI contributes to increased efficiency, accuracy, and compliance at every stage of the workflow.
Journal entry automation
Automating journal entries helps streamline the accounting process, improving efficiency and reducing human error.
-
Automated journal entry creation: AI automates the creation of journal entries, ensuring accuracy and reducing manual errors in transaction recording.
-
Approval workflow: AI-based approval systems facilitate real-time reviews and validations of journal entries.
-
Error detection: AI identifies inconsistencies or anomalies in journal entries, ensuring data integrity.
General ledger maintenance
The general ledger is central to financial reporting, and maintaining its accuracy is crucial for timely and precise financial statements.
-
Real-time updates: AI ensures the general ledger is continuously updated with the most accurate and current data.
-
Automated reconciliation: AI reconciles accounts automatically, identifying discrepancies and reducing manual oversight.
-
Intelligent classification: AI uses machine learning to classify transactions correctly, improving efficiency and reducing errors.
Customer and vendor invoice management
Invoice management involves automating the matching, reconciliation, and payment processes for both customer and vendor invoices.
-
Invoice matching (PO-based and FI-based): AI automates the process of matching invoices with purchase orders or financial entries, ensuring accuracy.
-
Customer payment reconciliation: AI reconciles customer invoices against payments, speeding up the payment process.
-
Vendor payment reconciliation: AI ensures accurate processing of vendor invoices by automatically matching them to corresponding purchase orders.
Costing and cost control
Costing and cost control are critical for ensuring profitability and efficient resource allocation within a business.
-
Product costing: AI tracks and analyzes product cost data, forecasting potential cost overruns and suggesting cost-saving measures.
-
Production cost management: AI optimizes production costs by analyzing production data in real time and recommending adjustments to processes.
-
Cost center allocation: AI automates cost allocations across different business units, improving transparency and financial oversight.
Period-end closing
Period-end closing is a complex process that can benefit from AI automation to reduce manual effort and ensure timely financial reporting.
-
Automated period closing: AI automates tasks like journal entry posting, account reconciliation, and generating closing reports for faster and more accurate period-end closure.
-
Trial balance preparation: AI prepares trial balances by automatically posting entries and ensuring that debits and credits are balanced.
-
Financial consolidation: AI consolidates financial data from various subsidiaries or business units to provide a unified and accurate financial statement.
Financial reporting
Financial reporting involves generating key statements that provide insights into an organization’s financial performance and compliance.
-
Automated report generation: AI automatically generates financial reports such as income statements and balance sheets, providing real-time insights into financial health.
-
Management dashboards: AI-driven dashboards display key financial metrics and trends, enabling data-driven decision-making with real-time access to financial data.
-
Regulatory compliance reporting: AI ensures reports are compliant with both local and international regulations, reducing the risk of compliance errors.
Audit trail and compliance
Maintaining an audit trail and ensuring compliance with regulatory requirements is essential for financial transparency and accountability.
-
Transaction monitoring: AI tracks financial transactions in real time, ensuring that every movement is accurately recorded and easily retrievable for audits.
-
Audit trail generation: AI automatically creates an immutable audit trail, providing clear documentation of all financial transactions for regulatory review.
-
Compliance reporting: AI automates the generation of regulatory compliance reports, ensuring that all financial activities meet legal and industry standards.
These AI applications improve efficiency, reduce human error, and strengthen compliance across the A2R process, ultimately supporting better financial decision-making and performance tracking.
Credit management
Credit management focuses on assessing customer creditworthiness and managing credit limits to minimize financial risk.
-
Credit risk assessment: AI can analyze historical data and external sources to assess the creditworthiness of customers and manage credit limits.
-
Predictive credit modeling: AI could predict customer payment behavior based on past transactions, industry trends, and external financial factors.
-
Automation of credit approvals: AI can automate the credit approval process by verifying customer data and determining appropriate terms.
Tax accounting
Tax accounting involves the accurate calculation, reporting, and compliance of tax liabilities in accordance with local and international regulations.
-
Tax compliance automation: AI can automate the calculation of tax liabilities, ensuring compliance with local and international tax laws.
-
Tax reporting: AI can automatically generate tax reports, file documents, and ensure correct deductions and credits.
-
Tax compliance validation: AI can validate tax on transactions, ensuring accurate tax reporting and compliance within the Account-to-Report process.
Asset accounting
Asset accounting tracks the acquisition, depreciation, and disposal of fixed assets, ensuring accurate financial reporting and asset management.
