Monitoring ZBrain AI agents: Significance, key metrics, best practices, benefits and future trends
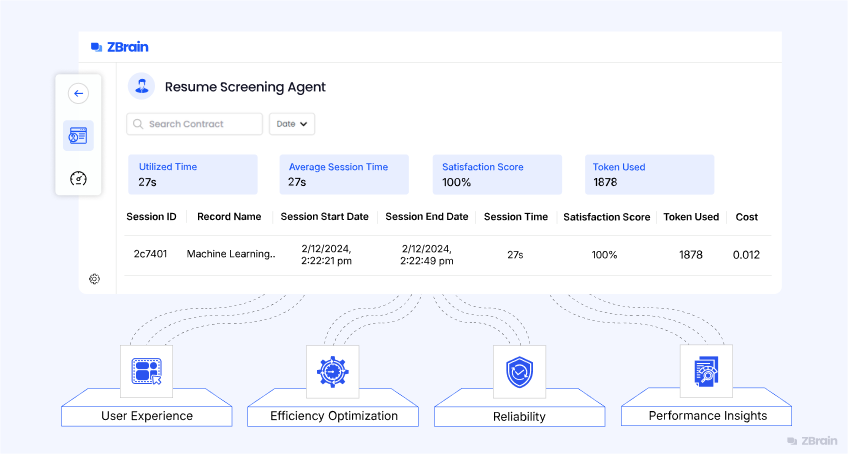
Listen to the article
In today’s digital era, AI agents are no longer just an option—they’re essential. As the backbone of modern business operations, these intelligent tools redefine efficiency, streamline costs, and transform interactions across industries. Their integration spans from everyday enterprise workflows to complex applications, marking a crucial evolution in business technology use.
By 2025, the AI agent market is projected to surge to $7.63 billion. What’s driving this growth? A staggering 85% of enterprises are expected to deploy AI agents, driven by the promise of significant automation gains and efficiencies. A 2024 Capgemini report highlights a compelling trend: 82% of companies plan to integrate AI agents within the next three years, anticipating significant benefits in automation and improved efficiency.
However, the path to operationalizing AI is still fraught with challenges. Integration isn’t seamless—85% of IT leaders admit that blending AI agents into existing systems presents significant hurdles. Data security is another major concern, with 76% of enterprises expressing concerns about protecting sensitive information handled by AI agents. 70% of consumers demand transparency in AI-driven decisions. 39% of consumers strongly trust AI when its benefits are clear and its operations ethical.
So, how can we maximize the benefits of AI agents while overcoming these challenges? One effective strategy is to monitor their performance. AI agent monitoring involves tracking AI agents’ performance, behavior, and interactions. This includes real-time monitoring of AI agents task execution, time required to complete tasks, accuracy and other parameters. Effective monitoring of AI agents ensures they perform optimally and align with business goals. Overall, it involves tracking key metrics to evaluate efficiency, compliance, and impact, turning AI agents into strategic assets.
ZBrain is a unified AI enablement platform that supports enterprises from AI readiness evaluation to full-scale implementation. Its AI agents streamline processes and enhance productivity across organizations, providing practical intelligence for various departmental needs. These agents improve task efficiency and decisions and empower teams to focus on strategic goals. This insight covers AI agent monitoring, the essential metrics for evaluating AI agents, effective monitoring strategies for ZBrain AI agents, and best practices to ensure optimal performance.
- Why monitoring AI agents is essential?
- Understanding ZBrain metrics for agent monitoring
- Post-deployment monitoring and effective management of ZBrain AI agents
- Best practices for monitoring AI agents
- Key benefits of monitoring AI agents
- The future of AI agent monitoring: Key trends and enhancements
Why monitoring AI agents is essential?
