Building blocks of AI: ZBrain’s modular stack for custom AI solutions
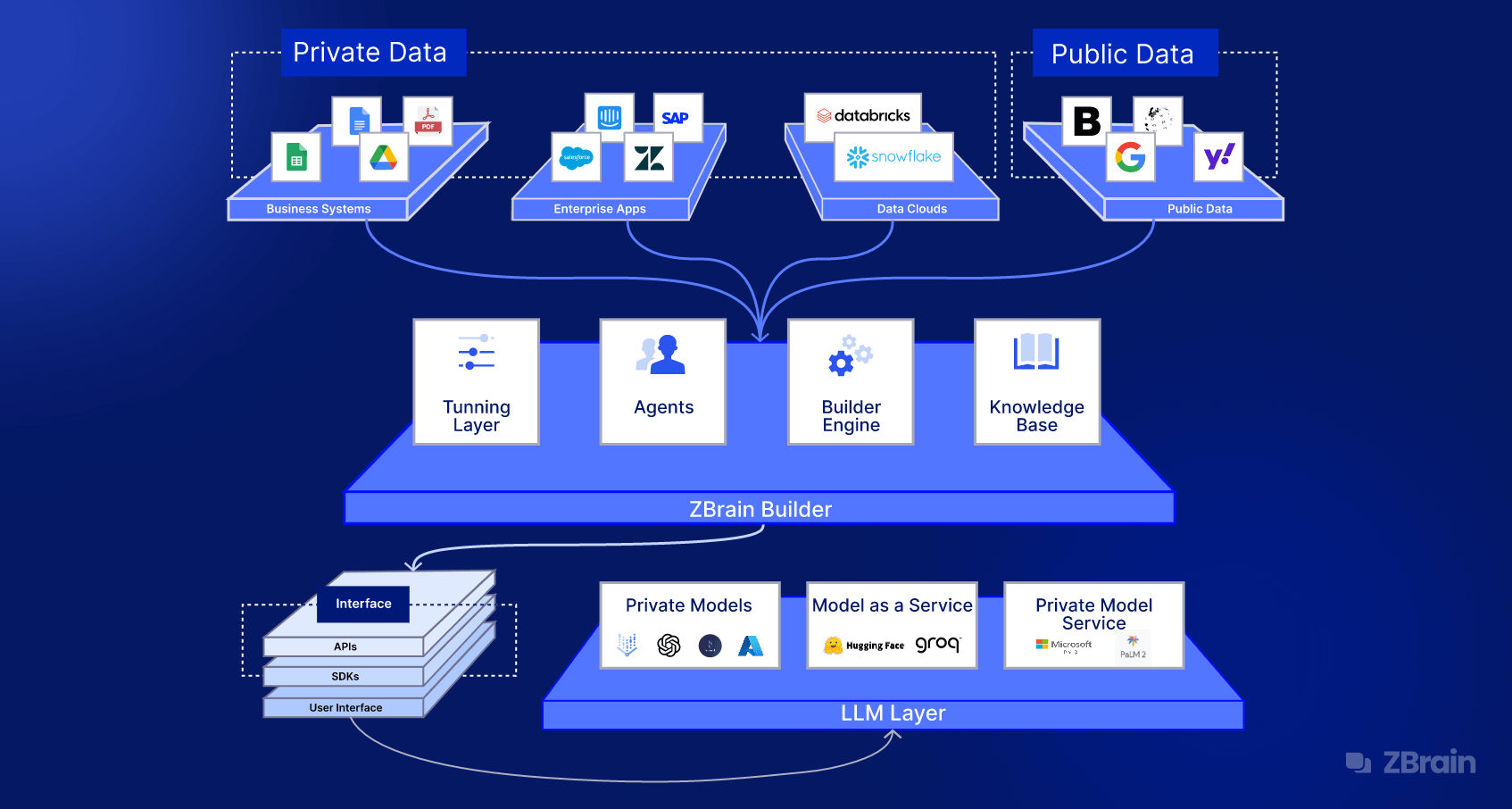
Listen to the article
In the contemporary digital era, enterprises increasingly invest in artificial intelligence (AI) to maintain competitiveness and foster innovation. A recent McKinsey report indicates that by 2028, 92% of companies plan to boost their AI investments. However, despite this substantial commitment, only 1% of business leaders consider their organizations to be at a mature stage of AI deployment, where AI is fully integrated into workflows and delivers significant business outcomes.
This disparity underscores the challenges enterprises face in effectively adopting AI. Integrating AI into existing systems often encounters obstacles such as legacy infrastructure limitations, data silos, and a shortage of skilled personnel. Additionally, concerns about vendor lock-in, interoperability, and stringent regulatory compliance further complicate the AI adoption process.
Adopting a modular AI approach can be advantageous to navigate these challenges. A comprehensive GenAI orchestration platform like ZBrain offers a flexible modular architecture that enables organizations to mix and match components and customize every layer, from data ingestion and knowledge management to model orchestration and user interface. This design not only facilitates seamless integration with existing infrastructures but also promotes scalability, continuous innovation, and operational efficiency.
This article delves into the strategic benefits of such a modular AI approach. By leveraging a composable architecture, enterprises can tailor each component of their AI platform to meet specific needs while maintaining a cohesive, enterprise-grade solution. This methodology mitigates the risks associated with traditional, monolithic deployments and ensures that technology investments align with long-term business objectives and a competitive market vision.
- Navigating the challenges enterprises face in AI adoption
- What are the strategic benefits of modular architecture for enterprise AI?
- Introducing ZBrain: A comprehensive enterprise AI platform
- How does ZBrain Builder's modular architecture enable the building of flexible, scalable, and vendor-agnostic AI solutions?
- Customization and extensibility: Tailoring ZBrain to your enterprise needs
- Best practices for ZBrain: Security, performance, and governance
Navigating the challenges enterprises face in AI adoption
For enterprises, deploying AI isn’t just a technological upgrade—it’s a strategic transformation that must integrate seamlessly with your existing infrastructure while driving innovation. This section outlines the key challenges enterprises may face when adopting AI at an enterprise scale. From integrating with legacy systems and managing vast data volumes to mitigating vendor lock-in and ensuring robust data security and regulatory compliance, understanding these hurdles is crucial. By addressing these challenges head-on, enterprises can build a resilient, scalable AI framework that aligns with their business objectives and supports long-term growth.
Here are the challenges:
Technical complexity and integration
-
Legacy system integration
Your organization’s established IT ecosystem may not be designed for the data-intensive, computationally heavy workloads that modern AI requires. Integrating AI with legacy systems often means rethinking workflows and potentially overhauling core data architectures. This not only affects short-term deployment timelines but also influences long-term scalability and maintainability. -
Skill and expertise gap
Developing and operationalizing AI solutions requires specialized skills that are often scarce. Enterprises must balance investing in upskilling internal teams or partnering with external experts. This challenge isn’t just technical—it’s also a critical resource planning and talent management issue.
Scalability and infrastructure demands
-
Infrastructure investment
AI workloads demand robust, high-performance computing resources such as GPUs, TPUs, and scalable storage. Balancing on-premise infrastructure with cloud-based solutions is key. The right mix enables you to scale efficiently without incurring prohibitive costs, ensuring your AI systems can grow with the business. -
Data management and silos
Large enterprises often grapple with fragmented data silos and inconsistent data quality. For AI to be effective, you need a comprehensive data governance strategy that not only consolidates disparate data sources but also ensures ongoing data accuracy and accessibility. This is crucial for developing reliable, scalable AI solutions.