-
Depreciation calculation: AI can calculate asset depreciation automatically, adjusting for changes in usage or market value.
-
Asset lifecycle management: AI could track assets through their lifecycle, from acquisition to disposal, providing insights on asset utilization and potential write-offs.
-
Asset reconciliation: AI can automate the reconciliation of physical assets with financial records to ensure accuracy.
Treasury – bank accounting
Treasury – bank accounting manages cash flow and reconciles bank statements to ensure accurate financial records and liquidity.
-
Bank reconciliation: AI can automate the reconciliation of bank statements with internal records, identifying discrepancies and reducing manual oversight.
-
Cash flow forecasting: AI can provide real-time cash flow forecasts by analyzing historical transaction data and predicting future liquidity needs.
-
Bank statement automation: AI can automatically process incoming bank data, match transactions to the appropriate accounts, and update the financial system.
Treasury – financial instruments
Treasury – financial instruments involve the valuation and management of various financial assets and liabilities to mitigate risk and optimize returns.
-
Financial instruments valuation: AI can help automatically value financial instruments such as stocks, bonds, and derivatives based on market data.
-
Portfolio risk management: AI can assess the risk exposure of financial portfolios and suggest adjustments based on predictive analytics.
-
Trading automation: AI can assist in executing trades based on pre-set strategies and real-time market conditions.
Treasury – risk management
Treasury – risk management focuses on identifying, assessing, and mitigating financial risks that may impact the organization’s stability.
-
Risk identification and monitoring: AI can analyze data to identify potential financial risks, including credit, liquidity, and market risks.
-
Predictive analytics: AI can use historical data to predict financial risks and provide strategies to mitigate them.
Treasury – cash and liquidity management
Treasury – cash and liquidity management ensures that an organization has sufficient cash flow to meet its obligations while optimizing cash reserves.
-
Liquidity forecasting: AI can predict future liquidity needs based on transaction data, market conditions, and historical cash flows.
-
Cash position management: AI can optimize cash positioning by analyzing real-time balances and forecasting cash requirements.
-
Automated cash transfers: AI can suggest or automate internal cash transfers to optimize cash reserves across business units.
Intercompany
Intercompany processes involve managing transactions and reconciliations between different business units or subsidiaries within the same organization.
-
Intercompany reconciliation: AI can automate the reconciliation of intercompany transactions, ensuring accurate and timely reporting.
-
Cross-border transaction processing: AI could streamline the processing of intercompany transactions across multiple jurisdictions, factoring in currency conversions and tax regulations.
-
Intercompany settlement: AI could assist in managing and automating the settlement of intercompany balances, ensuring accurate financial reporting.
Financial planning and budgeting
Financial planning and budgeting encompass the forecasting and allocation of financial resources to align with the organization’s strategic goals.
-
Budget forecasting: AI can automate the creation of budgets based on historical data, future trends, and predictive analytics.
-
Scenario planning: AI can model various financial scenarios to assist in planning for different business conditions.
-
Predictive budget monitoring: AI can track actual performance against budgeted figures, offering early alerts for potential budget overruns.
Project accounting
Project accounting tracks the financial performance of specific projects, ensuring that costs are managed and revenue is recognized appropriately.
-
Project cost tracking: AI can track project-related expenses and compare them to budgets, helping to detect potential overruns early.
-
Automated billing: AI can generate invoices for project milestones or time-based work, streamlining revenue recognition.
-
Resource allocation optimization: AI could optimize the allocation of resources based on project timelines, costs, and priority, reducing inefficiencies.