As AI agents become core to business operations, ensuring their consistent performance post-deployment is critical. It requires ongoing oversight. AI agent monitoring is the practice of systematically tracking and analyzing an agent’s behavior, outputs, and impact to ensure it operates reliably, fairly, and efficiently. Without it, organizations risk blind spots in decision-making, compliance, and value realization. Monitoring AI agents is critical for organizations deploying these systems, as it addresses several fundamental challenges unique to AI implementation:
-
Performance variability management: AI agents are highly responsive to input complexity, exhibiting dynamic behavior that adapts to a wide range of scenarios. Unlike traditional software, their output may vary depending on context, which underscores the importance of continuous monitoring. This helps establish reliable performance baselines and ensures consistent optimization over time.
-
Explainability and transparency: Overall, monitoring helps track how AI makes decisions, which is essential for regulatory compliance and building trust with stakeholders. This is particularly important in high-stakes domains like healthcare, finance, and legal applications. Monitoring decision paths, outcomes and stepwise execution in agents improves transparency, helping teams debug logic issues and meet audit requirements.
-
Detection of subtle degradation: AI performance can degrade gradually and often imperceptibly until serious issues emerge. To be very specific, agents relying on retrievers, long prompts, or dynamic contexts can degrade silently if tools fail, embeddings drift, or workflows grow stale. Continuous monitoring creates early warning systems that catch declining performance before it impacts business operations.
-
Multidimensional success evaluation: AI systems require complex, multifaceted evaluation metrics beyond traditional software measurements. Effective monitoring approaches track these diverse metrics, from accuracy and speed to problem-solving capabilities and customer satisfaction scores.
-
Business value validation: Monitoring provides concrete data to justify ongoing AI investments by demonstrating measurable business impact. For SMBs, properly monitored AI implementations can substantially save costs while maintaining or improving service quality. Monitoring helps link agent output to KPIs, such as cost savings, resolution time, or lead conversion.
-
Quality control and customer experience: For customer-facing AI applications, monitoring ensures interactions meet quality standards, enhancing satisfaction. Tracking metrics like response accuracy and problem-solving success rates helps refine AI agent’s behaviors based on user interactions.
-
Operational optimization: Comprehensive monitoring identifies bottlenecks, inefficiencies, and opportunities for improvement in AI agent deployment, allowing organizations to maximize operational benefits.
-
Human-AI collaboration metrics: For systems designed to work alongside humans, monitoring the effectiveness of these partnerships and handoffs between AI and human workers becomes important.
AI agents require continuous monitoring to ensure reliability, transparency, and sustained performance across evolving business contexts. By tracking the right metrics, organizations can proactively optimize agent behavior and drive measurable value.
Potential challenges in AI agent monitoring
AI agent development introduces a dynamic and nuanced challenge that necessitates an iterative and adaptive approach to evaluation, making effective monitoring difficult to implement.
-
Data variability management: AI agents encounter diverse and unpredictable scenarios, making standard performance metrics potentially unreliable without proper contextual understanding.
-
Reliability maintenance: As AI systems evolve through continuous learning, ensuring consistent performance over time becomes increasingly complex.
-
Metric accuracy: Traditional performance metrics often fail to capture the nuanced capabilities of AI agents, particularly for complex tasks requiring sophisticated decision-making.
-
Scale and speed: AI agents can rapidly make thousands of decisions, making comprehensive real-time monitoring computationally intensive and potentially cost-prohibitive.
-
Resource constraints: Implementing robust monitoring systems requires significant computational resources, which could potentially create performance overhead or increase operational costs.
-
Balancing autonomy with control: Excessive monitoring might restrict an AI system’s adaptability, while insufficient oversight creates safety and performance risks.
-
Technical complexity: Most AI solutions based on complex algorithms and deep learning are opaque (Black boxes) and complex, which makes understanding and monitoring their internal workings difficult. This complexity can obscure decisions, complicating efforts to diagnose and fix issues.
Organizations that implement comprehensive benchmarks, continuous training programs, and real-time data analysis can overcome these challenges and transform their AI agents into reliable, effective business tools that consistently deliver measurable value.
