Generative AI for financial reporting: Development, integration, use cases, benefits and future outlook
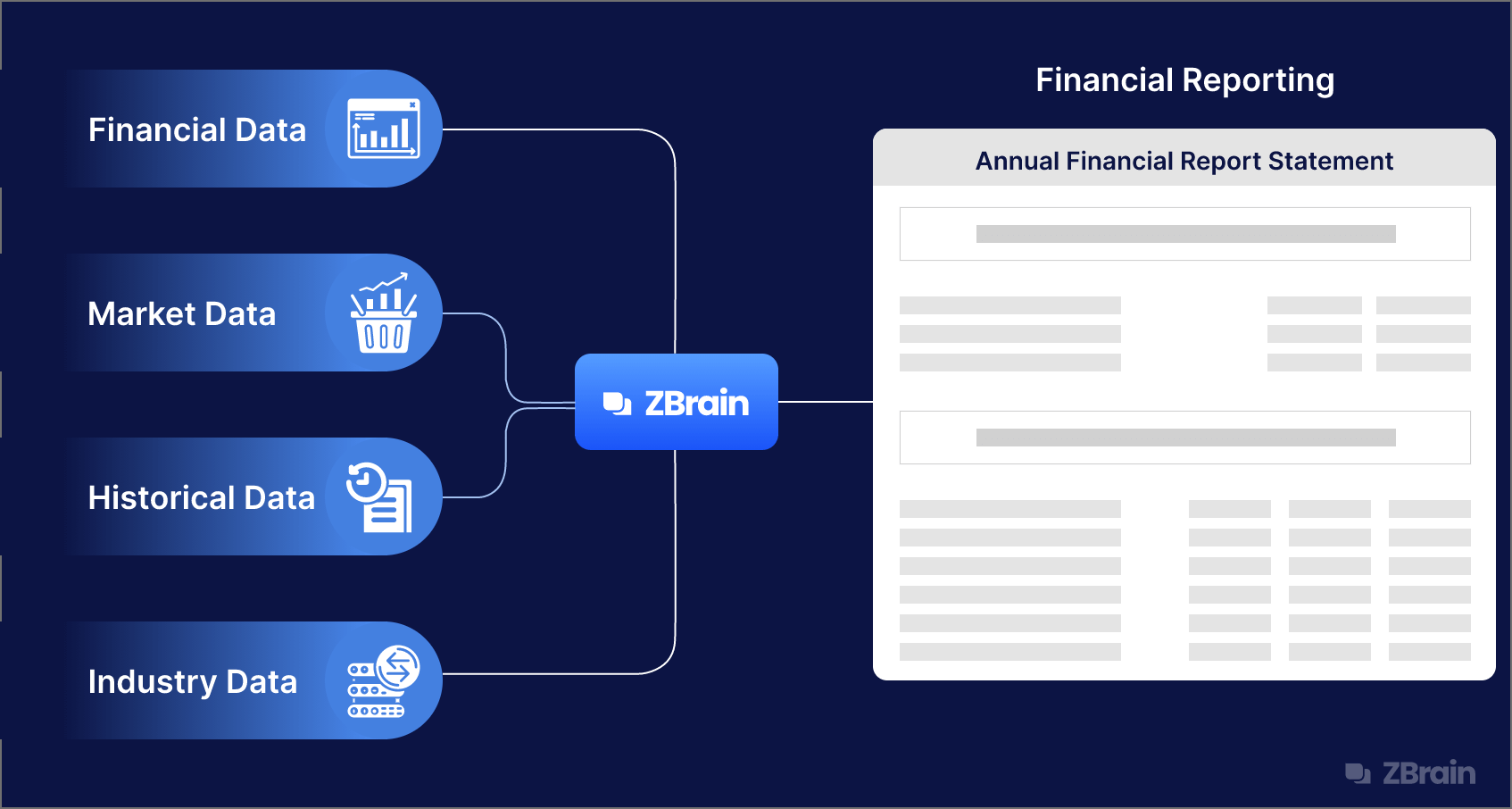
Listen to the article
Financial reporting is undergoing a significant transformation, fueled by the rapid emergence of generative artificial intelligence (AI). This powerful technology, capable of generating new content like text, images, and code, is poised to transform how businesses analyze financial data, generate reports, and make informed decisions. This document explores the various ways generative AI is being integrated into financial reporting systems, highlighting its potential to automate tasks, enhance accuracy, and provide deeper insights.