ZBrain AI solutions for account-to-report use cases
ZBrain is an end-to-end AI enablement platform that can help streamline and optimize A2R processes. By leveraging its components—ZBrain XPLR for AI readiness assessment and ZBrain Builder for designing and deploying customized solutions— ZBrain helps organizations address A2R challenges effectively. Below is a breakdown of key A2R use cases and how ZBrain can enhance each with AI-powered solutions:
A2R use case |
Description |
How ZBrain helps |
---|---|---|
Automated journal entries |
Automating creation, approval, and validation of journal entries to reduce manual effort. |
ZBrain Builder can help build agent which can automate journal entry creation, detect errors, and ensure validation for accuracy. |
Real-time ledger updates |
Ensuring the general ledger reflects the most current and accurate data. |
ZBrain AI solutions can automatically update ledgers, reconcile accounts, and maintain financial data integrity. |
Invoice matching |
Matching customer/vendor invoices with POs or financial records to prevent discrepancies. |
ZBrain’s Purchase-Order-Invoice Matching agent automates matching purchase orders and invoices for accuracy in quantities, prices, and delivery terms, ensuring timely and accurate payment approvals. |
Credit and debit note processing |
Managing adjustments in invoices for overpayments or returns. |
ZBrain’s Dispute Resolution AI Agent analyzes contracts, delivery records, and shipping information to resolve disputes and automate the issuance of debit notes quickly. |
Intercompany invoice reconciliation |
Handling transactions between internal entities to ensure accurate financial reporting. |
ZBrain Builder can help create AI agent that can automate intercompany reconciliation, maintaining balance and compliance across subsidiaries. |
Cost center allocations |
Allocating costs across departments or business units for accurate financial analysis. |
ZBrain AI solutions can automate allocations, enhance visibility, and track cost performance. |
Product costing optimization |
Tracking product costs to identify overruns and improve cost efficiency. |
ZBrain AI solutions can analyze historical data and recommend cost-saving measures for product development. |
Period-end closing |
Completing financial activities at the end of each reporting period. |
ZBrain AI solutions can accelerate period-end activities such as account reconciliation and trial balance preparation. |
Financial data consolidation |
Combining data from various units for unified reporting. |
ZBrain AI solutions can automate data aggregation and ensure consistency in consolidated financial statements. |
Regulatory compliance reporting |
Generates reports adhering to local and international standards to mitigate risks. |
ZBrain’s Regulatory Compliance Monitoring Agent automates the validation of financial reports against local and international standards. It is designed to reduce compliance risks, ensure timely submission, and minimize errors in the reporting process by streamlining compliance checks. |
Audit trail generation |
Tracking all financial transactions to maintain transparency and facilitate audits. |
ZBrain AI solutions can create detailed, immutable audit trails for easier regulatory reviews and internal tracking. |
Tax filing and compliance |
Preparing and validating tax filings in adherence to regulations. |
ZBrain’s Corporate Tax Review Agent automates corporate tax reviews, ensuring accurate calculations and filings and reducing compliance risks. |
Real-time financial analysis |
Providing insights into profitability, liquidity, and operational efficiency. |
ZBrain AI solutions can deliver real-time analytics, allowing businesses to adjust strategies dynamically. |
ZBrain’s AI-powered solutions are designed to help organizations automate complex A2R workflows, improve data integrity, and provide actionable insights, potentially driving better financial performance and strategic decision-making.
Optimize Your Operations With AI Agents
Our AI agents streamline your workflows, unlocking new levels of business efficiency!
Why ZBrain is the ideal platform for account-to-report
ZBrain, with its AI capabilities, can help organizations optimize their account-to-report processes. It provides a range of features aimed at improving automation, increasing efficiency, and supporting informed decision-making.
-
AI readiness assessment: ZBrain’s AI readiness assessment framework, ZBrain XPLR, can evaluate an organization’s current capabilities and preparedness for AI adoption in A2R processes. It can provide actionable insights to help organizations understand their strengths and areas for improvement, ensuring a smooth and successful AI implementation.
-
Low-code development: ZBrain’s low-code platform ZBrain Builder simplifies the creation of custom AI solutions to address unique A2R challenges, making it accessible to business users without extensive technical expertise.
-
Proprietary data utilization: The platform enables organizations to leverage their proprietary data effectively, ensuring AI solutions are tailored to the specific needs and goals of their A2R operations.
-
Enterprise-ready: ZBrain is designed for enterprise environments, offering features such as security, scalability, and integration with existing A2R systems, which can benefit large organizations.
-
End-to-end support: ZBrain manages the end-to-end processes of A2R AI applications—from initial development to deployment and ongoing support—ensuring continuous optimization and smooth transitions.
-
Flexible data ingestion: ZBrain integrates data from multiple sources to support A2R processes with real-time financial information, potentially improving decision-making, financial reporting, and operational efficiency.
-
Intelligent agent creation: AI agents built on Zbrain Builder can assist in automating critical A2R tasks such as journal entries, ledger reconciliation, and financial reporting, potentially reducing manual effort and enhancing operational efficiency.
These capabilities position ZBrain as a tool that may assist organizations in optimizing and automating their A2R processes, potentially improving efficiency, accuracy, and scalability in financial operations.