Understanding ZBrain metrics for agent monitoring
ZBrain provides built-in capabilities for monitoring AI agent performance with specialized metrics and a user dashboard designed for operational clarity:
- Processing time: Measures the total duration for the agent to complete a task. This metric is crucial for assessing the agent’s efficiency in handling operations and identifying tasks that may require optimization.
- Session time: This represents the total time spent from the beginning to the end of a session, giving insights into the overall engagement duration with the agent. It can help understand how long users interact with the agent on average.
- Satisfaction score: User feedback rating the agent’s performance. This score is integral for gauging user satisfaction and helps correlate user experience with the agent’s technical performance.
- Tokens used: This tracks the number of computational tokens consumed during task execution. This metric is vital for managing operational costs and assessing the agent’s resource efficiency.
- Cost: Calculates the financial cost associated with executing tasks per session based on token consumption. This allows for effective budget management and helps evaluate the cost-efficiency of deploying the AI agent.
ZBrain makes these metrics accessible from each agent’s dedicated dashboard, where users benefit from:
- Comprehensive session logs: Detailed records of every agent interaction, including timestamps, inputs, outputs, and status.
- Input/output data tracking: This provides visibility into what information was provided to the agent and what results were generated.
- Status filtering: The ability to quickly sort tasks by their current status (pending, completed, failed) for efficient troubleshooting.
- Detailed Flow logs: Insights into specific flows employed by agents, including their status and timestamps.
- Feedback collection: An option to integrate user feedback on common issues and any specific aspects.
This integrated monitoring setup allows ZBrain users to track performance metrics in real-time and refine agents based on operational feedback, usage patterns, and business outcomes. The platform’s unified view of technical and business metrics enables data-driven optimization that balances performance with cost-effectiveness.
Optimize Your Operations With AI Agents
Our AI agents streamline your workflows, unlocking new levels of business efficiency!
Post-deployment monitoring and effective management of ZBrain AI agents
Effectively managing and monitoring AI agents is essential for leveraging their full capabilities to enhance business operations. This comprehensive section outlines how to use ZBrain’s sophisticated tools to ensure your AI agents perform optimally, align with business goals, and adapt seamlessly to changing needs.
Once an agent is deployed, follow these key steps to maintain performance and proactively manage issues:
-
Access uploaded documents: Begin by navigating to the Agent Dashboard where you can review and verify that all uploaded documents are processed correctly. This step ensures that the agents are working with the correct and intended data.
-
Review activity logs and reports: Regularly check the logs and generated reports to monitor the agent’s performance. This will help you detect any operational issues or anomalies in real-time.
-
Monitor the agent’s performance: Visit the Performance page to track essential metrics such as utilized time, satisfaction score, tokens used, etc. These metrics are crucial for ensuring that the agents are operating efficiently, delivering consistent results, and aligning with your overall business goals.
By thoroughly testing and deploying the agent, you ensure its reliability and smooth integration into your workflow. Ongoing monitoring after deployment helps maintain performance and address issues proactively. Here is a detailed guide on monitoring task status, viewing detailed logs, filtering and reviewing tasks, and assessing agent performance.
Accessing agents
The Agents Dashboard is your central command center for monitoring all AI agents. It provides a quick snapshot of each agent’s status, including:
-
Agent ID and name: Quickly identify each agent for easier management. Each agent has a unique ID (like 2c5efa) and descriptive name (such as Resume Screening Agent) for easy reference and management.
-
Accuracy and status: The dashboard provides immediate insight into each agent’s performance and operational status, helping you gauge which agents are performing as expected and which may require troubleshooting.
-
Accuracy: The number of times the agent has run successfully without failing any run. The dashboard displays accuracy percentages for agent’s task execution.
-
Status indicators: Indicates agent status such as Active or Draft.
-
-
Action required: Redirects to specific immediate actions needed for the successful execution of an agent’s failed tasks.
-
Last task execution: Date and time when the agent last ran a task.