We delve into the different approaches companies are taking to adopt generative AI, from building custom AI stacks to utilizing pre-built solutions and adopting comprehensive platforms like ZBrain. Furthermore, we examine the key use cases of generative AI in financial reporting, including automated report generation, financial statement analysis, and fraud detection, among others.
This article also addresses the challenges and considerations associated with adopting generative AI, such as data quality, model bias, and ethical implications. It discusses strategies for mitigating these challenges and provides a glimpse into the future of generative AI for financial reporting, outlining its potential to enhance automation, insights, and transparency further.
- What is generative AI?
- AI in financial reporting
- The different approaches to integrating generative AI into financial reporting systems
- Why ZBrain is the ideal platform for financial reporting
- Key use cases of generative AI for financial reporting
- Challenges and considerations in adopting generative AI for financial reporting
- Future outlook of generative AI in financial reporting
- Transforming financial reporting with ZBrain: A full-stack GenAI orchestration platform
What is generative AI?
Generative AI is a type of artificial intelligence that produces new content—such as text, images, music, or code—based on patterns it has learned from existing data, typically in response to a prompt. These AI models can generate novel outputs by predicting and producing sequences that resemble the input they were trained on. For example, a generative AI model trained in vast amounts of text can write coherent paragraphs, while one trained in images can create new visuals.
A common type of generative AI is the Generative Adversarial Network (GAN), often used for generating images. Another widely used model for text generation is Generative Pre-trained Transformer, abbreviated as, GPT. The core function of generative AI is to automate the creation of new, usable outputs that can serve in creative, technical, or operational processes.
AI in financial reporting
AI is transforming financial reporting by automating tasks, improving accuracy, and providing deeper insights. Machine learning algorithms can analyze vast amounts of data, identify trends, and detect anomalies in financial statements, freeing up human analysts to focus on higher-level tasks. AI-powered tools can also generate reports, automate data entry, and translate financial information across languages, streamlining the reporting process and reducing the risk of errors. Moreover, AI can help organizations analyze future financial performance, identify potential risks, and make more informed business decisions, leading to greater efficiency and profitability.
The different approaches to integrating generative AI into financial reporting systems
The financial reporting landscape is rapidly evolving with the introduction of generative AI. Companies are exploring different strategies to leverage these powerful tools, each with its unique advantages and considerations:
- Custom AI stacks: This approach involves building a custom AI solution from scratch using various tools and models for specific business needs.
- Pros:
- Tailored solutions: Enables businesses to create tailored AI solutions that perfectly align with their specific needs and reporting requirements.
- Complete control: Provides complete control over the AI model selection, data integration, and workflow development, allowing for greater customization and flexibility.
- Enhanced innovation: Encourages experimentation and innovation in AI-driven financial reporting, fostering a competitive edge.
- AI point solutions: This involves utilizing pre-built AI applications focused on specific tasks within the financial reporting process. It could be a solution to automate the extraction of financial data from invoices and receipts, streamlining the bookkeeping process.
- Pros:
- Focused functionality: Offers dedicated AI solutions designed to address specific financial reporting challenges, such as automating data extraction or generating financial summaries.
- Ease of implementation: Typically easier to integrate and deploy compared to custom stacks, requiring less development effort and expertise.
- Cost-effectiveness: Often more cost-effective than building a full-fledged AI stack, particularly for businesses with limited resources.
- Fully integrated platforms like ZBrain: Generative AI orchestration platforms like ZBrain Builder are comprehensive solutions that can be used to build and deploy GenAI-powered financial reporting applications. They encompass everything from data management and model selection to workflow creation and solution deployment. They streamline the entire process by offering a unified platform for developing solutions that address all critical aspects of AI-powered financial reporting.
- Pros:
- End-to-end solution: Provides a comprehensive platform that facilitates AI model selection, data management, workflow development, and GenAI solution deployment, offering a holistic approach to AI-driven financial reporting.