Benefits of implementing AI in account-to-report (A2R)
Integrating AI into A2R processes offers transformative benefits for organizations, employees, and other stakeholders. Here is a breakdown of how AI enhances A2R:
For organizations:
-
Cost efficiency: Automation reduces manual effort, saving costs on repetitive tasks like journal entries and reconciliations.
-
Enhanced decision-making: Real-time, decision-ready data provides actionable insights for better financial management.
-
Improved compliance: AI helps ensure regulatory compliance by automating validation and reporting processes.
-
Data integration: Seamless integration with existing ERPs ensures up-to-date, accurate data flows, reducing errors and inefficiencies.
-
Operational efficiency: AI optimizes financial operations, improving speed and accuracy in tasks like period-end closing and financial reporting.
For employees:
-
Increased productivity: Routine tasks like data entry and reconciliation are automated, enabling employees to focus on strategic tasks.
-
Skill development: Employees have opportunities to learn and grow by managing more complex AI-driven tasks and data analysis.
-
Job satisfaction: Automation of monotonous tasks improves employee morale and job satisfaction, allowing staff to engage in more meaningful work.
For customers:
-
Faster reporting: With AI handling key A2R processes, businesses can provide quicker financial reporting and insights.
-
Enhanced transparency: Automated reporting and compliance monitoring provide customers with clear, reliable financial information.
-
Improved service delivery: The efficiency of A2R processes ensures timely financial transactions, improving customer relations.
By implementing AI in A2R, organizations can achieve long-term cost savings, improved financial accuracy, and strategic decision-making, contributing to overall business growth.
Measuring the ROI of AI in account-to-report
Implementing AI in accounting-to-reporting processes provides substantial returns by enhancing accuracy, efficiency, and data-driven decision-making. ZBrain’s AI solutions support key financial operations, from automating journal entries to aiding financial reporting and compliance monitoring. Businesses may assess the impact of these solutions by evaluating factors such as cost reduction, process efficiency, and financial control to determine the value of their AI investments. Below are examples of how ZBrain’s applications optimize A2R workflows, delivering measurable business benefits and a clear ROI.
ZBrain implementation in A2R processes: Key ROI indicators
AI implementation in A2R processes using ZBrain can drive ROI by improving efficiency, reducing errors, and enhancing decision-making. Here’s a breakdown of ROI for key A2R use cases:
-
Automated journal entries
-
Use case: Automating the creation and approval of journal entries for accurate financial records.
ROI metrics:-
Reduced manual processing
-
Faster approvals
-
Improved accuracy
-
Example: ZBrain AI agents can automate journal entry creation, ensuring data integrity and speeding up financial reporting with fewer errors.
-
General ledger maintenance
-
Use case: Automating the reconciliation and real-time updating of the general ledger.
ROI metrics:-
Lower reconciliation costs
-
Fewer errors
-
Example: ZBrain AI agents can ensure continuous updates and support reconciliations, enhancing the accuracy of the general ledger.
-
Cost center allocation
-
Use case: Automating cost allocation across different cost centers for efficient financial management.
ROI metrics:-
Efficient cost distribution
-
Improved transparency
-
Example: ZBrain AI agents can automate cost center allocations, streamlining financial reporting and improving visibility.
-
Financial reporting
-
Use case: Automating financial report generation for faster and more accurate reporting.
ROI metrics:-
Faster report generation
-
Enhanced compliance
-
Real-time insights
-
Example: ZBrain AI agents can generate real-time financial reports, ensuring compliance and providing timely insights for better decision-making.
-
Regulatory compliance reporting
-
Use case: Automating compliance checks to ensure financial reports meet regulatory standards.
ROI metrics:-
Improved accuracy
-
Reduced compliance risks
-
Example: ZBrain AI agents can automate the validation of reports, ensuring adherence to local and international standards, minimizing errors, and reducing compliance risks.
These examples highlight the significant impact of AI in transforming A2R processes by streamlining tasks, improving financial accuracy, and reducing operational costs. Organizations can validate the effectiveness of their AI investments by measuring key ROI metrics such as improved efficiency, reduced errors, and enhanced compliance. ZBrain offers tools designed to support continuous optimization, real-time insights, and automation, which may assist finance teams in focusing on strategic decision-making and improving A2R processes.