Vendor lock-in and interoperability
-
Proprietary ecosystems
Many turnkey AI platforms lock you into a specific vendor’s ecosystem. This limits flexibility and may complicate future technology integrations or migrations. Enterprises should prioritize solutions with open architectures and interoperability standards to maintain long-term agility. -
Strategic independence
Mitigating vendor lock-in is not just about technology—it’s a strategic decision that impacts your organization’s innovation trajectory and negotiation leverage with suppliers. A hybrid or multi-cloud strategy can provide the flexibility you need to avoid being tied to a single provider.
Data privacy, security, and compliance
-
Regulatory demands:
AI systems inherently process vast amounts of data, including sensitive information. Ensuring compliance with regulations such as SOC 2 is non-negotiable. Enterprises must implement robust data security measures—like access controls, and continuous monitoring—to mitigate risks and build stakeholder trust. -
Ethical and transparency concerns
The “black box” nature of some AI models can create accountability and trust issues. Transparent AI practices—including explainable AI and rigorous validation protocols ensure that AI systems deliver reliable and ethically sound outcomes.
Transitioning from these key challenges, it’s clear that overcoming integration hurdles, scalability issues, vendor lock-in, and data governance concerns is critical for enterprise AI success. To address these pain points effectively, enterprises must rethink their approach to AI implementation.
Here comes the need for a modular AI stack. A modular strategy offers a flexible, scalable framework that can seamlessly integrate with existing systems, enabling you to mitigate the challenges discussed while aligning with long-term business objectives. By adopting a modular AI stack, your enterprise can achieve greater agility, reduce dependency on single vendors, and build a more robust, adaptable AI infrastructure for the future.
What are the strategic benefits of modular architecture for enterprise AI?
Adopting a modular architecture is a game-changer in AI development, offering a transformative approach to overcoming integration hurdles, scalability issues, vendor dependencies, and data governance challenges. In this section, we explore how building AI systems with composable components enables seamless integration with existing infrastructure, dynamic scaling, improved security, and continuous innovation. We’ll delve into the technical and strategic benefits that make modular design essential for building robust, future-ready AI solutions.
Composable architecture for flexibility and integration
Plug-and-play modularity for rapid deployment:
ZBrain’s architecture is designed with true modularity in mind, allowing users to configure and customize pipelines without touching the core infrastructure. Each module—whether it’s data connectors, preprocessing layers, vector storage, or LLM orchestration—can be assembled like building blocks via a low-code interface, enabling rapid solution development.
This composability:
-
Enables fast iteration and experimentation:
-
Users can replace or tweak specific modules (e.g., swapping out a data source or changing the embedding model) without impacting the end-to-end pipeline. This reduces iteration cycles and allows teams to respond faster to evolving requirements.
Scalable architecture for evolving enterprise AI needs
Scale across teams, workflows, and use cases:
ZBrain is built with scalability at its core, enabling enterprises to deploy and manage AI agents across varied departments, use cases, and data environments—all from a single platform. Its modular and composable architecture ensures that scalability is not just vertical (adding more compute) but horizontal (expanding use cases, users, and integrations without friction).
This scalability:
-
Supports distributed AI agent deployment:
ZBrain allows organizations to deploy multiple AI agents—each fine-tuned for a specific use case—while maintaining a unified control layer. This ensures scalability across business functions without siloed systems. -
Handles increasing data loads efficiently:
With its ability to integrate with both real-time (e.g., Webhooks) and batch data sources (e.g., SQL, CRMs), ZBrain scales to accommodate growing volumes of enterprise data. As data grows, ingestion pipelines and vector indexing mechanisms can be scaled independently. -
Optimized for scalable LLM orchestration:
ZBrain supports the dynamic selection and routing of prompts to multiple LLMs (such as GPT-4 or Claude) based on the task. This abstraction allows enterprises to scale model performance intelligently by choosing the right model per use case. -
Elastic infrastructure compatibility:
The platform can be deployed on scalable infrastructure—cloud-native environments (AWS, GCP, Azure), on-premises, or hybrid setups. This flexibility allows ZBrain to scale alongside enterprise IT requirements without vendor lock-in.
Mitigating vendor lock-in
Interoperability and strategic independence:
One significant pain point for enterprises is the risk of vendor lock-in. Proprietary AI solutions often tie you to specific ecosystems, limiting flexibility.
Modular architecture:
-
Promotes open standards:
By adopting open APIs and containerized microservices, enterprises can integrate components from different vendors or even swap out entire modules as needed. This reduces dependency on any single provider. -
Enables hybrid deployments:
A modular system can seamlessly operate across on-premise, cloud, or hybrid environments. This flexibility allows you to leverage the strengths of different platforms—for example, using cloud services for scalability while retaining critical data processing on-premise for enhanced security.
Enhancing data privacy, security, and compliance
Modular architecture with enterprise-grade controls:
ZBrain’s modular, composable architecture supports robust governance and data protection practices. By isolating workflows across independent applications and layering in certified security frameworks, ZBrain helps enterprises build AI systems that align with stringent privacy and compliance standards.
This modularity:
Enables application-level data isolation:
Each ZBrain application—built from its own pipeline of modules—functions independently. This separation ensures that sensitive data remains isolated within its designated workflow unless explicitly shared.
Allows privacy-conscious component choices:
ZBrain’s modular design enables teams to select different embedding models (OpenAI, Azure OpenAI) and vector databases (Pinecone, Qdrant, Chroma). This flexibility allows enterprises to opt for self-hosted or open-source alternatives when handling sensitive data, helping them minimize external data exposure and align with internal data governance policies.
Streamlined maintenance and continuous improvement
Ease of updates and upgrades:
Maintaining and evolving an AI system is a continuous process. A modular framework allows for:
-
Rapid iteration:
Updates can be rolled out to individual modules without impacting the entire system. This approach enables a continuous delivery model where improvements and security patches are deployed swiftly. -
Improved troubleshooting:
Composable modules simplify monitoring and debugging. If an issue arises, the isolated nature of modules helps in pinpointing the exact area of failure, reducing recovery time and minimizing operational disruption.
Modular architecture addresses the core challenges of enterprise AI by offering flexibility, scalability, vendor independence, and robust security. Embracing modular design enables you to navigate the complexities of AI adoption, ensuring that your infrastructure remains agile, secure, and future-ready.
Optimize Your Operations With AI Agents
Our AI agents streamline your workflows, unlocking new levels of business efficiency!
Introducing ZBrain: A comprehensive enterprise AI platform
ZBrain is an enterprise-grade generative AI platform comprising two major components: ZBrain XPLR and ZBrain Builder. It is designed to facilitate the seamless integration of artificial intelligence into organizational workflows, offering a structured approach to assess AI readiness, identify high-impact opportunities, and develop custom AI solutions that align with strategic business objectives.
ZBrain XPLR: AI readiness and opportunity assessment framework
ZBrain XPLR enables organizations to evaluate their preparedness for AI adoption and pinpoint areas where AI can deliver substantial value. Key features include:
-
Taxonomy explorer: Maps organizational processes across various functions to uncover opportunities for AI-driven transformation.
-
AI effectiveness tool: Assesses the potential impact of AI on different operational areas, facilitating informed decision-making to prioritize initiatives that offer the greatest value.
-
Customizable process flows: Provides visual representations of workflows, tasks, and decision points, aiding in the analysis and optimization of complex business processes.
-
AI Hubble: An AI-powered solution discovery tool that evaluates business workflows to recommend effective AI profiles and solutions for specific tasks.
-
Case studies and educational resources: Offers a comprehensive knowledge hub with educational materials and real-world case studies to support informed decision-making.
-
Repository of use cases: Access to numerous enterprise use cases to kickstart AI initiatives with proven solutions tailored to various industries and functions.
By leveraging ZBrain XPLR, organizations can develop actionable roadmaps for AI implementation, ensuring alignment with strategic objectives and maximizing return on investment.