- Rapid development and deployment: Their low-code interface allows for faster development and deployment of AI-powered financial reporting solutions..
- Streamlined integration: ZBrain can integrate smoothly with existing financial systems, ensuring seamless data flow and minimizing disruption during adoption.
- Rapid development and deployment: Utilizes low-code or no-code interfaces, allowing for faster development and deployment of AI-powered financial reporting solutions.
Choosing the right approach
The best approach depends on the specific needs, resources, and technical expertise of each organization. Custom AI stacks offer maximum control but require substantial investment. Point solutions are affordable and efficient for specific tasks, while fully-integrated platforms provide a comprehensive and scalable solution for complex financial reporting requirements. Ultimately, the key is to carefully assess the benefits and challenges of each approach and select the one that best aligns with the organization’s goals and resources.
Why ZBrain Builder is the ideal platform for financial reporting
ZBrain, with its generative AI capabilities, can automate various aspects of financial reporting. Its comprehensive suite of features is designed to streamline and enhance the financial reporting process. Its robust data ingestion and knowledge base capabilities allow for seamless integration with various financial data sources, ensuring a complete and accurate picture for analysis. ZBrain Builder’s low-code interface empowers users to create complex GenAI solutions with minimal coding, accelerating the development and deployment processes. Its model agnosticism ensures compatibility with a wide range of public and private LLMs, providing flexibility to choose the most appropriate model for specific needs. Furthermore, ZBrain’s evaluation suite and continuous improvement features ensures the accuracy and quality of AI outputs, ensuring reliable and insightful financial reporting. These features, combined with its focus on security and scalability, make ZBrain suitable for organizations looking to leverage generative AI to transform their financial reporting processes.
Optimize Your Operations With AI Agents
Our AI agents streamline your workflows, unlocking new levels of business efficiency!
Key use cases of generative AI for financial reporting
Use case |
Description |
How ZBrain helps |
Automated report generation |
Generating financial reports, such as income statements, balance sheets, and cash flow statements, based on raw data. |
ZBrain can analyze vast datasets, extract key information, and automatically generate accurate and insightful financial reports in various formats. |
Data extraction and summarization |
Extracting relevant information from financial documents like invoices, contracts, and regulatory filings to streamline analysis. |
ZBrain solutions can efficiently scan documents, identify key data points, and summarize them into concise and actionable insights, saving time and improving accuracy. |
Financial analysis |
Analyzing financial data to identify trends, assess historical performance, and inform investment decisions.
|
ZBrain can analyze historical data, integrate market trends, and generate data-driven insights, providing a more holistic view of financial performance and potential risks. |
AI-enhanced financial compliance |
Automating the process of reviewing financial reports and ensuring compliance with regulations and accounting standards. |
ZBrain solutions can scan financial documents for potential discrepancies and non-compliance issues, flagging risks. Its GDPR compliance monitoring agent ensures that all financial processes comply with the General Data Protection Regulation (GDPR), flagging any potential violations for review. It enhances compliance monitoring, helping finance teams uphold robust data protection. |
Risk management and fraud detection |
Identifying and mitigating potential financial risks, such as fraud, credit risk, and market volatility. |
ZBrain’s financial risk mitigation agent identifies and mitigates financial risks by analyzing operational, market, and credit risk factors. This intelligent agent flags threats and suggests mitigation strategies, empowering finance teams to address risks and proactively maintain stability. |
Personalized financial advice |
Providing customized financial advice to clients based on their individual needs and financial situations. |
ZBrain’s ability to analyze data and generate personalized insights allows for the development of tailored financial recommendations, improving client satisfaction and service quality. |
Customer service automation |
Automating customer interactions related to financial inquiries and transactions, providing quicker and more efficient service. |
ZBrain can power chatbots and virtual assistants that can answer basic financial questions, guide customers, and provide personalized support, freeing up human agents to handle more complex issues. |
Market research and competitive analysis |
Analyzing industry trends, competitor activities, and market data to identify growth opportunities. |
ZBrain can integrate various sources of market data, identify patterns and trends, and generate insightful reports that help businesses make informed strategic decisions. |
Challenges and considerations in adopting generative AI for financial reporting
While generative AI offers significant potential to transform financial reporting, its adoption comes with several challenges and considerations:
Data quality and integrity
- Data accuracy: Generative AI models are only as good as the data they are trained on. Inaccurate or incomplete financial data can lead to misleading or erroneous reports.