Challenges and considerations in adopting AI for account-to-report
While the integration of AI in account-to-report processes can bring transformative benefits, organizations must address various challenges to ensure successful adoption. These challenges include overcoming resistance to change, ensuring data quality and security, managing integration with legacy systems, and addressing regulatory compliance concerns. Additionally, companies must consider the costs of implementation, the need for employee upskilling, and the potential complexities in managing AI-driven decision-making processes. By understanding these considerations, businesses can better navigate the path to effective AI adoption in A2R.
Aspect |
Challenge |
How ZBrain addresses these challenges |
---|---|---|
Data integration |
Integrating data from various systems (ERP, external databases, spreadsheets) can be challenging due to different formats and technologies. |
ZBrain Builder can integrate data from multiple systems and formats, which may help facilitate smoother integration across diverse platforms. |
Legacy system compatibility |
Legacy systems may not be compatible with AI tools, requiring an AI maturity evaluation to assess readiness for AI adoption. |
ZBrain XPLR provides an AI readiness assessment to evaluate your systems’ ability to integrate with AI, ensuring that your infrastructure can support AI initiatives before full implementation. |
High initial investment |
Initial costs for AI implementation, including software, system upgrades, and training, can be prohibitive, especially for smaller organizations. |
The ZBrain team can help assess the feasibility and ROI of AI adoption, enabling a phased implementation approach. This can potentially reduce initial costs and ensure strategic planning for a smooth roll-out. |
Ongoing maintenance costs |
Regular updates and monitoring of AI systems can result in unforeseen costs. |
ZBrain ensures ongoing monitoring and updation, minimizing manual intervention and reducing maintenance costs. |
Data security risks |
AI systems handling sensitive financial data may expose businesses to data breaches or unauthorized access. |
ZBrain implements access control mechanisms, such as role-based access, along with compliance checks, to help mitigate data security risks. |
Data privacy compliance |
Adhering to regional and global data privacy regulations for cross-border financial data can be complex. |
ZBrain ensures compliance with frameworks like ISO 27001:2022 and SOC 2 Type II, adapting to regulatory changes with AI-driven automation for data security and privacy. |
Lack of skilled personnel |
Specialized expertise in machine learning and AI implementation may be lacking, delaying AI deployment. |
ZBrain Builder’s low-code platform facilitates seamless development and deployment of AI solutions, potentially reducing the need for advanced AI expertise. |
Training and adoption |
Employees may resist AI adoption due to unfamiliarity with the tools or fear of job displacement. |
ZBrain Builder’s user-friendly interface with high customizability eases the transition and fosters adoption. |
Inaccurate or incomplete data |
Poor or inconsistent data quality can lead to flawed AI predictions and inaccurate decision-making. |
ZBrain Builder’s preprocessing and data normalization features may help improve data accuracy and completeness, potentially reducing errors before feeding data into AI models. |
Scalability issues |
Expanding AI tools to different business units or regions can be challenging, especially if scalability wasn’t considered in the original implementation. |
ZBrain’s cloud-native architecture and modular design may support scalability, potentially enabling gradual expansion with fewer additional resources. |
Optimize Your Operations With AI Agents
Our AI agents streamline your workflows, unlocking new levels of business efficiency!
Best practices for implementing AI in account-to-report
Implementing AI in the account-to-report (A2R) processes can enhance financial accuracy, streamline workflows, and improve decision-making. However, a successful implementation requires thorough planning and strategic execution. Below are key best practices for implementing AI in A2R processes:
1. Assess process readiness for AI integration
Before adopting AI, evaluate the current state of your A2R workflows to identify optimization opportunities.
-
Map existing workflows: Conduct process discovery to understand bottlenecks and areas for automation, such as journal entries and reconciliation.
-
Assess data quality and infrastructure: Ensure access to structured, clean data and a robust infrastructure capable of supporting AI tools.
-
Gauge change readiness: Involve stakeholders to understand concerns and align expectations.
-
Define clear goals: Establish measurable objectives like improved financial reporting accuracy or reduced reconciliation time.
2. Leverage the right AI technologies
Selecting appropriate AI technologies ensures maximum impact on A2R processes.
-
Machine learning for anomaly detection: Identify irregularities in financial transactions and predict trends, ensuring compliance and reducing risks.
-
NLP for document processing: Automate data extraction from financial documents, such as contracts and invoices, improving accuracy and efficiency.
-
Dashboards for insights: Use AI-driven dashboards for real-time financial performance tracking and compliance reporting.