ZBrain Builder: Enterprise-grade generative AI orchestration platform
ZBrain Builder is a low-code platform that simplifies the creation, deployment, and management of custom AI applications and agents. Its key features include:
-
Efficient data ingestion and processing: Collects data from both private and public sources, processing it using an ETL (Extract, Transform, Load) workflow to convert it into a usable format for seamless storage in the knowledge base.
-
Build apps and AI agents: Enables the creation of enterprise-grade AI solutions that automate tasks, streamline workflows, and enhance productivity.
ZBrain Builder empowers organizations to leverage their proprietary data securely, facilitating the development of AI solutions that align with specific business needs and integrate seamlessly with existing systems.
By leveraging ZBrain XPLR and ZBrain Builder, organizations can strategically assess their AI readiness, identify valuable opportunities, and implement tailored AI solutions that drive innovation and efficiency.
How does ZBrain Builder’s modular architecture enable the building of flexible, scalable, and vendor-agnostic AI solutions?
Enterprises today demand AI platforms that can adapt to their unique needs—from data handling to model selection—without requiring a complete rebuild for each use case. ZBrain addresses this by offering a modular AI architecture that is both composable and flexible, enabling organizations to mix and match components and customize each layer of the stack. The result is an enterprise-grade AI platform where every layer (from data ingestion and knowledge management to model orchestration and user interface) can be tailored or swapped out as needed to build custom solutions. Below is a technical overview of ZBrain’s modular architecture and how its building blocks work together to support adaptable AI solutions for the enterprise.
ZBrain is a full-stack generative AI orchestration platform built with a modular design. In practice, this means each major component of the platform operates as an independent layer that can integrate with or be replaced by equivalent technologies. This modularity ensures enterprises have flexibility in deployment and integration, scaling each part of the system as needed without disrupting others.
At a high level, ZBrain’s architecture comprises the following key modules (or “building blocks”):
- Data integration and ingestion – Connectors and pipelines to ingest data from various sources (documents, databases, APIs, etc.) and preprocess it for AI use.
- Knowledge base – A centralized repository (often a vector database) where ingested data is stored as embeddings or indexed information for efficient retrieval.
- LLM orchestration layer – The interface to large language models (LLMs), which is model-agnostic and can route queries to different AI models as needed.
- Orchestration engine and agents – The “brain” of the platform that defines the logic and workflows (via a low-code builder) and executes multi-step AI agents or automation.
- User interface and integration layer – APIs, SDKs, and UI components that allow end-users or external applications to interact with the AI (for example, through chat interfaces or integrations into tools like Slack or a CRM).
Each of these layers interacts through well-defined interfaces. For example, an AI agent can retrieve context from the knowledge base, call an LLM via the orchestration layer, and then return results through an API to a business application. Because components are composable, organizations can independently develop, extend, or replace each module (for instance, swapping out the vector store or choosing a different LLM) without overhauling the entire system.
In the sections below, we dive into each module in detail and explain how they contribute to ZBrain’s flexible stack.
Data integration and ingestion
ZBrain’s data ingestion module is designed to seamlessly connect to diverse enterprise data sources and funnel real-time information into your AI system. Its core strength lies in its extensive library of pre-built, plug-and-play connectors that enable rapid integration with common platforms—such as SharePoint, Google Drive, OneDrive, Salesforce, and many others—eliminating the need for custom ETL code.
This module supports both continuous and scheduled ingestion from APIs, event streams (e.g., Kafka), and live databases. With features like automatic syncing and update triggers, ZBrain ensures that your AI models are consistently fed the latest data from new transactions and support tickets, keeping insights timely and relevant.
Data is ingested in a variety of formats, including structured formats (CSV, JSON) and unstructured documents (PDFs, Word docs, emails). Once data is ingested, ZBrain performs a robust ETL (Extract, Transform, Load) process. It extracts text from different file types using integrations with OCR and document processing tools like AWS Textract or Google Document AI. The system then cleans, transforms, and splits the content into manageable, semantically coherent chunks, each tagged with metadata (such as source, title, etc.) to preserve context and ensure that both structured and unstructured data are converted into a unified, queryable format.
Enterprise connectivity and security are paramount at this layer. Data from internal systems (like Salesforce, SAP, and Jira) is ingested securely, with strict access controls and compliance measures ensuring that sensitive information remains within the company’s controlled environment. This comprehensive approach unifies both private enterprise data and public data into a single knowledge base, laying a robust foundation for effective AI processing.
Challenges enterprises face in data integration and how ZBrain’s data layer addresses them
Enterprise Challenge | How ZBrain Addresses It | Enterprise Benefit |
---|---|---|
Diverse Data Sources & Integration Complexity | Provides an extensive library of pre-built, plug-and-play connectors for platforms such as SharePoint, Google Drive, OneDrive, Salesforce, etc., eliminating the need for custom ETL code. | Rapid, hassle-free integration of data from multiple sources. |
Real-time Data Availability | Supports both continuous and scheduled ingestion from APIs, event streams (e.g., Kafka), and live databases, complete with automatic syncing and update triggers. | Ensures AI models are consistently fed with the most up-to-date information for timely insights. |
Data Heterogeneity | Ingests various data formats—structured (CSV, JSON) and unstructured (PDFs, Word docs, emails)—and employs a robust ETL process to extract, clean, transform, and split data into unified, queryable chunks with metadata. | Comprehensive coverage and improved quality of data, making it ready for efficient AI processing. |
Security & Compliance Requirements | Ingests data securely from internal systems like Salesforce, SAP, and Jira, enforcing strict access controls and compliance measures. | Protects sensitive information and ensures adherence to regulatory and data residency requirements. |
Scalability with Increasing Data Volumes | Utilizes a modular design that enables independent scaling of data ingestion pipelines to handle growing data volumes without compromising overall performance. | Maintains consistent performance as data loads increase, supporting enterprise growth. |
Streamlined Data Processing | Uses a robust ETL process that automatically extracts text via integrations with OCR and document processing tools (e.g., AWS Textract, Google Document AI), then cleans, transforms, and organizes the data with appropriate metadata. | Converts diverse data into a coherent, queryable format for effective AI processing and improved decision-making. |
Knowledge base
The knowledge base is the core of ZBrain’s modular stack, acting as a central knowledge repository that all AI applications and agents draw upon. Once data is ingested and processed into chunks, it is stored in the knowledge base for fast retrieval. ZBrain’s knowledge base is designed to be extremely flexible and scalable, supporting multiple backend storage options and retrieval strategies. It supports seamless data ingestion from multiple sources and formats and serves as the foundation for building LLM-based applications.
- Storage and indexing: Under the hood, the knowledge base uses vector databases and other indexing techniques to store embeddings of the data for similarity search. Importantly, ZBrain is storage-agnostic – it supports various vector stores and does not lock the user into a single provider. Enterprises can choose a managed vector DB service like Pinecone for scalable indexing, or use ZBrain’s built-in vector store for a more cost-effective in-house solution.
This modular approach means a company could swap out the vector database if needed (e.g., migrate to a different database technology) without changing how the rest of the system operates. Additionally, ZBrain can index content in traditional ways if needed (for example, full-text search indexes), enabling hybrid search capabilities (combining semantic vector search with keyword search).
- Retrieval and query engine: The knowledge base module implements advanced retrieval techniques so that AI agents can fetch relevant information efficiently. When an AI query comes in, ZBrain can perform a vector similarity search against the stored embeddings to find relevant pieces of text/data that pertain to the query. This retrieval can be tuned with configurable parameters like Top-K (how many results to retrieve) and confidence score thresholds. ZBrain’s platform even automatically optimizes retrieval methods for performance while also allowing strategy customization.