- Data security and privacy: Financial data is highly sensitive. Ensuring the security and privacy of this data during AI model training and usage is crucial.
- Data governance and compliance: Financial institutions must adhere to strict data governance and compliance regulations. AI models need to be designed and deployed in a way that respects these regulations.
Model bias and explainability
- Algorithmic bias: AI models can inherit biases from the training data, which can lead to unfair or discriminatory outcomes in financial reporting.
- Explainability and transparency: It’s essential to understand how AI models arrive at their conclusions to ensure transparency and accountability. The “black box” nature of some AI models can make it difficult to interpret and explain their decisions.
Human oversight and control
- Human validation and verification: AI models should not replace human oversight entirely. Financial reports require human judgment and validation to ensure accuracy and ethical considerations.
- Skill gap and training: Implementing generative AI requires a skilled workforce with expertise in AI, data science, and finance. Organizations need to invest in training and upskilling their staff to effectively utilize and manage these technologies.
Ethical and regulatory considerations
- Misuse and manipulation: There are concerns about the potential for misuse of generative AI for financial reporting, such as creating fabricated reports or manipulating data for fraudulent purposes.
- Regulatory landscape: The regulatory landscape for AI in finance is still evolving. Organizations need to stay informed about emerging regulations and ensure their AI implementations comply with these rules.
Cost and infrastructure
- High initial investment: Implementing generative AI for financial reporting requires significant upfront investment in technology, data, and specialized expertise.
- Scalability and maintenance: Organizations need to consider the long-term costs of scaling AI systems, maintaining them, and adapting to evolving technologies.
Other considerations
- User adoption: Encouraging user adoption and ensuring seamless integration into existing workflows can be challenging.
- Data integration and interoperability: Integrating AI models with existing financial reporting systems and data sources can be complex.
- Continuous improvement: Generative AI models require ongoing evaluation and refinement to ensure their accuracy and effectiveness.
Mitigating challenges
ZBrain addresses many of the challenges associated with adopting generative AI for financial reporting, providing a secure, robust, and user-friendly platform. Here’s how:
- Data validation and cleaning: ZBrain offers tools for data validation and cleaning, ensuring that the data used is accurate and free from errors. This helps mitigate the risk of misleading or inaccurate reports.
- Secure data handling: ZBrain prioritizes data security and privacy, offering features like encryption, access control with Single Sign-On (SSO), role-based permissions and private deployments to protect sensitive financial data.
- Human-in-the-loop feedback: ZBrain integrates human feedback loops, allowing financial professionals to validate and refine AI outputs and reduce model bias, ensuring accuracy and ethical considerations.
- User-friendly interface: ZBrain’s intuitive low-code interface empowers users to manage and control AI solution development, eliminating the need for extensive technical expertise.
- Compliance features: ZBrain is designed with compliance in mind, offering features to ensure adherence to regulatory frameworks and data privacy regulations.
- Scalable and efficient: The platform’s cloud-based architecture allows organizations to scale AI implementations efficiently and optimize infrastructure costs.
- Low-code development: ZBrain Builder’s user-friendly, low-code interface can potentially reduce development time and costs associated with building GenAI solutions.
- Seamless integration: ZBrain integrates smoothly with existing financial systems, ensuring seamless data flow and minimizing disruption during adoption.
By addressing these challenges and considerations carefully, organizations can unlock the full potential of generative AI for financial reporting, enabling them to generate more accurate, insightful, and efficient reports, enhancing their financial decision-making capabilities and improving overall business performance.
Optimize Your Operations With AI Agents
Our AI agents streamline your workflows, unlocking new levels of business efficiency!
Future outlook of generative AI in financial reporting
The future of generative AI in financial reporting is bright, promising significant transformation and innovation across the industry. Here’s a glimpse into the potential trajectory:
Increased automation and efficiency
- End-to-end automation: Generative AI will likely automate even more aspects of financial reporting, from data collection and analysis to report generation and presentation. This will lead to significant time and cost savings, freeing up finance professionals to focus on higher-value activities like strategic analysis and decision-making.