-
Tasks completed: Total number of tasks successfully executed by the agent.
Monitoring task status
On the Queue page, you can see the real-time status of tasks assigned to your agents. This feature allows you to quickly identify and resolve issues to maintain operational flow:
-
Green dot: Successfully completed tasks.
-
Yellow dot: Tasks that are pending or in progress.
-
Red dot: Any failed tasks.
Analyzing session logs
For more granular insights, the session logs provide a detailed overview of what each agent does during task execution. This includes:
-
Input and output data: Examining the data processed by agents and their results can help understand the efficacy of each operation and identify potential areas for improvement.
-
Step-by-step overview: An agent activity section provides a breakdown of the agent’s actions during task execution. It provides each action’s status, timing, input and output for detailed analysis.
Reporting and feedback
Download or export session reports for comprehensive analysis. If the outcomes from an agent’s activities fall short of expectations, providing feedback directly through the platform is crucial. This functionality helps refine the agent’s performance and tuning the AI models according to real-world results and feedback.
-
Rating system: Use the thumbs-down option to submit your feedback on results, which can help refine agent performance over time.
-
Detailed feedback categories: Select predefined specific issue types such as “Not following company’s guidelines” or “Not factually correct” to highlight specific problems.
-
Custom feedback: Add specific details in the text field to guide improvements, such as instructions to prioritize specific values or generate results in a specific way.
Task management
Optimize your workflow management by filtering tasks based on their status. The Filter Queue option on the Queue page streamlines task management by sorting tasks based on their current status. This tool helps prioritize actions based on the immediacy and status of tasks, ensuring that resources are allocated effectively and that time-sensitive operations are addressed promptly.
-
Processing: Focus on tasks actively being executed to monitor current workloads.
-
Completed: Review successfully finished tasks to analyze successful outcomes.
-
Failed: Identify problematic tasks to prioritize troubleshooting efforts where they’re most needed.
-
Pending: Identify pending tasks in the queue.
View detailed agent performance
The Performance dashboard offers a comprehensive overview of various performance metrics. It reflects key metrics that help teams assess both operational efficiency and user impact. These include:
-
Utilized time: The total time the agent was active during interactions.
-
Average session time: The average time users interact with the agent per session.
-
Satisfaction score: User feedback rating the agent’s performance. This metric provides insight into the overall effectiveness of each session.
-
Tokens used: A metric for tracking the token consumption during task execution. This metric is critical for managing operational costs and understanding the resource utilization of each agent.
-
Cost: This metric indicates the total amount charged for each session based on token usage.
-
Session time: Total time spent per session. Captures the total duration of each session, offering insight into engagement length or task complexity.
-
Session start date: The date and time when the session began.
-
Session end date: The date and time when the session was concluded.
The “Select Date Range” option enables users to define a specific timeframe for viewing these performance metrics.
Flow-related details
On the All Flows Page, review the specific workflows that automate your agents’ tasks. It offers a detailed look at the steps involved in each workflow:
-
Flow name: Identifies each workflow used by the agent.
-
Updated: The date and time when each flow was last updated. It also provides date-wise sorting options for the flow list.
-
Flow status: Current status of the flow with toggle option to change it – enable or disable.
By diligently accessing, monitoring, and managing your ZBrain AI agents using these structured approaches, you can maximize their efficiency, ensure seamless integration into business processes, and adapt quickly to evolving operational demands. This proactive management not only maintains high performance but also fosters continuous improvement, making AI agents invaluable assets to your organization.
Best practices for monitoring AI agents
Monitoring AI agents is not just a technical necessity—it’s a strategic imperative. From ensuring real-time reliability to long-term cost efficiency, effective monitoring helps teams identify issues early, validate agent behavior, and continuously refine performance. The following best practices provide a strong foundation for scalable, secure, and intelligent agent oversight.
1. Establish real-time monitoring from day one
Set up observability and monitoring tools early in the agent development lifecycle. Logging and tracing should be embedded into workflows before agents move to production.