In practice, this means the system might intelligently choose whether to use pure vector search, keyword search, or a combination, based on what yields the best results for a given knowledge base – yet users can override settings or select their preferred approach.
All these features ensure that the knowledge base isn’t just a dumb data store, but can optimize information for LLM consumption.
- Security and privacy: Since the knowledge base holds potentially sensitive enterprise data, ZBrain includes enterprise-grade security at this layer. Data stored to the knowledge base is governed by role-based access controls and user permissions managed by the ZBrain Engine’s governance features. Moreover, if an organization deploys ZBrain in a private cloud or on-premises, the knowledge base resides entirely within their secure environment.
This allows companies in regulated sectors (finance, healthcare, etc.) to use ZBrain while keeping data compliant with their policies – for instance, using private S3 storage for vector data so that nothing leaves their AWS account.
- Automated reasoning: ZBrain’s Automated Reasoning feature enriches the knowledge base by automatically extracting key rules and variables to underpin intelligent query processing. Through a policy-driven approach, users can define a reasoning model with tailored prompts, allowing the system to interpret and apply embedded conditions from the ingested data. This mechanism not only identifies critical data attributes and relationships but also empowers users to test and refine reasoning logic in an interactive playground. The result is a robust, context-aware engine that delivers precise, data-driven responses, ultimately enhancing decision-making and operational efficiency.
In summary, the knowledge base module supplies the contextual memory for ZBrain’s AI solutions. By being able to ingest diverse data, store it in a pluggable vector database, and retrieve it intelligently, this module enables retrieval-augmented generation workflows where LLMs can draw on up-to-date, company-specific information rather than just their static training data. It’s a key reason ZBrain can deliver accurate, context-specific responses in enterprise applications.
Enterprise challenges addressed by ZBrain’s knowledge base and how the solution tackles each issue
Enterprise Challenge | How ZBrain’s Knowledge Base Addresses It | Enterprise Benefit |
---|---|---|
Diverse Data Integration | Ingests data from multiple sources and formats (structured and unstructured) and unifies it through a robust ETL process. Uses pluggable vector databases and supports hybrid indexing (vector & keyword search). | Ensures comprehensive, consistent, and queryable data storage for seamless AI processing. |
Scalability & Flexibility | Designed with a modular, storage-agnostic architecture that supports various backend storage options and allows for easy swapping of vector databases without disrupting the overall system. | Enables organizations to adapt and expand their data infrastructure as data volumes and business requirements grow. |
Efficient Data Retrieval | Implements advanced retrieval techniques such as vector similarity search with configurable parameters (e.g., Top-K, confidence thresholds) and allows users to customize the search strategy (vector, keyword, or hybrid). | Facilitates fast and relevant retrieval of information, ensuring that AI models can access the most pertinent, up-to-date data. |
Security & Compliance | Incorporates enterprise-grade security measures, including role-based access controls, and the option for private or on-premises deployment to keep sensitive data secure. | Ensures data integrity, regulatory compliance, and protection of sensitive enterprise information. |
LLM orchestration layer
At the heart of any generative AI platform is the connection to Large Language Models (LLMs) and other AI models. ZBrain’s architecture takes a model-agnostic approach to LLM integration, providing a flexible orchestration layer that can work with different AI models and even multiple models in tandem. The LLM orchestration layer abstracts away the details of any given model provider, offering a uniform interface to the rest of the system. This design lets enterprises plug in the models of their choice – whether they are public API-based models or custom models – and even use several types of models concurrently.
- Multiple model support: ZBrain supports most of the leading AI model providers and frameworks out of the box. For instance, it can integrate with OpenAI’s GPT series (via API), Google’s PaLM models (through Vertex AI), Anthropic’s Claude, Amazon Bedrock’s model suite, and Azure’s hosted OpenAI service. It also supports open-source models (like Meta’s LLaMA or other community models) and even specialized models that a company might bring (for example, a fine-tuned domain-specific model). ZBrain refers to this as supporting both public and private models, meaning you can use third-party hosted models or your own proprietary ones within the same platform. In practice, a ZBrain app might use GPT-4 for one task and an internal model for another, all coordinated through the same orchestration layer.
- Intelligent routing and model selection: Because it’s common for enterprises to have different models suited to different jobs (e.g., a smaller, faster model for simple queries and a larger, more powerful model for complex tasks), ZBrain’s LLM layer includes intelligent routing capabilities. The platform can route a request to the most appropriate model based on factors like the query complexity, the content domain considerations. This dynamic switching is transparent to the user and can significantly optimize both performance and cost.
- Orchestration of multi-step reasoning: Beyond just selecting which model to call, ZBrain’s orchestration layer can manage complex interactions with LLMs. The system supports advanced prompting techniques and multi-turn dialogues as part of workflows. For instance, it can implement chain-of-thought prompting, self-reflection, or automatic prompt engineering strategies to boost accuracy. These techniques might involve multiple calls to LLMs: one to break down a problem, another to retrieve knowledge (from the knowledge base), another to formulate an answer, etc. ZBrain’s engine coordinates these behind the scenes, so the LLM layer acts as a hub that can orchestrate sequences like “first use a smaller model to classify the query, then use a larger model to generate a detailed answer using retrieved context, and finally run the answer through a moderation model.” All such patterns are configurable without altering the core architecture.
- Abstraction and interchangeability: The benefit of ZBrain’s LLM layer abstraction is that enterprises retain control and flexibility. If, at any point, a company decides to switch providers (say from one cloud’s LLM service to another or to an on-prem model for data residency reasons), they can do so with minimal change. The rest of the application doesn’t need to know which model is being used – it just asks the LLM layer for an answer. This is especially useful in enterprise settings where AI services might be evaluated for compliance or cost, and the ability to change out models (or use multiple to avoid vendor lock-in) is crucial. ZBrain even allows “Model-as-a-Service” integration with platforms like HuggingFace or Groq, indicating it can incorporate hosted custom models or even specialized AI hardware accelerators as needed.
In essence, the LLM orchestration layer ensures that ZBrain is future-proof and customizable on the model front. As new models emerge or enterprise preferences change, the platform can accommodate those changes readily. It separates the “brains” (LLMs) from the rest of the application logic, which is a cornerstone of the platform’s modular philosophy.
Enterprise challenges addressed by ZBrain’s LLM orchestration layer
Enterprise Challenge | How ZBrain’s LLM Orchestration Layer Addresses It | Enterprise Benefit |
---|---|---|
Integrating Diverse AI Models | Utilizes a model-agnostic approach that supports integration with various AI model providers (e.g., OpenAI’s GPT, Google’s PaLM, Anthropic’s Claude) as well as open-source and custom models. This allows the platform to work with multiple models concurrently via a uniform interface. | Offers maximum flexibility in selecting the most appropriate model for different tasks without being locked into one vendor. |
Cost and Performance Optimization | Employs intelligent routing and dynamic model selection based on query complexity, content domain, and cost considerations. This allows the system to use high-accuracy models for critical tasks and more cost-effective models for simpler queries. | Optimizes resource utilization while ensuring that performance is aligned with business requirements. |
Complex Multi-step Reasoning | Supports advanced prompting techniques and multi-turn dialogues to orchestrate complex interactions among LLMs (e.g., chain-of-thought prompting, self-reflection, automatic prompt engineering). The orchestration layer coordinates multiple calls to LLMs for different stages of a workflow. | Delivers more accurate, context-aware responses and enhances the overall reliability of AI-driven processes. |
Vendor Lock-in and Interoperability | ZBrain’s model-agnostic framework allows models to be replaced or updated independently without affecting the rest of the system. This enables enterprises to switch between model providers or use multiple models without requiring major system overhauls. | Ensures long-term flexibility and future-proofing by avoiding dependency on a single vendor, while maintaining seamless integration. |
By addressing these challenges through its modular, model-agnostic orchestration layer, ZBrain provides enterprises with a robust, flexible, and efficient framework for integrating and optimizing large language models in their AI applications.