3. Engage stakeholders and manage change effectively
AI implementation success depends on stakeholder engagement and change management.
-
Communicate the strategy: Highlight how AI simplifies roles and improves productivity while addressing concerns about job displacement.
-
Provide training and upskilling: Equip teams with knowledge and skills for leveraging AI tools effectively.
-
Involve stakeholders early: Secure buy-in from finance, compliance, and IT teams to ensure smooth integration.
-
Pilot and phased rollouts: Start with small-scale implementation, gradually expanding as teams adapt.
4. Ensure scalability and flexibility
AI solutions should be designed to adapt to evolving business needs and grow with the organization.
-
Scalability: Implement AI systems that accommodate increased transaction volumes and more complex reporting requirements.
-
Continuous improvement: Regularly evaluate AI systems, updating algorithms and processes based on new data and business objectives.
-
Interoperability: Choose solutions that integrate seamlessly with ERP systems for unified workflows across the A2R process.
By following these best practices, organizations can harness AI to optimize A2R processes, improve financial accuracy, and support strategic decision-making, ensuring sustained value creation and adaptability in a dynamic business environment.
The future of AI in account-to-report
The integration of AI into the account-to-report (A2R) process promises a transformative future marked by improved efficiency, accuracy, and innovation. Key advancements shaping this future include:
1. AI and blockchain integration
The synergy of AI and blockchain technology could revolutionize financial data management in A2R.
-
Data integrity and security: Blockchain ensures tamper-proof records, while AI processes them in real time to minimize errors and fraud.
-
Streamlined reconciliation: AI accelerates transaction validation, enabling faster financial close processes.
-
Cost-effective compliance: Automated ledger audits reduce manual efforts and ensure regulatory adherence.
2. Advanced financial consolidation
AI simplifies the complexities of multi-entity reporting and consolidation for global organizations.
-
Automated data harmonization: AI integrates financial data across systems, minimizing manual errors.
-
Global adaptability: Advanced models manage multi-currency transactions and align with diverse tax laws and accounting standards.
-
Faster closing cycles: AI-driven automation reduces financial closing times, offering real-time insights.
3. NLP for dynamic financial reporting
Natural language processing (NLP) will shift A2R reporting from static to real-time, actionable insights.
-
Automated report generation: NLP extracts key data from documents, enabling accurate and timely reporting.
-
Sentiment analysis: AI assesses financial sentiment from news or market trends to anticipate risks.
-
Compliance scanning: NLP ensures regulatory alignment by validating documentation against compliance requirements.
4. AI-driven financial forecasting
AI leverages real-time data to transform financial forecasting, making it more precise and relevant.
-
Real-time adaptability: Forecasts dynamically adjust using live inputs, improving prediction accuracy.
-
Scenario analysis: AI simulates multiple outcomes to guide proactive risk management and strategic planning.
-
Strategic insights: AI uncovers trends to support decisions on cash flow, revenue, and costs.
5. Ethical AI in financial reporting
As AI adoption grows, maintaining ethical standards will be critical.
-
Transparent decision-making: AI models will provide explainable outputs, building stakeholder trust.
-
Bias mitigation: Organizations will ensure algorithms are free from biases, supported by diverse training data and audits.
-
Data privacy: Advanced security measures will protect sensitive financial information, ensuring compliance with regulations like GDPR.
AI in A2R is set to redefine financial operations, providing organizations with tools to improve transparency, accuracy, and efficiency while fostering responsible innovation.
Transform account-to-report operations with ZBrain
ZBrain aims to enhance account-to-report (A2R) operations by identifying potential automation opportunities and streamlining workflows. It can support businesses in optimizing their A2R processes with AI solutions designed to improve workflow efficiency, reporting accuracy, and insights, from data collection to financial reporting.
ZBrain XPLR can empower businesses by assessing their AI readiness, preparing them for successful AI integration. The comprehensive assessment uncovers areas for improvement and helps align AI strategies with business objectives, ensuring a smooth transition to AI-driven solutions and minimizing potential risks.
ZBrain Builder’s intuitive, low-code interface, enables users to create custom A2R solutions to automate various financial tasks.
By integrating seamlessly with existing systems, offering scalable performance, and ensuring security, ZBrain helps organizations transform their A2R operations, improve efficiency, and stay competitive in today’s rapidly evolving business environment.