Key practices:
-
Capture detailed logs of each execution step, including function/tool calls, context usage, and response latency.
-
Monitor system health in real time—track CPU, memory, and token consumption to detect overloads or failure patterns.
-
Configure automated alerts for high-latency sessions, failed tasks, or unusual cost spikes.
This proactive approach prevents blind spots and enables teams to resolve performance issues before they impact end users.
2. Use dashboards as a central monitoring hub
Custom dashboards are essential for visualizing and responding to live performance signals. They centralize critical metrics and provide clarity for both technical and business stakeholders.
Dashboard best practices:
-
Highlight key indicators like response time, success rate, token usage, and satisfaction score.
-
Set custom alerts for deviation thresholds—such as drops in task success or spikes in token consumption.
-
Visualize historical performance to spot trends, regressions, or emerging patterns over time.
An effective dashboard transforms data into decisions—supporting daily operational control and long-term agent optimization.
3. Conduct regular data reviews with human oversight
Automated monitoring is powerful, but human judgment adds essential context—especially in cases of ambiguous agent behavior.
Recommended practices:
-
Review task sessions weekly or monthly to audit failure reasons and behavioral edge cases.
-
Use diagnostic tools (e.g., confusion matrices or input-output analysis) to evaluate accuracy trends.
-
Pair these reviews with scheduled security checks, including access controls and data protection audits.
A structured review cadence ensures the agent remains aligned with evolving user expectations and compliance requirements.
4. Leverage advanced monitoring techniques
Move beyond static thresholds with adaptive, intelligent monitoring. These methods allow teams to anticipate problems, rather than react to them.
Advanced methods include:
-
Implementing evaluation frameworks that assess routing logic, tool usage, and iteration loops.
-
Using A/B testing and controlled experiments to compare prompt variants, workflows, or response strategies.
-
Tracking agent “execution paths” to identify unnecessary loops, repeated steps, or failed tool sequences.
These techniques help refine both agent architecture and user outcomes—based on real behavioral data, not guesswork.
5. Adopt a proactive, iterative monitoring culture
Monitoring is not a one-time setup—it’s an ongoing process. Treat it as a strategic function that evolves with your AI agents.
Operational tips:
-
Audit your monitoring setup quarterly to identify process gaps, inefficiencies, or technical bottlenecks.
-
Use feedback loops (via agent rating systems or session scoring) to drive iterative improvements.
-
Stay aligned with emerging observability standards to future-proof your setup as the ecosystem matures.
When monitoring is built into the core of your agent orchestration framework, you ensure every deployment is measurable, improvable, and resilient.
Optimize Your Operations With AI Agents
Our AI agents streamline your workflows, unlocking new levels of business efficiency!
Key benefits of monitoring AI agents
Let’s explore the key benefits of monitoring AI agents in this section:
- Performance insights:
- Monitoring provides crucial data on AI agent performance, including accuracy, response times, and satisfaction score, etc. For instance, ZBrain’s ‘Utilized Time’ metric reveals how long agents take to complete tasks, helping teams identify and fix performance bottlenecks.
- Efficiency optimization:
- By identifying resource usage patterns, monitoring helps optimize the cost-effectiveness and scalability of operations. ZBrain’s ‘Tokens Used’ metric measures how efficiently the agent uses computational resources, enabling precise cost control.
- Reliability tracking:
- Consistent performance is crucial for the dependability of AI agents. ZBrain’s ‘Satisfaction Score’ and ‘Accuracy’ provide insights into the stability and quality of agent outcomes over time.
- User experience enhancement:
- Monitoring also evaluates user satisfaction and usability to enhance interaction quality and engagement.
- Continuous improvement:
- Effective monitoring supports ongoing training and adaptation, ensuring AI agents remain efficient in dynamic environments.