Agents and automation workflows
One of the most powerful aspects of ZBrain is the ability to create AI agents – autonomous AI systems that can perform specific tasks. These agents are at the intersection of business process automation and AI reasoning. In ZBrain, an AI agent is essentially a multi-step workflow that can take inputs, consult the knowledge base, call LLMs, execute logic, and interact with external systems, all to accomplish a particular task or function. Because ZBrain’s architecture is modular, these agents leverage all the previously discussed layers (ingestion, knowledge base, LLMs) and add another layer of orchestration and automation on top.
- Workflow definition (Flows): ZBrain’s “Flow” component enables users to define the logic of an AI agent. Using this, users (developers or even non-developers via a visual UI) can string together a sequence of steps that the agent will execute. Each step in a flow is a modular component – it could be a data retrieval step, an LLM invocation, a conditional branch (if/else logic), a call to an external API or database, or even another sub-agent. The platform comes with a library of pre-built components (or actions) that can be dragged into these flows, covering common operations like sending an email, querying a database, invoking a cloud service, or performing a calculation. For example, one step might use the knowledge base to “retrieve relevant chunks” of text given a query, and the next step might feed those chunks into an LLM prompt for analysis, and a further step might take the LLM’s answer and create a ticket in a project management system via API.
The above workflow illustrates how an AI agent can combine multiple actions: it starts with an input query, extracts elements via some code, converts a PDF to images (utility step), then uses an LLM to extract data. Later in the flow, it retrieves chunks from the knowledge base, uses an LLM step called “Self Reflection,” branches based on whether data was matched, possibly requests new data via an HTTP call, and even performs a Guardrail Check (an example of content moderation or validation step) before finishing. This highly orchestrated logic enables the creation of a dynamic enterprise agent that can interact with real-world systems and data.
- Agents in action: Because these agents can incorporate conditional logic and integration steps, they can handle sophisticated use cases. ZBrain AI agents can provide intelligent automation across various business functions, enabling users to tackle complex tasks with ease. For instance, an agent could be set up to monitor incoming emails, automatically extract key information using an LLM, consult a database for additional context, and then draft a response or take an action (like updating a record or triggering an alert). Another agent might handle customer support chats by retrieving relevant product info from the knowledge base and formulating answers, but if the query is complex or falls outside a confidence threshold, the agent could escalate it (that’s the human-in-the-loop concept). Essentially, agents tie the AI’s cognitive capabilities (LLMs + knowledge) into the day-to-day workflows of the business.
- Continuous learning through real-time feedback loops: ZBrain AI agents are designed with continuous learning capabilities, incorporating human feedback loops that enable them to refine their performance over time. This adaptability ensures that agents remain effective and accurate in dynamic business environments. For instance, the Feedback Summarization Agent utilizes ongoing human feedback to stay responsive to evolving customer needs and organizational objectives.
- Agent-to-agent communication and coordination: ZBrain facilitates seamless communication and collaboration among multiple AI agents, allowing them to share data, update each other, and work together towards coordinated goals efficiently. This inter-agent communication is pivotal in handling complex tasks that require a collaborative approach. For example, ZBrain AI agents can coordinate to automate and optimize various business processes across diverse functions, enhancing overall operational efficiency.
- Deployment, monitoring, and adaptation in production: ZBrain provides robust tools for deploying, monitoring, and managing AI agents in production environments. Users can test agents with sample data to verify functionality, deploy them for live tasks, and continuously monitor performance to ensure effectiveness. The platform’s monitoring dashboard offers insights into agent performance, logs decisions, and provides controls for real-time intervention or updates. This comprehensive management framework ensures that AI agents remain reliable, auditable, and adaptable to evolving business needs.
- Modularity and reuse: Each agent is a self-contained workflow, which means agents can be developed and deployed independently from one another. This is a big advantage for large organizations: one team can build an AI agent for, say, invoice processing, while another builds a different agent for sales analytics, and each can be updated or scaled without affecting the other. If an organization wants to extend an agent’s capability, they can modify its flow or add new steps (for example, add a new data source to consult, or a new action to perform at the end). If they want to reuse components, they can: e.g., the same “Summarize document” LLM step or the same “Send Slack message” step can be used in many agent workflows. ZBrain also provides an Agent Store with pre-built agent templates for common tasks (like a “Regulatory Monitoring Agent” or “Customer Support Agent”), which enterprises can use as a starting point and then customize.
Under the hood, when an agent runs, the ZBrain Engine (discussed in detail in the next section) takes the defined flow and executes each step, coordinating between the knowledge base and LLM layers as needed. The result is a platform that can not only answer questions but also take actions based on AI outputs. This bridges the gap from insight to execution – a critical capability for AI in enterprise automation.
Enterprise challenges addressed by ZBrain’s agents and automation workflows
Enterprise Challenge | How ZBrain’s Agents and Automation Workflows Address It | Enterprise Benefit |
---|---|---|
Complex, Multi-step Processes | Provides a low-code interface to build autonomous AI agents that coordinate multiple steps (e.g., data retrieval, LLM calls, conditional logic, API interactions) into a single workflow. | Simplifies the automation of intricate business processes, reducing manual effort and errors. |
Customization and Flexibility | Leverages a modular design with reusable pre-built components (such as actions for email, database queries, and notifications) that can be easily assembled and customized to fit specific business needs. | Enables tailored automation solutions that adapt to diverse functions across the enterprise. |
Continuous Improvement and Adaptability | Integrates real-time feedback loops and advanced prompting techniques (e.g., chain-of-thought, self-reflection) to refine performance over time, along with support for agent-to-agent communication for coordinated actions. | Ensures AI agents remain effective, learning from feedback to improve accuracy and adapt to changes. |
Monitoring and Management of Deployed Agents | Offers robust deployment, monitoring, and management tools (such as dashboards, performance logs, and real-time intervention controls) to track agent performance and system health. | Enhances reliability, auditability, and operational efficiency in production environments. |
Bridging the Gap from Insight to Action | Orchestrates multi-step reasoning where agents not only provide insights (by processing data and querying LLMs) but also take actions (e.g., creating tickets, sending alerts) based on those insights. | Transforms static insights into dynamic, actionable outputs that drive business results. |
Builder (the orchestration engine)
The ZBrain Builder engine is the implementation and orchestration layer that ties everything together. It refers both to the backend engine that executes workflows and enforces rules, and the front-end low-code builder interface that developers use to design AI applications. Essentially, ZBrain Builder is the “operating system” for your AI agents and applications, handling the heavy lifting of execution, integration, and management, while providing a user-friendly way to create and manage those AI apps.
- Low-code development interface: ZBrain Builder provides an intuitive, visual interface for designing AI solutions. Instead of writing hundreds of lines of code to call APIs, handle data, and manage AI prompts, a user can utilize Builder’s drag-and-drop canvas to define flows (as illustrated earlier). The platform comes with a suite of tools and pre-built modules to expedite development. Builder is the orchestration engine at the heart of the ZBrain ecosystem, offering users an intuitive interface to design, build, and deploy AI-powered solutions. Users can add third-party tools, LLMs, programming logic, helper methods, and the proprietary knowledge base into their workflows, all within a single integrated development environment. This means that building a custom AI agent might be as simple as selecting a few components and drawing connections between them in the Builder UI, greatly reducing the need for traditional programming. Non-technical domain experts can work alongside developers to craft AI solutions, thanks to this approachable design.