Endnote
The integration of AI into account-to-report (A2R) processes is transforming financial operations by automating key tasks and delivering actionable insights that enhance accuracy and decision-making. AI reduces manual effort, improves compliance, and enables faster financial closes, aligning with strategic goals. As AI technology advances, its ability to optimize A2R processes will expand, helping organizations remain competitive, agile, and prepared for evolving financial landscapes. Embracing AI-driven solutions allows businesses to stay ahead, ensuring improved efficiency and sustained innovation in their financial operations.
Ready to elevate your account-to-report processes with AI? Unlock the power of ZBrain’s intelligent automation for streamlined workflows, improved compliance, and actionable financial insights.
Listen to the article
Author’s Bio
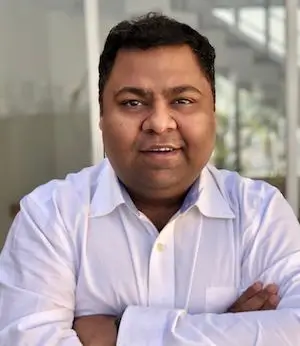
An early adopter of emerging technologies, Akash leads innovation in AI, driving transformative solutions that enhance business operations. With his entrepreneurial spirit, technical acumen and passion for AI, Akash continues to explore new horizons, empowering businesses with solutions that enable seamless automation, intelligent decision-making, and next-generation digital experiences.
Table of content
- What is account-to-report(A2R)?
- Understanding the account-to-report process flow
- Transforming account-to-report: How AI solves traditional challenges
- Approaches to integrating AI into account-to-report
- AI solutions transforming account-to-report processes
- ZBrain AI solutions for account-to-report use cases
- Why ZBrain is the ideal platform for account-to-report
- Benefits of implementing AI in account-to-report (A2R)
- Measuring the ROI of AI in account-to-report
- Challenges and considerations in adopting AI for account-to-report
- Best practices for implementing AI in account-to-report
- The future of AI in account-to-report
- Transform account-to-report operations with ZBrain
Frequently Asked Questions
What is ZBrain, and how can it optimize the account-to-report (A2R) process with AI?
ZBrain is an end-to-end AI enablement platform designed to streamline the AI readiness assessment, use case identification, development and deployment of AI solutions. From data integration and model selection to solution development, deployment and continuous optimization, ZBrain provides end-to-end support for AI implementation across business functions, including A2R.
Here’s how ZBrain enhances A2R processes:
-
AI readiness assessment with ZBrain XPLR: ZBrain XPLR provides a comprehensive AI readiness assessment, helping organizations evaluate their current state and identify key opportunities for AI adoption in A2R processes. ZBrain XPLR guides businesses toward informed, strategic AI adoption for enhanced financial operations by assessing AI maturity and highlighting automation possibilities.
-
Seamless data ingestion and integration: ZBrain Builder connects with various financial systems, general ledger tools, and reporting platforms, enabling the efficient ingestion of structured and unstructured data. This ensures the creation of a unified data pipeline for accurate, real-time financial reporting.
-
Low-code development environment: ZBrain Builder’s intuitive, low-code interface helps accounting and finance teams to create AI agents with minimal programming knowledge, significantly reducing development cycles and accelerating the deployment of AI tools to improve financial workflows.
-
Cloud and model flexibility: ZBrain supports various AI models such as GPT-4 and LLaMA and integrates seamlessly with cloud environments like AWS, Azure, and GCP, providing flexibility in choosing the optimal infrastructure to balance cost, performance, and scalability for A2R processes.
-
Enhanced compliance and governance: With ZBrain’s AI-powered capabilities, organizations can track and ensure compliance with regulatory standards and internal policies, flagging potential risks during financial reporting and closing processes. This ensures continuous audit readiness and strengthens financial governance.
By offering a flexible, low-code platform and robust data integration with custom AI capabilities, ZBrain enables organizations to automate, optimize, and innovate across their entire account-to-report process, transforming the way finance teams manage their financial data and reporting requirements.
How does ZBrain ensure the security and privacy of sensitive data in account-to-report (A2R) processes?
ZBrain is designed with a strong emphasis on data privacy and security, ensuring that sensitive information involved in A2R operations is safeguarded at all stages. Here’s how ZBrain protects sensitive A2R data:
-
Private cloud deployments: ZBrain agents can be deployed in a private cloud environment, ensuring that critical financial data is stored securely within the organization’s infrastructure.
-
Robust security features: ZBrain incorporates multiple layers of security to protect sensitive data, including:
-
Access controls: Granular role-based access controls ensure that only authorized personnel can view or manage sensitive A2R information.