- Cost-effectiveness and accuracy tradeoff management:
- AI agent monitoring helps manage the tradeoff between achieving high accuracy and controlling operational costs. Real-time monitoring of model usage and costs supports strategic decisions on resource allocation and operational budgeting, ensuring agents deliver desired performance efficiently.
- Enhanced debugging and error resolution:
- Monitoring the intermediate steps in AI agent processes is essential for debugging complex tasks where early inaccuracies can lead to system-wide failures. The ability to continually test agents against known edge cases and integrate new ones found in production improves the robustness and reliability of AI agents.
- Improved user interaction insights:
- Analyzing how users interact with AI agents provides critical insights that can refine and tailor AI applications to meet user needs better. Capturing user feedback provides a measure of quality over time and across different versions. Additionally, monitoring ‘cost’ metrics enables precise optimizations, enhancing user experience and operational efficiency.
The future of AI agent monitoring: Key trends and enhancements
The field of AI agent monitoring is rapidly evolving, driven by the increasing sophistication of agents and their deeper integration into critical business processes. As organizations move beyond initial implementations, monitoring strategies must mature to ensure sustained performance, reliability, and value alignment. Based on current trajectories and identified needs, we can anticipate several key future trends and enhancements:
- Business-aligned metrics: AI agent monitoring metrics must directly align with business objectives rather than technical performance alone, ensuring AI agents deliver meaningful organizational value. As the AI landscape evolves, there’s an increased focus on developing metrics that assess ethical considerations, transparency, and fairness. These metrics ensure AI systems operate responsibly and do not perpetuate biases, aligning AI operations with emerging ethical standards and regulatory requirements. Moreover, clear outcome targets drive better optimization decisions, shifting focus from process efficiency to result quality.
- Workforce transformation: Human teams must evolve alongside AI technology, developing specialized skills in monitoring, evaluating, and optimizing AI performance.
- Sophisticated outcome evaluation with Human-in-the-Loop: Evaluating whether an agent’s output truly aligns with desired goals or complex requirements often involves subjective judgment that automation alone cannot capture. While automated evaluation metrics will improve, complex or nuanced tasks will necessitate robust human feedback mechanisms integrated directly into monitoring workflows. Expect tools that streamline the capture, aggregation, and analysis of human evaluations (expert reviews, user feedback) to continuously refine agent performance and retrain models based on qualitative assessments, moving beyond simple pass/fail metrics.
- Unified monitoring dashboards: Future iterations will likely centralize all monitoring capabilities in comprehensive dashboards accessible to all stakeholders, eliminating the need to connect with specialists for monitoring insights.
- Enhanced explainability and interpretability: Knowing that an agent failed is insufficient; understanding why is critical, especially as agent workflows become more complex. Monitoring platforms will incorporate more advanced explainability features, visualizing the agent’s decision-making process, tracing data flow through intricate workflows, and pinpointing the exact source of errors or unexpected behavior. Explainability and interpretability in AI metrics are becoming essential as organizations strive to enhance trust and allow for better oversight. Implementing metrics that measure the transparency of AI processes helps ensure that AI agents are understandable and accountable, which is critical as AI decision-making becomes more integrated into business operations.
- Integrated time-based alerting: Generative AI platforms will likely expand their capabilities to automatically flag when steps consistently exceed expected execution times, allowing for proactive workflow optimization.
- Leveraging comprehensive monitoring solutions: Integrating advanced observability platforms with internal monitoring tools is a growing trend in AI agent management. This approach provides a comprehensive view of AI operations, combining internal performance metrics with external insights to ensure every component performs optimally, from service calls to data handling. This strategy leverages the strengths of both sets of tools to enhance the overall monitoring and management of AI agents.
- Standardization of AI metrics: There are ongoing initiatives to standardize AI agent metrics, facilitating better comparison across systems and promoting best practices. Standardized metrics allow organizations to align on performance expectations and benchmarks, fostering collaboration and advancing the field.