- Core orchestration engine: Behind the scenes, the Builder Engine executes the flows and manages the state of applications. It is responsible for business logic execution, data and user governance, and runtime integrations. For example, it will enforce any rules defined (perhaps an enterprise might impose that certain data never be sent to an external model – the engine will ensure compliance by routing that query to a local model). It also manages user access rights and roles, making sure that only authorized components are accessed (governance is built-in at this layer). The runtime integration capability means the engine can connect in real-time with other systems – e.g., fetching live data from a CRM in the middle of an agent’s execution or writing back results to a database – effectively acting as middleware between AI and enterprise IT systems.
The Builder Engine includes many enterprise-grade features out of the box to support production AI applications:
- Pre-built algorithms and functions: Common algorithms (say, for data processing or calculations) are provided so you don’t have to reinvent them in each app. This could include things like text preprocessing functions, data converters, or ML utilities.
- Evaluation and testing suite: There are tools to test and validate AI workflows. ZBrain provides an evaluation suite that can run test cases against your agent to see how it performs, and even continuous automated testing to catch regressions. This is crucial for enterprise confidence, ensuring the AI behaves as expected before wide deployment.
- Guardrails and controls: The platform implements guardrails to prevent or correct undesired outputs. This might include content filters (to catch sensitive or inappropriate content), sanity checks on LLM outputs (to mitigate hallucinations), and the ability to set fallback behaviors (e.g., if the AI is not confident, don’t answer or escalate to a human). ZBrain Builder has features like “Hallucination Detection & Guardrails” and prompt auto-correction mechanisms to keep outputs reliable.
- Human-in-the-loop and feedback: The engine supports incorporating human feedback at key points. For instance, an agent could pause for human approval on a step, or learn from user ratings on answers. ZBrain explicitly allows gathering end-user feedback and using techniques like Reinforcement Learning from Human Feedback (RLHF) to improve model responses over time.
- AppOps (Application Operations): Once AI applications are deployed, the Builder Engine also handles monitoring and maintenance. ZBrain includes AppOps features for continuous background validation of agents, performance monitoring, and proactive issue detection. This means the platform can alert operators if an agent starts failing or if data drift is detected, ensuring high uptime and reliability for the AI solutions.
In summary, the Builder Engine is what makes ZBrain an enterprise-ready AI platform rather than just a collection of AI models. It provides the scaffolding to build, run, and manage AI applications at scale. By combining a user-friendly builder interface with a robust orchestration back-end, ZBrain enables faster development cycles and easier maintenance. ZBrain Builder’s modular and cloud-native architecture allows organizations to scale AI adoption based on business needs, ensuring flexibility and adaptability.
Enterprise challenges addressed by the ZBrain Builder engine:
Enterprise Challenge | How ZBrain Builder Engine Addresses It | Enterprise Benefit |
---|---|---|
Complex AI Application Development | Provides a low-code, drag-and-drop interface with pre-built modules, algorithms, and integration of third-party tools, enabling users to design and deploy AI applications without extensive coding. | Accelerates development cycles and reduces reliance on heavy coding, resulting in faster time-to-market. |
Integration & Governance with Existing Systems | Acts as a centralized orchestration engine that manages runtime integrations, enforces business logic, and implements role-based access controls, ensuring seamless connectivity between AI workflows and enterprise IT systems. | Enhances interoperability, maintains robust data governance, and secures the overall system environment. |
Ensuring AI Output Quality and Reliability | Includes an evaluation and testing suite that runs automated test cases, implements guardrails (e.g., content filters, sanity checks), and supports fallback mechanisms, along with continuous monitoring of AI workflows. | Minimizes errors, ensures compliance, and improves confidence in the quality and reliability of AI outputs. |
Ongoing Maintenance & Operational Stability | Features AppOps capabilities for continuous background validation, performance monitoring, and proactive issue detection, allowing real-time intervention when necessary. | Reduces downtime, streamlines maintenance, and ensures consistent operational performance in production. |
Customization & Scalability of AI Solutions | Employs a modular architecture that allows individual components to be added, removed, or scaled independently, and enables reusability across different projects. | Provides flexibility to adapt to evolving business requirements, lowers development costs, and supports scalable growth. |
User interface and integration layer
No AI solution is complete without a way for end-users or external systems to interact with it. ZBrain’s top-most layer is the interface and integration layer, which exposes the AI capabilities to users via APIs, SDKs, and UI components. The platform recognizes that enterprises may want to embed AI into their existing applications or create new user-facing applications powered by ZBrain. Thus, this layer provides multiple options to integrate AI outputs into business workflows and end-user experiences.
Key aspects of the interface layer include:
- APIs: ZBrain exposes RESTful APIs that allow external applications to send queries to ZBrain agents or apps and receive results. This makes it straightforward to incorporate ZBrain’s AI into any environment that can make HTTP calls. For example, an enterprise could use the API to connect their internal CRM system with a ZBrain agent, so that a salesperson clicking a “Generate Proposal” button in the CRM triggers an AI workflow in ZBrain and gets back a draft proposal. The documentation highlights APIs as programmatic interfaces that enable the smooth integration of ZBrain with other enterprise systems.
- SDKs: For developers who want a more native integration, ZBrain offers software development kits. These SDKs (available in various programming languages) wrap the API and provide convenient libraries to interact with ZBrain from custom applications. Using the SDK, a developer can authenticate and invoke ZBrain functions directly in their code with minimal boilerplate. This is useful for embedding AI capabilities into complex enterprise software or mobile apps.
- Agent UI: The ZBrain agent interface is a user-friendly interface that enables interaction with AI agents through structured input fields and file uploads. Users can submit inputs such as text or documents in formats like PDF, TXT, CSV, JSON, DOCX, PPTX, and XLSX, and receive contextually relevant AI-generated outputs. Through the interface, users also gain access to API integration capabilities, which allow them to embed ZBrain agents directly into their own applications or workflows. Additionally, a built-in performance dashboard displays key usage metrics such as utilized time, average session time, satisfaction score, and tokens used, offering valuable insights into agent performance.
- Integrations with collaboration tools: Recognizing that a lot of enterprise knowledge work happens in tools like Slack or Microsoft Teams, ZBrain provides direct integrations for these platforms. For example, it has the ability to integrate AI agents into Slack channels or MS Teams chats. This means an employee could interact with a ZBrain agent by messaging a Slack bot, asking questions, and getting answers or summaries right within their chat application. Such integrations greatly drive adoption, since users can access AI help in the tools they already use daily, without switching context.
All these interface options underscore the composability of ZBrain’s solutions. A single AI agent created in ZBrain could be deployed in multiple ways: as a web app, as a chat assistant in Slack, invoked via API by a scheduled job, or embedded in a mobile app via the SDK. The interface layer cleanly separates the back-end logic from the front-end delivery. It also simplifies embedding ZBrain into existing enterprise workflows – rather than forcing users to come to a new application, ZBrain can be brought to where the users are (intranet, BI tools, messaging apps, etc.).
In the context of integration, it’s worth noting that ZBrain supports private deployment models, which complement the interface layer for security. For example, an enterprise can deploy ZBrain within its own AWS or Azure cloud, and then use the APIs internally without traffic ever leaving its network. This means the interface layer can be used in a secure, private manner appropriate for enterprise IT policies (e.g., using internal load balancers, VPNs, etc., to access the AI services).
To sum up, the Interface/Integration layer of ZBrain ensures that the powerful AI and automation capabilities of the platform are accessible to end-users and other software. Whether through a custom dashboard, a chat interface, or a simple API call, ZBrain’s modular stack cleanly surfaces its functionality, making it easy for enterprises to integrate AI into existing systems with minimal disruption.