-
Compliance and governance: ZBrain is built to adhere to industry-specific regulations and standards, ISO 27001:2022 and SOC 2 Type II, ensuring that data is handled in a manner that meets compliance requirements for confidentiality, integrity, and accountability.
-
Can ZBrain agents be integrated with existing account-to-report (A2R) systems?
Yes, ZBrain agents are designed to integrate seamlessly with existing A2R systems. The platform supports various data formats and standards, ensuring smooth interoperability with legacy financial, ERP, and reporting systems.
This integration allows organizations to:
-
Leverage existing infrastructure: Enhance current A2R processes without needing a full overhaul of legacy systems.
-
Enrich data and workflows: Connect ZBrain agents with existing tools to automate workflows and improve data accessibility.
-
Drive AI-driven insights: Utilize AI capabilities to optimize financial reporting and enhance decision-making while maintaining compatibility with existing technologies.
By enabling seamless integration, ZBrain ensures that organizations can modernize their A2R processes without disrupting existing systems.
What kind of A2R agents can be built on ZBrain Builder?
ZBrain Builder enables the development of AI agents tailored to diverse business functions, including key account-to-report processes. These agents support in financial consolidation, streamlining financial closing cycles, improving reconciliation tasks, and enhancing reporting accuracy. ZBrain’s advanced AI capabilities help organizations optimize data integration, automate manual tasks, and drive AI-based insights for decision-making.
How does ZBrain cater to diverse A2R needs across finance operations?
ZBrain’s flexibility allows it to address a variety of account-to-report (A2R) needs. You can create tailored AI agents to streamline financial consolidation, automate journal entries, improve period-end closing, and enhance regulatory compliance reporting. Its efficient AI agents enable businesses to improve efficiency, accuracy, and compliance in their A2R processes across any industry.
How can we measure the ROI of ZBrain in our account-to-report (A2R) processes?
Measuring ROI from ZBrain in A2R involves evaluating key performance indicators (KPIs) tied to automation, accuracy, and decision-making efficiency. Here are some important metrics to consider:
-
Reduced manual processing: Automating tasks like journal entry creation and general ledger reconciliation can lead to faster approvals, fewer errors, and improved accuracy.
-
Faster reporting: Automating financial report generation and cost center allocation can enhance reporting speed and transparency.
-
Improved compliance: Automated compliance checks can reduce the risk of errors and enhance adherence to regulations.
-
Operational efficiency: Real-time data updates and automated reconciliations can lower operational costs and improve overall financial management.
By monitoring these KPIs, businesses can quantify how ZBrain drives efficiency, reduces errors, and ensures timely, compliant financial reporting.
How can I get started with ZBrain for my account-to-report (A2R) processes?
To begin using ZBrain for optimizing your A2R processes, simply reach out to us at hello@zbrain.ai or fill out the inquiry form on our website. Our team will get in touch with you to explore how our platform can integrate with your financial systems and streamline your account-to-report workflows.
Insights
AI in contract management
The integration of AI into contract management within the finance domain is transforming financial operations by automating key tasks and providing actionable insights that enhance accuracy and decision-making.
AI in order management
Artificial intelligence is transforming order management processes by automating workflows, optimizing inventory, and enhancing decision-making.
AI in record-to-report
AI is transforming the record-to-report (R2R) process by automating tasks, improving accuracy, and delivering valuable insights for better decision-making.
Generative AI for billing
The integration of generative AI in billing accelerates processing times and significantly reduces human errors, allowing businesses to provide better service while focusing on scaling their operations.
CUA models
CUA models, or Computer-Using Agent models, represent a significant advancement in the field of artificial intelligence, which are designed to interact with graphical user interfaces like humans.
AI in procure-to-pay processes
AI enhances the procure-to-pay cycle by automating routine tasks, improving decision-making, and ensuring better compliance, resulting in smoother, faster, and more efficient procurement operations.
Understanding vertical AI agents
Vertical AI agents are systems designed to perform industry-specific tasks, leveraging proprietary data and domain expertise to deliver highly tailored solutions.
Generative AI for contracts management
Generative AI in contract management leverages natural language processing to understand and interpret the complex language used in legal documents.
Generative AI in manufacturing
Generative AI is significantly transforming the manufacturing sector by enhancing operational efficiencies, driving innovation, and enabling mass customization.