- Real-time reporting: Generative AI models will enable real-time financial reporting, allowing businesses to access up-to-date insights and make more informed decisions in dynamic market conditions.
Enhanced insights and analysis
- Personalized reporting: Generative AI will personalize financial reports based on individual user needs and preferences, providing tailored insights and recommendations.
- Advanced risk management: AI will play a larger role in identifying and mitigating financial risks, enabling more sophisticated risk management strategies and proactive risk mitigation.
Improved transparency and trust
- Explainable AI: Research and development in explainable AI will lead to more transparent and interpretable models, increasing trust in AI-generated reports and fostering greater accountability.
- Auditable AI: As regulations evolve, AI models will be designed to be auditable, ensuring compliance and accountability.
New applications and use cases
- Financial storytelling: Generative AI will create more engaging and informative financial reports, transforming them into compelling narratives that convey complex information effectively to diverse audiences.
- Personalized financial advice: AI will become even better at providing tailored financial advice, helping individuals and businesses make informed financial decisions based on their specific circumstances.
- Financial chatbots and virtual assistants: AI-powered chatbots and virtual assistants will provide on-demand financial support and guidance, enhancing customer service and streamlining communication.
Challenges and opportunities
- Ethical considerations: As generative AI becomes more sophisticated, the ethical implications of its use in finance will require careful consideration.
- Regulatory landscape: The regulatory landscape for AI in finance will continue to evolve, requiring constant adaptation and compliance.
- Talent development: The demand for skilled professionals in generative AI and finance will continue to grow, highlighting the need for robust training and upskilling programs.
Overall, the future of generative AI for financial reporting is promising, but it will require a collaborative effort from stakeholders across the industry to address ethical concerns, navigate regulatory changes, and invest in talent development. By embracing this technology responsibly, the finance industry can unlock new opportunities for efficiency, innovation, and better decision-making.
Transforming financial reporting with ZBrain: A full-stack GenAI orchestration platform
ZBrain, with its AI capabilities, helps organizations streamline financial reporting by automating data analysis, ensuring compliance, and improving accuracy. It provides a range of features designed to enhance efficiency, reduce errors, and support data-driven decision-making.
-
AI readiness assessment: ZBrain’s AI readiness assessment framework, ZBrain XPLR, evaluates an organization’s preparedness for AI adoption in financial reporting. It provides actionable insights to identify strengths, gaps, and opportunities for automation, ensuring a smooth AI implementation.
-
Low-code development: ZBrain’s low-code platform, ZBrain Builder, enables finance teams to create custom AI solutions for report generation, variance analysis, and compliance checks without requiring extensive technical expertise.
-
Proprietary data utilization: The platform allows organizations to leverage their proprietary financial data effectively, ensuring AI models are aligned with specific reporting requirements, accounting standards, and industry regulations.
-
Enterprise-ready: Designed for large-scale financial operations, ZBrain Builder offers security, scalability, and seamless integration with enterprise resource planning (ERP) and financial management systems, enabling efficient financial reporting at scale.
-
End-to-end support: ZBrain Builder manages the entire lifecycle of AI-driven financial reporting—from data ingestion to report generation and compliance validation—ensuring accurate, timely, and regulatory-compliant reporting.
-
Flexible data ingestion: ZBrain integrates financial data from multiple sources, including accounting software, enterprise databases, and compliance records, to provide real-time insights that improve reporting accuracy, risk assessment, and financial insights.
-
Intelligent agent creation: AI agents built on ZBrain Builder can automate key financial reporting tasks, such as variance analysis, fraud detection, and regulatory compliance checks, reducing manual effort and enhancing reporting accuracy.
These capabilities position ZBrain as a powerful tool for organizations looking to optimize their financial reporting processes, ensuring accuracy, efficiency, and compliance in an increasingly complex regulatory landscape.
Endnote
The adoption of generative AI in financial reporting can potentially transform the industry, offering tremendous potential for efficiency, accuracy, and insightful decision-making. However, it is crucial to approach this transformation with a balanced perspective, acknowledging the inherent challenges and considering the ethical and regulatory implications.