Endnote
As AI agents become central to enterprise operations, monitoring their performance is no longer a technical afterthought but a business-critical function. These agents operate in dynamic environments, where their behavior can shift based on input complexity, model drift, and system dependencies. Organizations risk poor outcomes, compliance issues, and missed optimization opportunities without robust monitoring of AI agents.
Effective monitoring hinges on well-defined, multidimensional metrics. From token usage and latency to instruction adherence and user satisfaction, these metrics form the foundation for evaluating agent efficiency, reliability, and business impact. They help teams detect anomalies early, fine-tune agent behavior, and continuously improve performance at scale.
ZBrain transforms this challenge into a streamlined, insight-driven process. It’s a comprehensive platform that provides performance dashboards and key insights that offer end-to-end visibility into every AI agent. By unifying technical data, user feedback, and cost metrics, ZBrain empowers organizations to track agent activity and understand it, optimize it, and align it with evolving business goals.
In the future of AI-driven operations, organizations that adopt structured monitoring, apply meaningful metrics, and leverage platforms like ZBrain will be positioned to scale confidently—knowing their AI agents are functional, trustworthy, efficient, and strategically valuable.
Ready to unlock the full potential of AI agents? Start building, deploying, and monitoring enterprise-grade AI agents with ZBrain. Gain real-time visibility into performance, costs, and outcomes——all within a single, unified dashboard.
Listen to the article
Author’s Bio
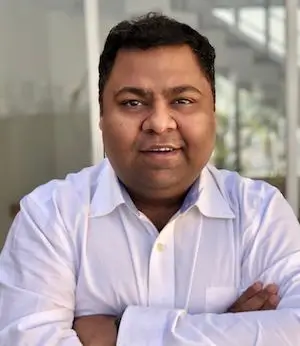
An early adopter of emerging technologies, Akash leads innovation in AI, driving transformative solutions that enhance business operations. With his entrepreneurial spirit, technical acumen and passion for AI, Akash continues to explore new horizons, empowering businesses with solutions that enable seamless automation, intelligent decision-making, and next-generation digital experiences.
Table of content
- Why monitoring AI agents is essential?
- Understanding ZBrain metrics for agent monitoring
- Post-deployment monitoring and effective management of ZBrain AI agents
- Best practices for monitoring AI agents
- Key benefits of monitoring AI agents
- The future of AI agent monitoring: Key trends and enhancements
What is AI agent monitoring and why is it essential?
AI agent monitoring involves systematically observing and analyzing the behavior, outputs, and overall performance of AI agents to ensure they function optimally. This practice is crucial because AI agents often handle complex, variable tasks that traditional software isn’t designed for. Monitoring helps maintain reliability, ensures compliance with various standards, and optimizes operational efficiency. It also allows businesses to respond proactively to performance anomalies and security vulnerabilities, thus safeguarding both the technology and the data it processes.
How does AI agent monitoring differ from traditional software monitoring?
Unlike traditional software that performs predictable, static functions, AI agents are dynamic and can learn from new data, making their behavior less predictable. AI agent monitoring therefore goes beyond checking for system uptime or bug reports; it includes evaluating decision-making processes, adaptation to new data, and adherence to specific instructions and guidelines. Furthermore, the monitoring of AI agents often requires more granular data about decisions and actions, which necessitates the use of sophisticated analytical tools to interpret the complex data these systems generate.
What are the key metrics for monitoring AI agents?
Effective AI agent monitoring utilizes a range of metrics such as accuracy, response times, satisfaction scores, and more nuanced measures like instruction adherence and context window utilization. These metrics provide a multidimensional view of an agent’s performance, from technical efficiency to impact on end-users, helping organizations optimize both the agent’s functionality and its alignment with business goals. This comprehensive metric tracking is vital for ensuring that AI agents remain reliable and effective over time, adapting to new conditions and user needs without compromising their integrity or performance.
How does monitoring improve the management of AI agents?