Enterprise challenges addressed by ZBrain’s user interface and integration layer:
Enterprise Challenge | How ZBrain Addresses It | Enterprise Benefit |
---|---|---|
Integrating AI with Existing Systems | Exposes RESTful APIs and offers SDKs that enable seamless connection with internal systems (e.g., CRM, ERP) and external applications. | Facilitates smooth integration of AI capabilities into current workflows without major overhauls. |
Ensuring User-friendly Interface | Offers a user-friendly interface with structured inputs, multi-format file uploads, and embeddable components like chat interfaces and dashboards. Built-in metrics enhance transparency and user engagement. | Boosts user engagement with intuitive design and real-time performance insights. |
Enabling Multi-channel Deployment | Supports various deployment options including web apps, mobile integrations, and chat assistants (integrated with collaboration tools like Slack and Microsoft Teams). | Broadens access to AI capabilities across multiple channels, increasing operational flexibility. |
Maintaining Security and Compliance | Allows private deployment on enterprise clouds (AWS, Azure, etc.) with secure access mechanisms (VPNs, internal load balancers) and stringent data isolation protocols. | Ensures data security and regulatory compliance, protecting sensitive enterprise information. |
Simplifying Integration and Customization | ZBrain simplifies integration through ready-to-use API endpoints, code snippets, and support for various data formats, enabling seamless embedding into external applications. Customization is made easy with a low-code interface, modular components, and flexible configuration of models, tools, and workflows. | Potential improvement in operational efficiency and reduction in development time through easy customization and reuse. |
Optimize Your Operations With AI Agents
Our AI agents streamline your workflows, unlocking new levels of business efficiency!
Customization and extensibility: Tailoring ZBrain to your enterprise needs
A major advantage of ZBrain’s modular architecture is that each component can be independently extended or swapped, allowing enterprises to customize the platform deeply. The interactions between modules are standardized, which means as long as a replacement adheres to the expected interface, it can plug into the system. Here are a few ways enterprises leverage this extensibility in practice:
-
Swapping vector databases: Organizations often have preferences for certain databases. ZBrain’s knowledge base is storage-agnostic so that teams can choose or change the vector store backend at will. For example, a company might start with ZBrain’s built-in vector store for cost reasons, then switch to Pinecone for scalable vector indexing as their data grows, without altering their application logic. The ability to integrate various vector databases (Pinecone, Qdrant etc.) means the knowledge layer can fit into whatever data infrastructure the enterprise is already comfortable with.
-
Integrating custom LLMs: If an enterprise has a proprietary LLM (say, a fine-tuned model specialized in their industry, or an open-source model running on-prem for data privacy), ZBrain can accommodate it. Thanks to the model-agnostic LLM layer, new model endpoints can be integrated. For instance, one could plug in a local instance of a LLaMA-2 model or a third-party AI service not originally bundled with ZBrain. The system’s broad support for leading AI models and open integration points enables this flexibility. This means companies are not locked into one AI vendor – they can bring their own AI models and still use ZBrain’s orchestration and workflow capabilities around them.
-
Extending agents with new tools: The library of pre-built flow components in ZBrain is extensive (spanning common apps and utilities), but it cannot possibly cover every custom internal tool a company might have. Fortunately, ZBrain allows developers to create custom steps or connectors as needed. If you have an in-house database or an API unique to your business, you can write a custom integration (e.g., a Python code step in the flow) to connect ZBrain to that system. The flow engine treats it as just another step. Enterprises often extend agents by adding these custom code blocks or by using the SDK to integrate niche services, thereby tailoring the automation to their exact processes.
-
Embedding AI in existing applications: Many enterprises choose to embed ZBrain’s capabilities into their existing user-facing applications. Because ZBrain exposes APIs and SDKs, a development team can, for example, insert an “AI Assistant” feature into an existing corporate intranet or mobile app. ZBrain handles the AI logic behind the scenes, while the enterprise app handles the UI. This extensibility means you don’t have to migrate users to a new platform – you can improve the software they already use by plugging in ZBrain on the back-end. One real-world scenario is integrating a ZBrain-powered agent into a customer support ticketing system, so that support reps see AI-suggested answers right inside their ticket interface. The integration layer’s design (with API endpoints and secure deployment options) makes such embedding straightforward.
-
Independent scaling of modules: Since each module is loosely coupled, they can be scaled independently. If your vector database needs a more powerful cluster to handle growing knowledge, you can scale that up. If your LLM calls are the bottleneck, you could instantiate more model servers or use a higher-tier model instance. This design supports extensibility by allowing you to scale capacity on a module-by-module basis rather than scaling the entire platform as a monolith. Moreover, deploying each agent separately is an option, enabling microservice-like scalability and independent updates.
Because of these capabilities, we can say the modular design of ZBrain can minimize the effort required to achieve the customization – each piece could be worked on without breaking the others. This composability is a core reason we can consider ZBrain for enterprise AI: it’s not a black-box solution, but rather a toolkit of building blocks that can be assembled and modified to fit one’s architecture and policies.
Best practices for ZBrain: Security, performance, and governance
Implementing ZBrain in an enterprise setting requires thoughtful planning of deployment and adherence to best practices to ensure security, compliance, and optimal performance. Below are some best practices and considerations for enterprises when rolling out ZBrain’s modular AI stack:
-
Choose the right deployment model: ZBrain supports flexible deployment options, including fully private deployments on your own cloud infrastructure. For sensitive environments, it’s recommended to deploy ZBrain in a single-tenant mode within your private cloud or data center. This ensures that all data (ingested documents, vector indexes, model interactions) remain under your control. Many enterprises deploy ZBrain on AWS, Azure, or GCP in their own account, leveraging Kubernetes or VMs, which allows integration with their network (VPC) and security controls. This way, the AI platform adheres to enterprise data residency requirements and can access internal data sources directly. If using a SaaS or multi-tenant version of ZBrain, ensure it offers appropriate data isolation.
-
Enforce governance and security controls: Take advantage of ZBrain’s built-in security features and governance. Define clear user roles and permissions in the platform so that only authorized team members can create or modify agents, access certain knowledge bases, or call certain models. Use the platform’s RBAC (role-based access control) to segregate duties (for example, limit who can deploy agents to production vs. who can only test in dev). All data ingested into ZBrain should be classified and handled according to its sensitivity – the knowledge base can store confidential data securely, but you should still apply data governance (e.g., do not ingest personally identifiable information unless needed, and if so, use appropriate retention policies).
-
Optimize the knowledge base for performance: The performance of AI responses often hinges on how fast and relevant the knowledge retrieval is. To optimize this:
-
Tune chunk size and indexing: Experiment with the chunk size (the granularity of pieces the documents are split into) to balance retrieval recall and precision. Smaller chunks can improve relevance but may require retrieving more of them. ZBrain allows configuring chunking rules and even the embedding model used for indexing – choose embeddings that work well for your data domain (for example, code vs. legal text).
-
Vector store selection: Use a vector database that meets your latency and scale needs. ZBrain’s storage-agnostic design means you can use high-performance vector DBs if low latency is crucial. For global deployments, consider a vector store that supports geo-distributed indexing to serve users in multiple regions quickly.
-
Hybrid search: Leverage the hybrid search (vector + keyword) capabilities for complex queries. This can often yield better performance for enterprise data by combining semantic understanding with exact term matching. It may reduce instances where the AI “misses” a relevant document due to phrasing.
-
-
Leverage evaluation and guardrails: Before deploying any AI agent to production, use ZBrain’s evaluation suite and guardrail features to validate its performance. Also, plan a human-in-the-loop review for critical tasks. For example, if an agent is drafting content for external use (like a press release or an email to a client), you might require a human approval step in the flow. ZBrain makes it easy to incorporate such a manual checkpoint. These practices ensure that the AI outputs remain reliable and compliant, increasing trust from stakeholders.