By investing in data quality, developing robust governance frameworks, and addressing the explainability and bias concerns, we can harness the power of AI while ensuring responsible and ethical use. Continuous learning, adaptation, and collaboration between industry stakeholders will be essential to navigate the evolving landscape of generative AI in finance.
As we move forward, it is vital to remember that AI is a tool, not a replacement for human judgment and expertise. By embracing a collaborative approach, combining human intelligence with the power of AI, we can unlock the full potential of this transformative technology and build a more robust, efficient, and ethical financial ecosystem.
Listen to the article
- What is generative AI?
- AI in financial reporting
- The different approaches to integrating generative AI into financial reporting systems
- Why ZBrain Builder is the ideal platform for financial reporting
- Key use cases of generative AI for financial reporting
- Challenges and considerations in adopting generative AI for financial reporting
- Future outlook of generative AI in financial reporting
- Transforming financial reporting with ZBrain: A full-stack GenAI orchestration platform
Frequently Asked Questions
What is ZBrain, and how can it optimize financial reporting with generative AI?
ZBrain is an end-to-end AI enablement platform that assists businesses in streamlining AI adoption across various functions, including financial reporting. From assessing AI readiness to solution development and deployment, ZBrain offers comprehensive support to optimize workflows, ensure compliance, and enhance the accuracy of financial statements.
Here’s how ZBrain enhances financial reporting:
AI readiness assessment with ZBrain XPLR: ZBrain XPLR provides a comprehensive AI readiness assessment, enabling organizations to evaluate current processes and identify strategic opportunities for AI integration in financial reporting. This enhances operational efficiency and supports data-driven decision-making.
Seamless data ingestion and integration: ZBrain Builder integrates with various financial systems, ensuring smooth data flow. This enables businesses to create accurate, real-time financial reports by combining structured and unstructured data for better analysis and compliance decisions.
Low-code development environment: ZBrain Builder’s intuitive, low-code interface empowers teams to quickly build and deploy AI-driven solutions with minimal coding expertise. This accelerates the automation of financial processes, from report generation and data consolidation to compliance monitoring.
Cloud and model flexibility: ZBrain supports various AI models and integrates seamlessly with cloud platforms like AWS, Azure, and GCP, providing the flexibility to select the optimal infrastructure for cost-effective, scalable financial reporting solutions.
Enhanced compliance and governance: ZBrain’s AI capabilities help ensure continuous monitoring and compliance with industry regulations, tax laws, and internal policies related to financial reporting. By flagging potential risks in reporting and data management, ZBrain strengthens operational governance and audit readiness.
By offering a low-code platform with powerful data integration and customizable AI capabilities, ZBrain enables organizations to automate, optimize, and innovate their financial reporting processes, enhancing accuracy, reducing manual effort, and improving profitability.
How does ZBrain ensure the security and privacy of sensitive data in financial reporting processes?
ZBrain is designed with a strong emphasis on data privacy and security, ensuring that sensitive financial information is protected throughout all stages of financial reporting. Here’s how ZBrain safeguards your data:
-
Private cloud deployments: ZBrain AI agents can be deployed in private cloud environments, ensuring that critical financial data—such as financial statements, transaction records, and audit trails—is securely stored within your organization’s infrastructure.
-
Robust security features: ZBrain incorporates multiple layers of security to protect sensitive data, including:
-
Access controls: Granular role-based access controls ensure only authorized personnel can view or manage sensitive financial information, such as financial reports, budgets, and forecasts.
-
Compliance and Governance: ZBrain is built to adhere to industry-specific regulations and standards ISO 27001:2022 and SOC 2 Type II, ensuring that financial data is handled in compliance with confidentiality, integrity, and accountability requirements.
This comprehensive security approach ensures that sensitive financial data remains protected throughout its lifecycle—from data collection and processing to reporting and auditing.
Can ZBrain AI agents be integrated with existing financial reporting systems?
Yes, ZBrain AI agents are designed to integrate seamlessly with existing financial reporting systems. The platform supports various data formats and standards, ensuring smooth interoperability with legacy ERP, accounting, and financial management systems.
This integration allows organizations to:
-
Leverage existing infrastructure: Enhance current financial reporting processes without the need for a complete overhaul of legacy systems.