Monitoring provides critical insights that can improve the accuracy and efficiency of AI agents by identifying and correcting errors, optimizing resource use, and ensuring that the agents adapt properly over time. It also helps in refining the agents based on real-world feedback, ensuring that they continue to meet organizational needs and comply with regulatory standards. By having a continuous loop of feedback and adjustment, organizations can enhance agent capabilities and ensure seamless integration into various business processes.
How does ZBrain enhance the real-time monitoring of AI agents?
ZBrain’s platform provides various real-time monitoring tools specifically designed for AI agents. It also includes detailed dashboards that track key performance indicators such as response times, accuracy, and user satisfaction. These features enable users to detect performance anomalies and inefficiencies as they occur, allowing for immediate corrective actions.
How does ZBrain support the operational management of AI agents post-deployment?
ZBrain simplifies the post-deployment management of AI agents through its comprehensive monitoring tools and customizable dashboards. It provides:
-
Performance Monitoring: Enables continuous tracking of key performance indicators to ensure agents operate within expected parameters.
-
Activity Logs and Reports: Offers in-depth analysis of agent operations through detailed logs and performance reports, helping identify any issues.
-
Agent Dashboard: Centralizes control and visibility, allowing users to quickly assess the health and efficiency of each agent and make adjustments as needed.
-
Feedback Mechanisms: Incorporates user feedback directly into the performance loop, enabling continuous improvement based on real-world usage and interactions.
These tools and metrics help organizations maintain high standards of performance and adapt quickly to changing operational needs, ensuring that AI agents continue to add value to business processes effectively.
What are the key benefits of monitoring AI agents?
Monitoring AI agents offers crucial performance insights, tracking metrics like accuracy and response times to ensure optimal efficiency. It facilitates cost management through efficiency optimization and enhances reliability tracking to ensure consistent performance. Additionally, monitoring refines user interactions and supports continuous improvement by adapting AI behavior based on user feedback and operational data, ultimately enhancing user satisfaction and operational efficiency.
How can organizations get started with building AI solutions using ZBrain?
To initiate AI development with ZBrain, organizations should contact ZBrain via email at hello@zbrain.ai or through the inquiry form on their website at http://zbrain.ai. The ZBrain team will assess your current AI infrastructure and needs, and help design a customized strategy that includes setup, integration, and comprehensive support to ensure successful implementation and maximization of AI capabilities within your business operations.
Insights
How to build a search-optimized knowledge repository with ZBrain
ZBrain’s advanced knowledge base is engineered to scale with your enterprise needs, whether you’re handling a few thousand records or millions of documents, without compromising performance or uptime.
How ZBrain enhances knowledge retrieval with intelligent reranking
ZBrain significantly enhances the relevance of enterprise search results through reranking, acting as an intelligent gatekeeper between user queries and corporate data.
How ZBrain’s multi-agent collaboration works
ZBrain’s multi-agent collaboration is an advanced approach in which multiple specialized AI agents work together as a cohesive team to solve complex enterprise challenges.
ZBrain’s modular stack for custom AI solutions
ZBrain Builder’s modular architecture – comprising data ingestion pipelines, a knowledge base, an LLM orchestration layer, automation agents, and integration-ready interfaces – serves as a set of building blocks for enterprise AI solutions.
AI in project and capital expenditure management
Adopting AI across the capital expenditure (CapEx) lifecycle can radically improve how organizations plan, execute, and evaluate big investments.
AI for plan-to-results
The integration of AI into plan-to-results operations is transforming how organizations align strategic planning with execution to optimize business performance.
AI for accounts payable and receivable
The integration of AI in AP and AR operations extends beyond routine automation, offering strategic insights that empower finance professionals to evolve from reactive problem-solvers into proactive strategists.
AI for HR planning and strategy
Integrating AI into HR planning and strategy is revolutionizing how organizations manage their workforce, optimize talent, and align HR initiatives with business goals.
AI in quote management
AI is redefining quote management by automating complex processes, improving pricing accuracy, and accelerating approval workflows.