-
Monitor, iterate, and scale: Once AI solutions are deployed, continuous monitoring is key. Use ZBrain’s AppOps to monitor agent performance. It continuously validates in the background to proactively identify and address issues, ensuring stability and preventing disruptions. It also offers monitoring capabilities for tracking AI application performance and health, providing real-time insights for timely interventions and optimizations.
By following these best practices, enterprises can deploy ZBrain’s AI capabilities in a way that is robust, secure, and efficient. The key is to treat each module with the same rigor as any enterprise system: secure your data ingestion and storage, QA your AI logic thoroughly, and monitor the system in production. ZBrain provides the tools to do this (from private deployments to guardrails and monitoring hooks), but the enterprise must apply its own standards and processes around those tools to truly achieve a reliable AI-driven solution.
Endnote
ZBrain Builder’s modular architecture – comprising data ingestion pipelines, a flexible knowledge base, an LLM-agnostic orchestration layer, powerful automation agents, and integration-ready interfaces – serves as a set of building blocks for enterprise AI solutions. Its composability allows organizations to craft custom AI applications aligned with their specific data, workflows, and compliance needs. Instead of a one-size-fits-all AI, ZBrain offers a “build-your-own-AI” platform, where each layer can be tuned or swapped to best suit the business.
For enterprises, this means AI initiatives can progress faster and with lower risk: teams can reuse the robust components of ZBrain and focus their effort on customizations that differentiate their solution (such as proprietary data and business logic). As AI technology evolves, ZBrain’s model-agnostic approach also safeguards these solutions from obsolescence, allowing new models or tools to be incorporated with minimal disruption.
In summary, ZBrain’s modular stack provides the “building blocks of AI” for the enterprise: each block (ingestion, knowledge, LLM, agents, UI) is powerful on its own, but together they form an end-to-end platform for generative AI and automation. By understanding and leveraging these components, organizations can accelerate their AI adoption and create custom solutions that drive significant business value – from improved decision-making and efficiency to enhanced customer experiences – all on a foundation that is flexible, extensible, and enterprise-ready.
Ready to transform your enterprise AI strategy? Contact us today and discover how ZBrain’s modular stack can be tailored to your unique business needs.
Listen to the article
Author’s Bio
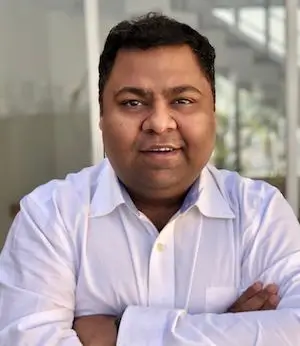
An early adopter of emerging technologies, Akash leads innovation in AI, driving transformative solutions that enhance business operations. With his entrepreneurial spirit, technical acumen and passion for AI, Akash continues to explore new horizons, empowering businesses with solutions that enable seamless automation, intelligent decision-making, and next-generation digital experiences.
Table of content
- Navigating the challenges enterprises face in AI adoption
- What are the strategic benefits of modular architecture for enterprise AI?
- Introducing ZBrain: A comprehensive enterprise AI platform
- How does ZBrain Builder's modular architecture enable the building of flexible, scalable, and vendor-agnostic AI solutions?
- Customization and extensibility: Tailoring ZBrain to your enterprise needs
- Best practices for ZBrain: Security, performance, and governance
What is ZBrain’s modular stack, and how does it support custom AI solutions?
ZBrain’s modular stack is composed of independent components designed to work together, enabling the creation of tailored AI solutions aligned with specific workflows and business needs. These modules include:
-
Data integration and ingestion: Connects to multiple data sources, ingests data (even in real time), and preprocesses it for AI use.
-
Knowledge base: Stores and indexes data for efficient retrieval, serving as the core repository for AI models.
-
LLM orchestration layer: Manages the interaction with different AI models, routing queries to the most appropriate model.
-
Orchestration engine and agents: Defines workflows and automates multi-step tasks across systems and AI models.
-
User interface and integration layer: Provides APIs, SDKs, and UI components for seamless interaction with AI solutions.
This modular design allows enterprises to mix and match components, enabling highly customizable, scalable, and flexible AI solutions that integrate smoothly into existing systems.
How flexible is ZBrain’s modular stack when it comes to building tailor-made AI solutions for various industries?
ZBrain’s modular architecture is highly customizable, enabling enterprises to build AI solutions suited to their unique industry requirements. Components like the knowledge base and LLM orchestration can be swapped or extended to fit specific business processes, ensuring that AI solutions are flexible and adaptable to different sectors such as healthcare, finance, and manufacturing.
What are the benefits of using ZBrain’s modular stack for integration?
The modular stack in ZBrain provides integration benefits by allowing enterprises to configure agents with components that align with their infrastructure and business logic. These components include selectable foundation models, vector memory stores, customizable system prompts and more. This modularity ensures that enterprises can tailor each agent to specific tasks or departments, accelerating development while maintaining flexibility and control.
Other benefits include faster deployment, reduced engineering overhead, and the ability to scale or adjust AI capabilities based on evolving requirements—all without restructuring existing systems.
Can ZBrain’s modular stack scale with growing data and complexity in AI projects? How does it handle high-demand applications?
Yes, ZBrain is designed for scalability. The modular design supports horizontal scaling, enabling individual components like data ingestion or LLMs to scale independently based on demand.
How does ZBrain ensure optimal performance of AI models used within its platform?
How do we get started with ZBrain for AI development?
To begin your AI journey with ZBrain:
- Contact us at hello@zbrain.ai
- Or fill out the inquiry form on zbrain.ai
Our dedicated team will work with you to evaluate your current AI development environment, identify key opportunities for AI integration, and design a customized pilot plan tailored to your organization’s goals.
Insights
How ZBrain accelerates AI development and deployment
ZBrain addresses the comprehensive AI development lifecycle with an integrated platform composed of distinct yet interconnected modules that ensure enterprises accelerate AI initiatives while maintaining strategic alignment, technical feasibility, and demonstrable value.
How to build AI agents with ZBrain?
By leveraging ZBrain’s blend of simplicity, customization, and advanced AI capabilities, organizations can develop and deploy AI agents that are both powerful and tailored to meet unique business demands, enhancing productivity and operational intelligence across the board.
How to build a search-optimized knowledge repository with ZBrain
ZBrain’s advanced knowledge base is engineered to scale with your enterprise needs, whether you’re handling a few thousand records or millions of documents, without compromising performance or uptime.
How ZBrain enhances knowledge retrieval with intelligent reranking
ZBrain significantly enhances the relevance of enterprise search results through reranking, acting as an intelligent gatekeeper between user queries and corporate data.
Monitoring ZBrain AI agents: Exploring key metrics
AI agent monitoring is the practice of systematically tracking and analyzing an agent’s behavior, outputs, and impact to ensure it operates reliably, fairly, and efficiently.
How ZBrain’s multi-agent collaboration works
ZBrain’s multi-agent collaboration is an advanced approach in which multiple specialized AI agents work together as a cohesive team to solve complex enterprise challenges.
AI in project and capital expenditure management
Adopting AI across the capital expenditure (CapEx) lifecycle can radically improve how organizations plan, execute, and evaluate big investments.
AI for plan-to-results
The integration of AI into plan-to-results operations is transforming how organizations align strategic planning with execution to optimize business performance.
AI for accounts payable and receivable
The integration of AI in AP and AR operations extends beyond routine automation, offering strategic insights that empower finance professionals to evolve from reactive problem-solvers into proactive strategists.