-
Enrich data and workflows: Connect ZBrain AI agents with existing tools to automate data consolidation, variance analysis, and compliance monitoring, improving data accessibility and efficiency.
-
Drive AI-driven insights: Utilize gen AI capabilities to generate real-time financial reports, detect anomalies, and enhance decision-making while maintaining compatibility with existing technologies.
By enabling seamless integration, ZBrain ensures that organizations can modernize their financial reporting processes without disrupting existing systems, thereby improving overall operational efficiency.
What kind of financial reporting agents can be built on ZBrain Builder?
ZBrain Builder enables the development of AI agents tailored to various financial reporting use cases. These agents support tasks such as automated financial statement analysis, variance analysis, fixed asset accounting, and audit trail generation. ZBrain’s advanced gen AI capabilities help organizations optimize data integration, automate manual tasks, and provide AI-driven insights to enhance decision-making. ZBrain helps businesses deliver more efficient and responsive financial operations by streamlining workflows and improving financial reporting processes.
How does ZBrain cater to diverse financial reporting needs across business operations?
ZBrain’s flexibility allows it to address various financial reporting requirements. You can create tailored AI agents to automate financial statement generation, streamline data consolidation, manage compliance reporting, and ensure accuracy. Its efficient AI agents enable businesses to enhance efficiency, precision, and financial decision-making across various financial reporting processes in any industry.
How can we measure the ROI of ZBrain in our financial reporting processes?
Measuring the return on investment (ROI) of ZBrain in financial reporting involves evaluating key performance indicators (KPIs) related to automation, accuracy, efficiency, and compliance. Here are some important metrics to consider:
-
Reduced manual effort: Automating tasks such as data consolidation, report generation, and variance analysis leads to faster processing, fewer errors, and improved accuracy.
-
Faster financial close: Streamlining financial reporting workflows and ensuring timely data processing improves cash flow and accelerates the financial close process.
-
Improved accuracy in reporting and compliance: Automated validation processes reduce errors, ensuring accurate financial reporting and adherence to regulatory requirements.
-
Operational efficiency: Streamlined workflows, real-time data updates, and automated processes help lower operational costs and improve overall financial reporting performance.
By monitoring these KPIs, businesses can quantify how ZBrain drives efficiency, reduces errors, and optimizes financial reporting processes.
How can I get started with ZBrain for my financial reporting processes?
To begin using ZBrain to optimize your financial reporting processes, please reach out to us at hello@zbrain.ai or fill out the inquiry form on our website. Our team will get in touch with you to explore how our platform can integrate with your existing financial reporting systems and streamline financial reporting workflows.
Insights
How ZBrain’s multi-agent collaboration works
ZBrain’s multi-agent collaboration is an advanced approach in which multiple specialized AI agents work together as a cohesive team to solve complex enterprise challenges.
ZBrain’s modular stack for custom AI solutions
ZBrain Builder’s modular architecture – comprising data ingestion pipelines, a knowledge base, an LLM orchestration layer, automation agents, and integration-ready interfaces – serves as a set of building blocks for enterprise AI solutions.
AI in project and capital expenditure management
Adopting AI across the capital expenditure (CapEx) lifecycle can radically improve how organizations plan, execute, and evaluate big investments.
AI for plan-to-results
The integration of AI into plan-to-results operations is transforming how organizations align strategic planning with execution to optimize business performance.
AI for accounts payable and receivable
The integration of AI in AP and AR operations extends beyond routine automation, offering strategic insights that empower finance professionals to evolve from reactive problem-solvers into proactive strategists.
AI for HR planning and strategy
Integrating AI into HR planning and strategy is revolutionizing how organizations manage their workforce, optimize talent, and align HR initiatives with business goals.
AI in quote management
AI is redefining quote management by automating complex processes, improving pricing accuracy, and accelerating approval workflows.
Generative AI for sales
The role of generative AI in sales is expanding rapidly, making it a critical tool for organizations seeking to stay competitive.
AI for control and risk management
AI is increasingly revolutionizing control and risk management by automating labor-intensive tasks, monitoring compliance in real-time, and enhancing predictive analytics.